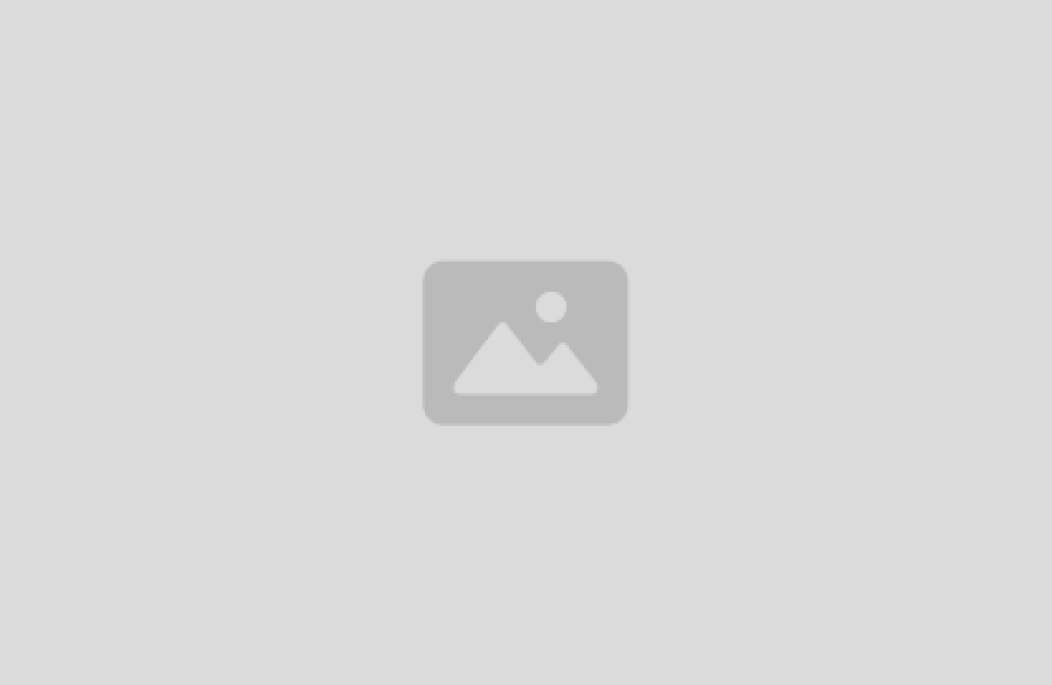
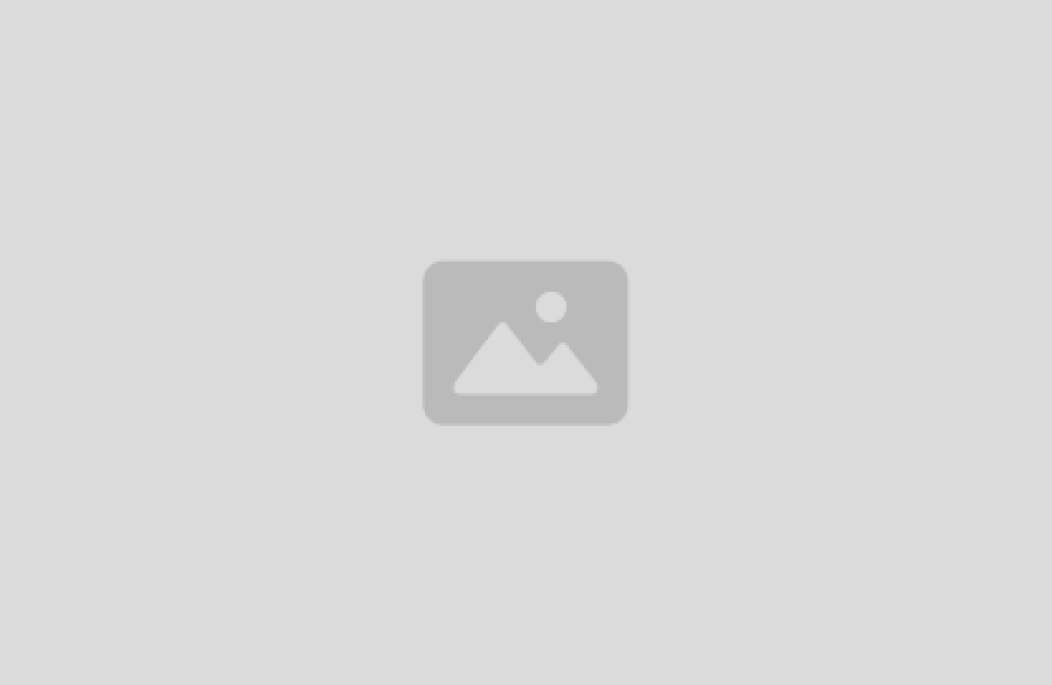
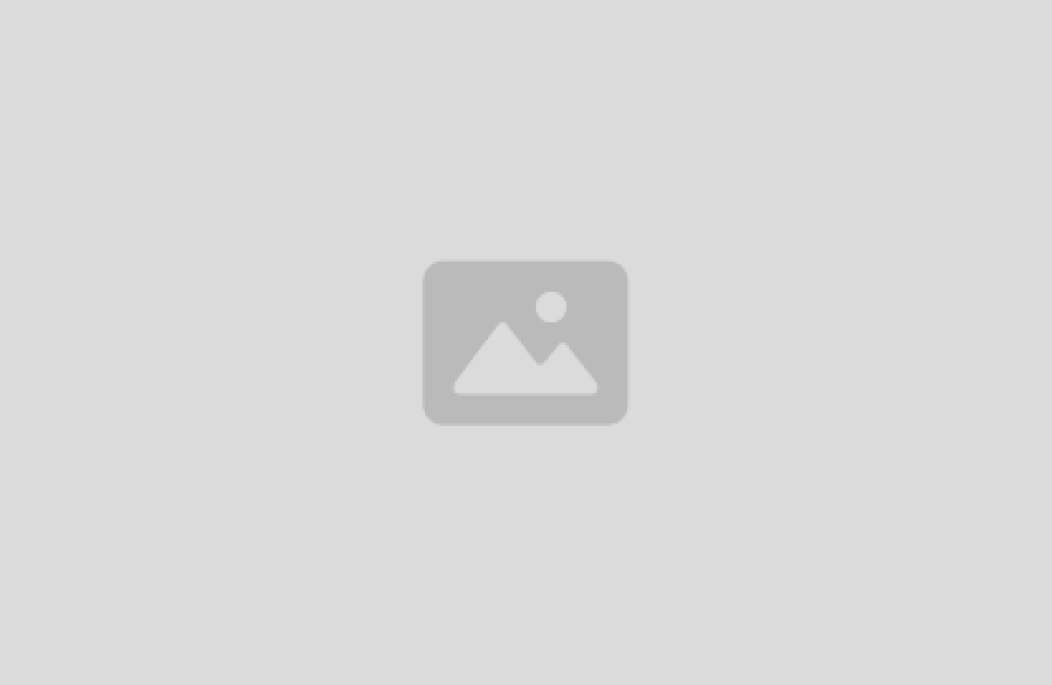
Discover more integrations
No items found.
Get in touch CTA Section
Lorem ipsum dolor sit amet, consectetur adipiscing elit, sed do eiusmod tempor incididunt ut labore et dolore magna aliqua.
Frequently asked questions
Why is this integration important for data pipeline monitoring?
Bringing Sifflet’s observability tools into Apache Airflow allows for proactive data pipeline monitoring. You get real-time metrics, anomaly detection, and data freshness checks that help you catch issues early and keep your pipelines healthy.
Can the Sifflet AI Assistant help non-technical users with data quality monitoring?
Absolutely! One of our goals is to democratize data observability. The Sifflet AI Assistant is designed to be accessible to both technical and non-technical users, offering natural language interfaces and actionable insights that simplify data quality monitoring across the organization.
What kind of monitoring should I set up after migrating to the cloud?
After migration, continuous data quality monitoring is a must. Set up real-time alerts for data freshness checks, schema changes, and ingestion latency. These observability tools help you catch issues early and keep your data pipelines running smoothly.
What are the main differences between ETL and ELT for data integration?
ETL (Extract, Transform, Load) transforms data before storing it, while ELT (Extract, Load, Transform) loads raw data first, then transforms it. With modern cloud storage, ELT is often preferred for its flexibility and scalability. Whichever method you choose, pairing it with strong data pipeline monitoring ensures smooth operations.
What is data observability and why is it important for modern data teams?
Data observability is the ability to monitor and understand the health of your data across the entire data stack. As data pipelines become more complex, having real-time visibility into where and why data issues occur helps teams maintain data reliability and trust. At Sifflet, we believe data observability is essential for proactive data quality monitoring and faster root cause analysis.
How do logs contribute to observability in data pipelines?
Logs capture interactions between data and external systems or users, offering valuable insights into data transformations and access patterns. They are essential for detecting anomalies, understanding data drift, and improving incident response in both batch and streaming data monitoring environments.
How does SQL Table Tracer handle complex SQL features like CTEs and subqueries?
SQL Table Tracer uses a Monoid-based design to handle complex SQL structures like Common Table Expressions (CTEs) and subqueries. This approach allows it to incrementally and safely compose lineage information, ensuring accurate root cause analysis and data drift detection.
How does Sifflet help detect and prevent data drift in AI models?
Sifflet is designed to monitor subtle changes in data distributions, which is key for data drift detection. This helps teams catch shifts in data that could negatively impact AI model performance. By continuously analyzing incoming data and comparing it to historical patterns, Sifflet ensures your models stay aligned with the most relevant and reliable inputs.