Analytics Trust and Reliability
Shared Understanding. Ultimate Confidence. At Scale.
When everyone knows your data is systematically validated for quality, understands where it comes from and how it's transformed, and is aligned on freshness and SLAs, what’s not to trust?
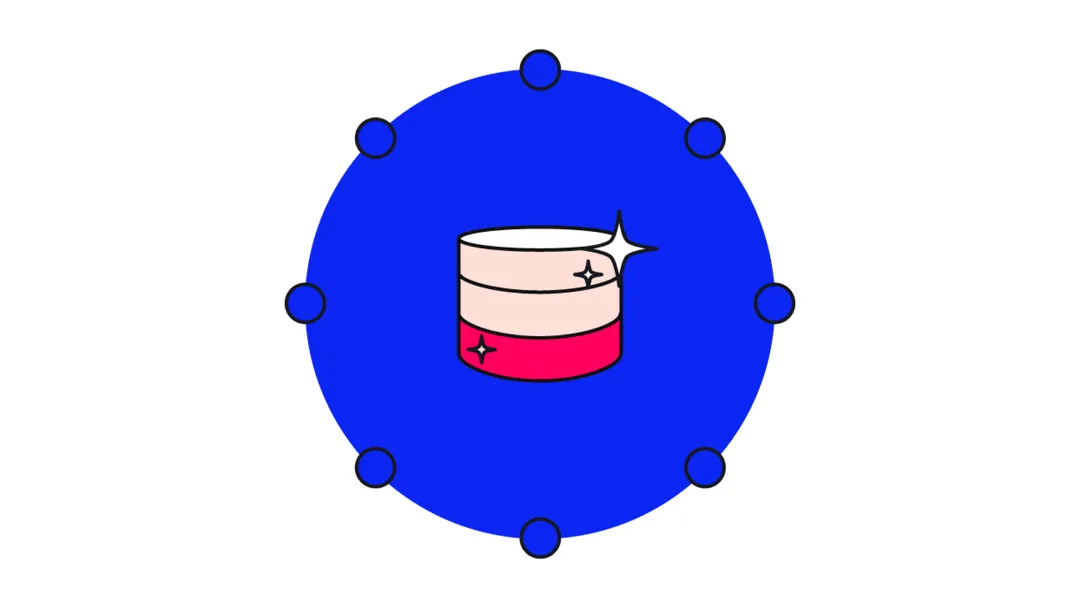
Always Fresh. Always Validated.
No more explaining data discrepancies to the C-suite. Thanks to automatic and systematic validation, Sifflet ensures your data is always fresh and meets your quality requirements. Stakeholders know when data might be stale or interrupted, so they can make decisions with timely, accurate data.
- Automatically detect schema changes, null values, duplicates, or unexpected patterns that could comprise analysis.
- Set and monitor service-level agreements (SLAs) for critical data assets.
- Track when data was last updated and whether it meets freshness requirements
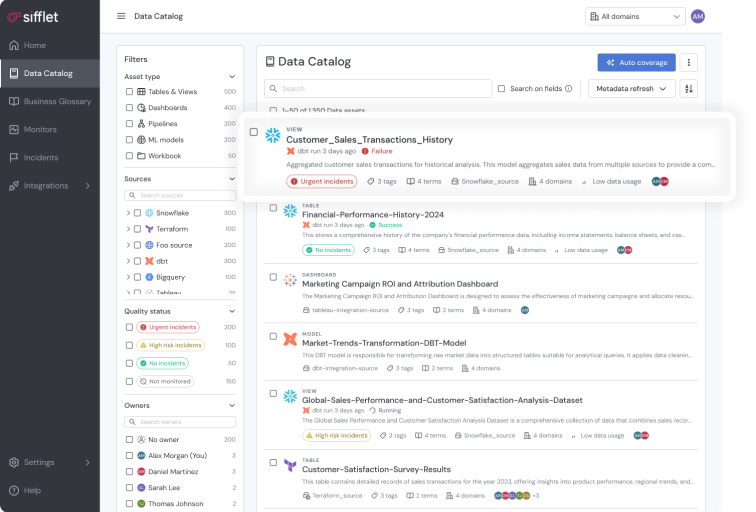
Understand Your Data, Inside and Out
Give data analysts and business users ultimate clarity. Sifflet helps teams understand their data across its whole lifecycle, and gives full context like business definitions, known limitations, and update frequencies, so everyone works from the same assumptions.
- Create transparency by helping users understand data pipelines, so they always know where data comes from and how it’s transformed.
- Develop shared understanding in data that prevents misinterpretation and builds confidence in analytics outputs.
- Quickly assess which downstream reports and dashboards are affected
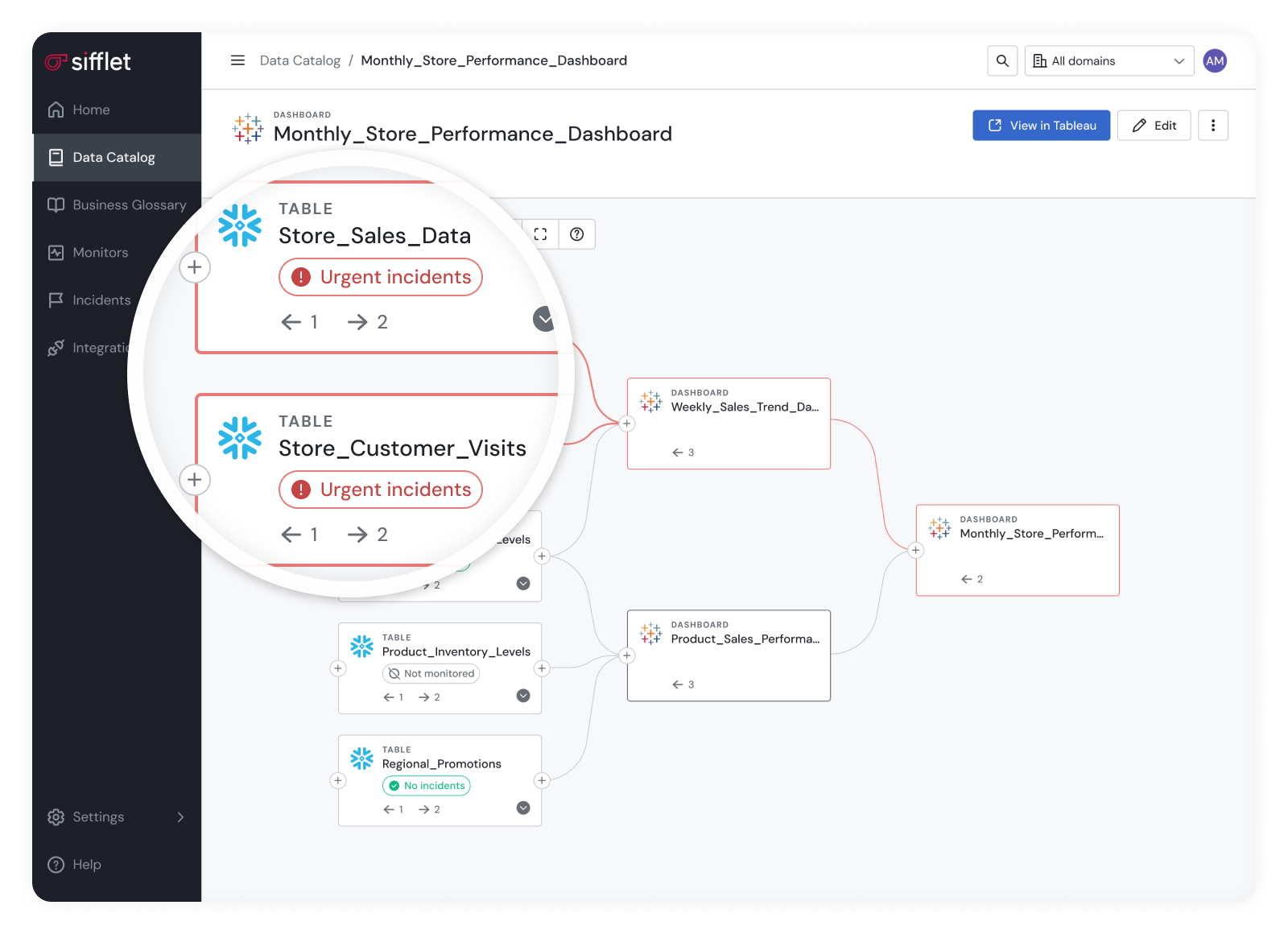

Frequently asked questions
What kind of insights can I gain by integrating Airbyte with Sifflet?
By integrating Airbyte with Sifflet, you unlock real-time insights into your data pipelines, including data freshness checks, anomaly detection, and complete data lineage tracking. This helps improve SLA compliance, reduces troubleshooting time, and boosts your confidence in data quality and pipeline health.
What are some best practices Hypebeast followed for successful data observability implementation?
Hypebeast focused on phased deployment of observability tools, continuous training for all data users, and a strong emphasis on data quality monitoring. These strategies helped ensure smooth adoption and long-term success with their observability platform.
What are the main differences between ETL and ELT for data integration?
ETL (Extract, Transform, Load) transforms data before storing it, while ELT (Extract, Load, Transform) loads raw data first, then transforms it. With modern cloud storage, ELT is often preferred for its flexibility and scalability. Whichever method you choose, pairing it with strong data pipeline monitoring ensures smooth operations.
What role does reverse ETL play in operational analytics?
Reverse ETL bridges the gap between data teams and business users by moving data from the warehouse into tools like CRMs and marketing platforms. This enables operational analytics, where business teams can act on real-time data. To ensure this process runs smoothly, data observability dashboards can monitor for pipeline errors and enforce data validation rules.
Why is stakeholder trust in data so important, and how can we protect it?
Stakeholder trust is crucial because inconsistent or unreliable data can lead to poor decisions and reduced adoption of data-driven practices. You can protect this trust with strong data quality monitoring, real-time metrics, and consistent reporting. Data observability tools help by alerting teams to issues before they impact dashboards or reports, ensuring transparency and reliability.
Why is data observability becoming essential for data-driven companies?
As more businesses rely on data to drive decisions, ensuring data reliability is critical. Data observability provides transparency into the health of your data assets and pipelines, helping teams catch issues early, stay compliant with SLAs, and ultimately build trust in their data.
How can integration and connectivity improve data pipeline monitoring?
When a data catalog integrates seamlessly with your databases, cloud storage, and data lakes, it enhances your ability to monitor data pipelines in real time. This connectivity supports better ingestion latency tracking and helps maintain a reliable observability platform.
What if I use tools that aren’t natively supported by Sifflet?
No worries at all! With Sifflet’s Universal Connector API, you can integrate data from virtually any source. This flexibility means you can monitor your entire data ecosystem and maintain full visibility into your data pipeline monitoring, no matter what tools you're using.