Sifflet is better for business
The best data leaders know that data quality isn’t just a technical issue. They want tooling that allows technical and business users to engage with data observability. That’s why teams are switching from Monte Carlo to Sifflet.
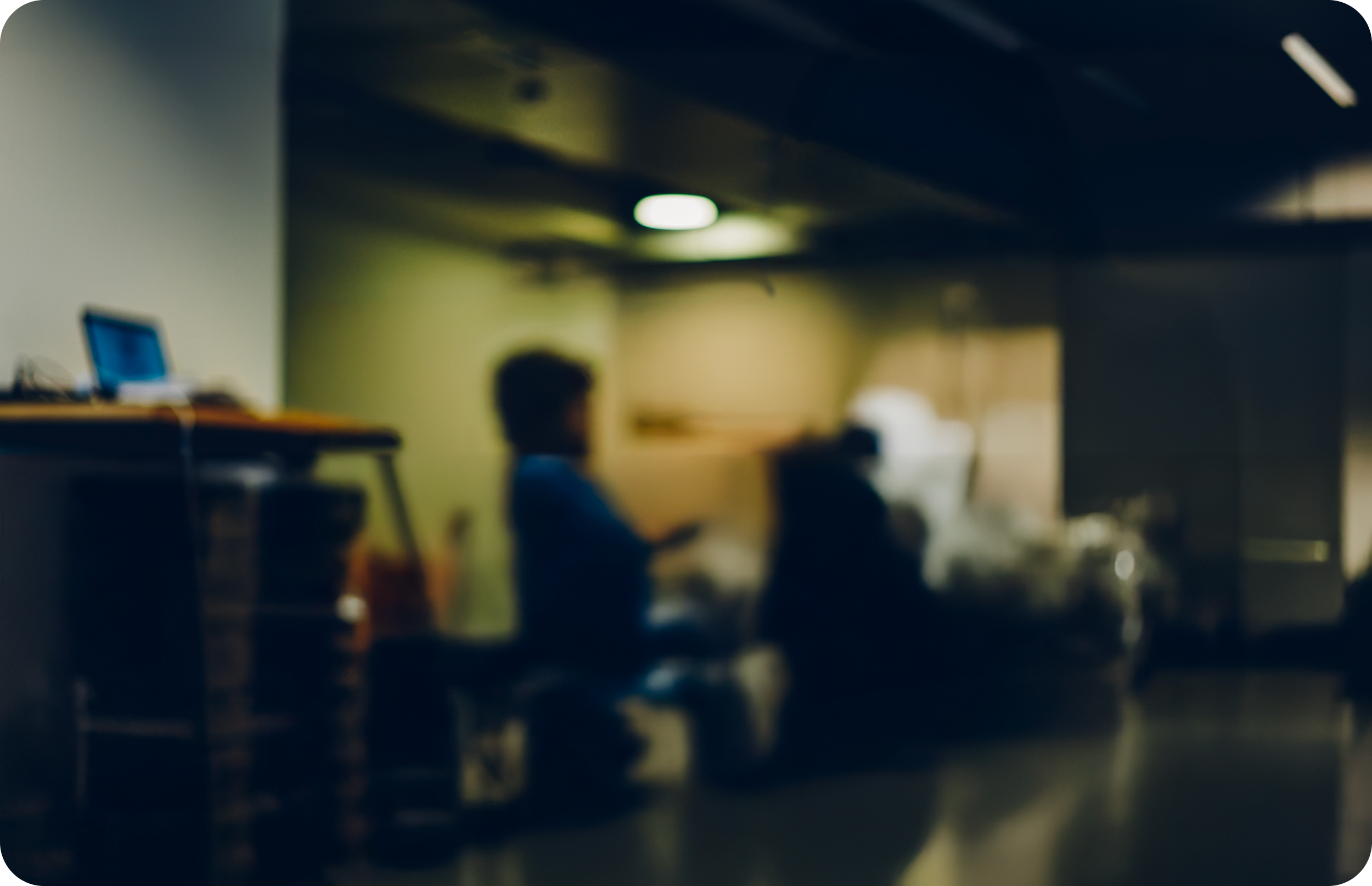
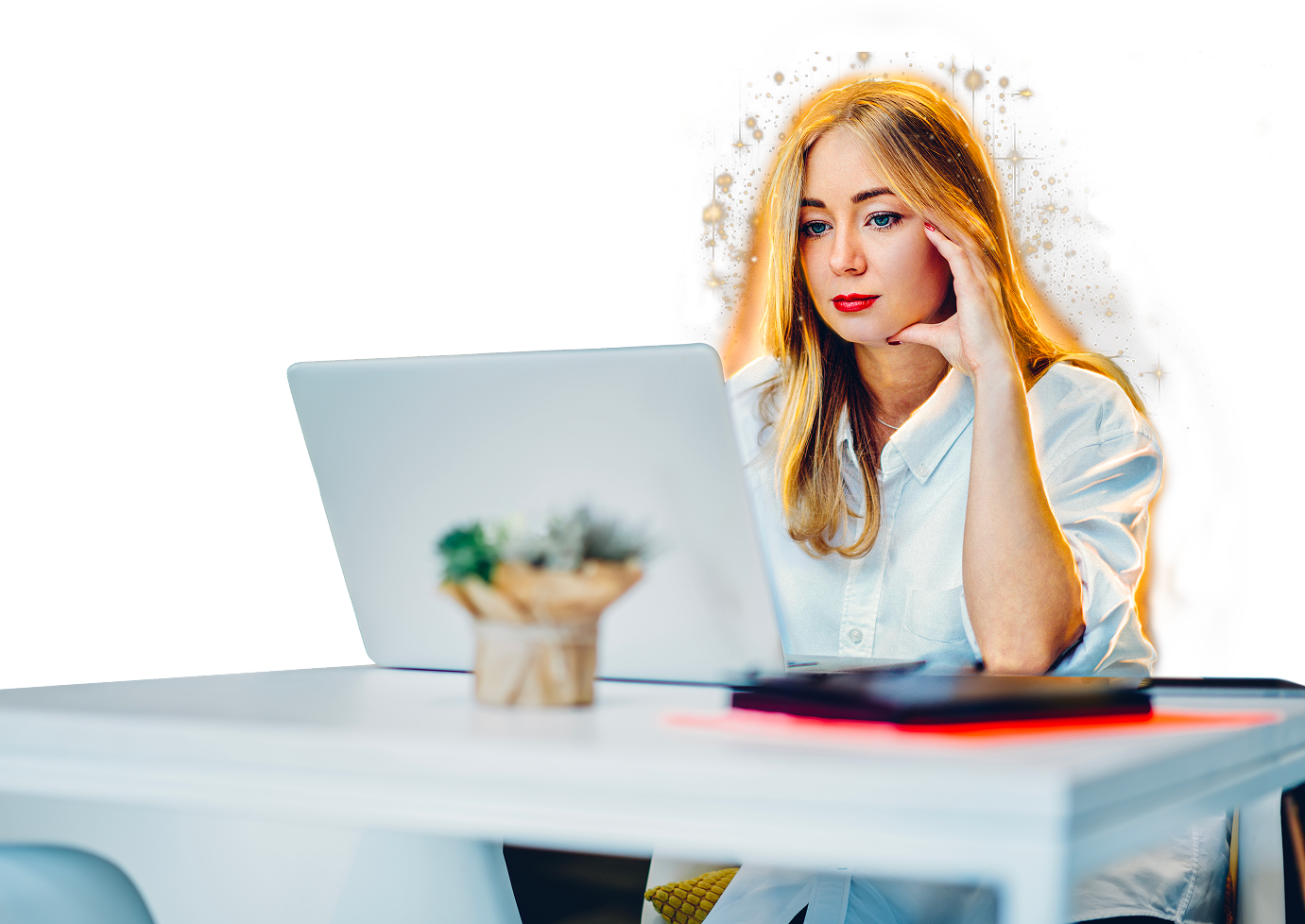

Augmented data quality for analytics and AI
Monte Carlo focuses on technical data quality, looking at freshness, accuracy, nulls, voids and more. Sifflet does this too, but it goes one step further—pulling in documentation and business context with input from downstream users to understand business critical data products and processes.
The result is truly augmented data quality for analytics and AI, because technical teams know exactly what to prioritize when it comes to monitoring and triaging.
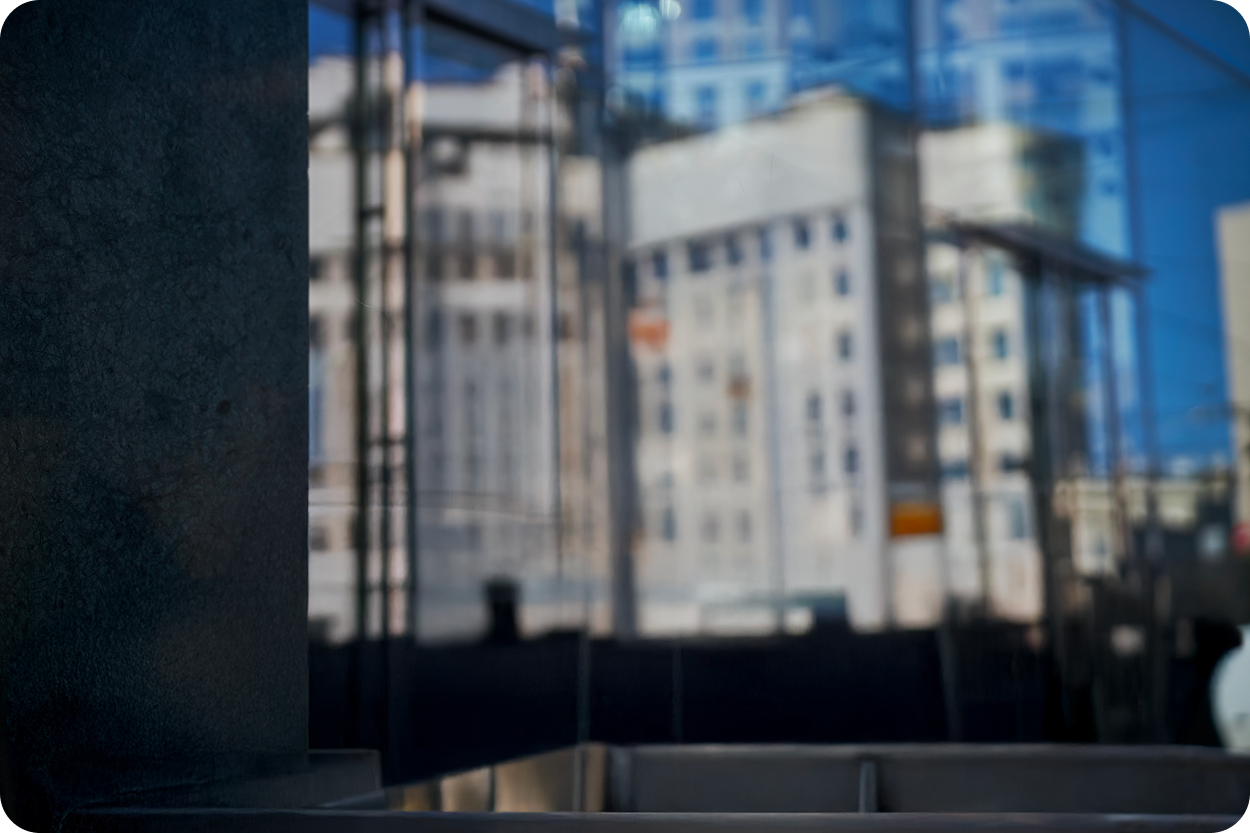
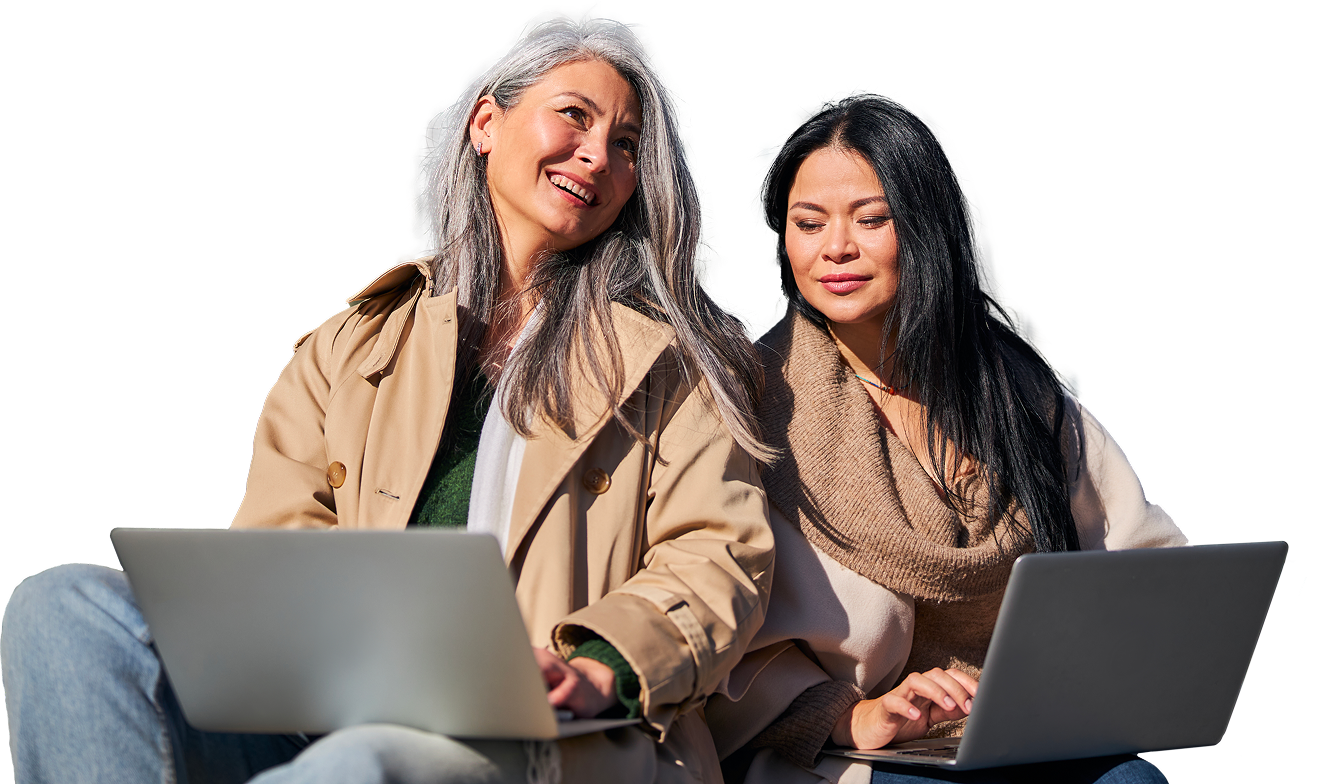

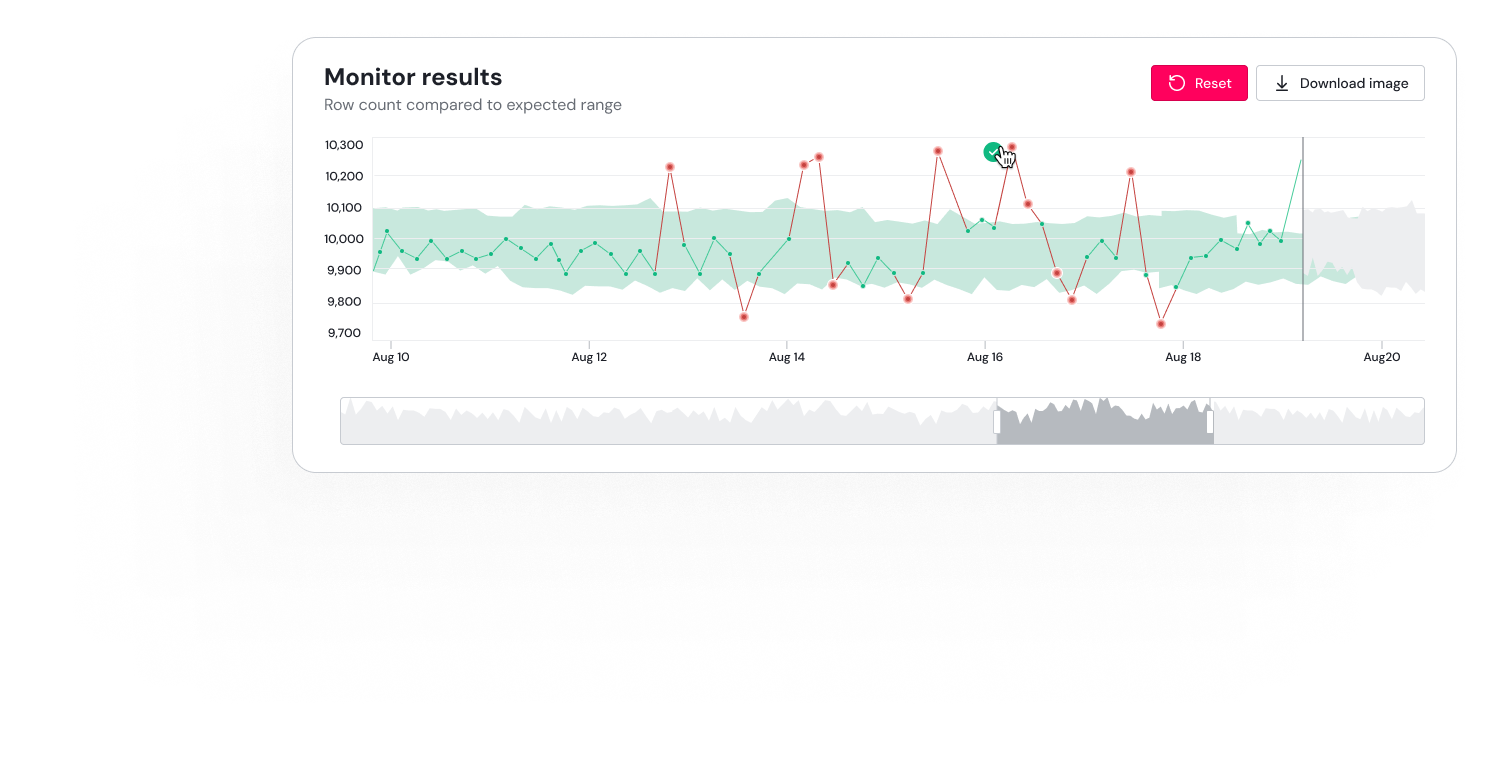
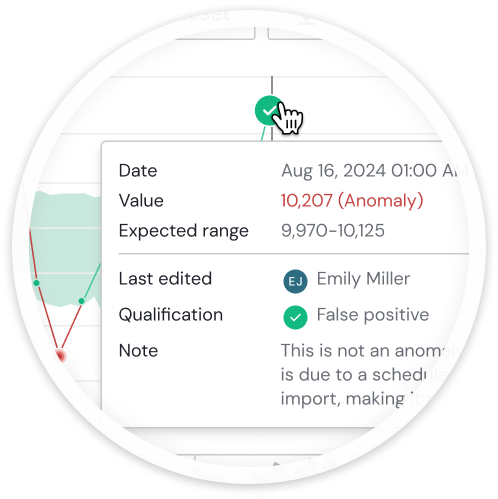
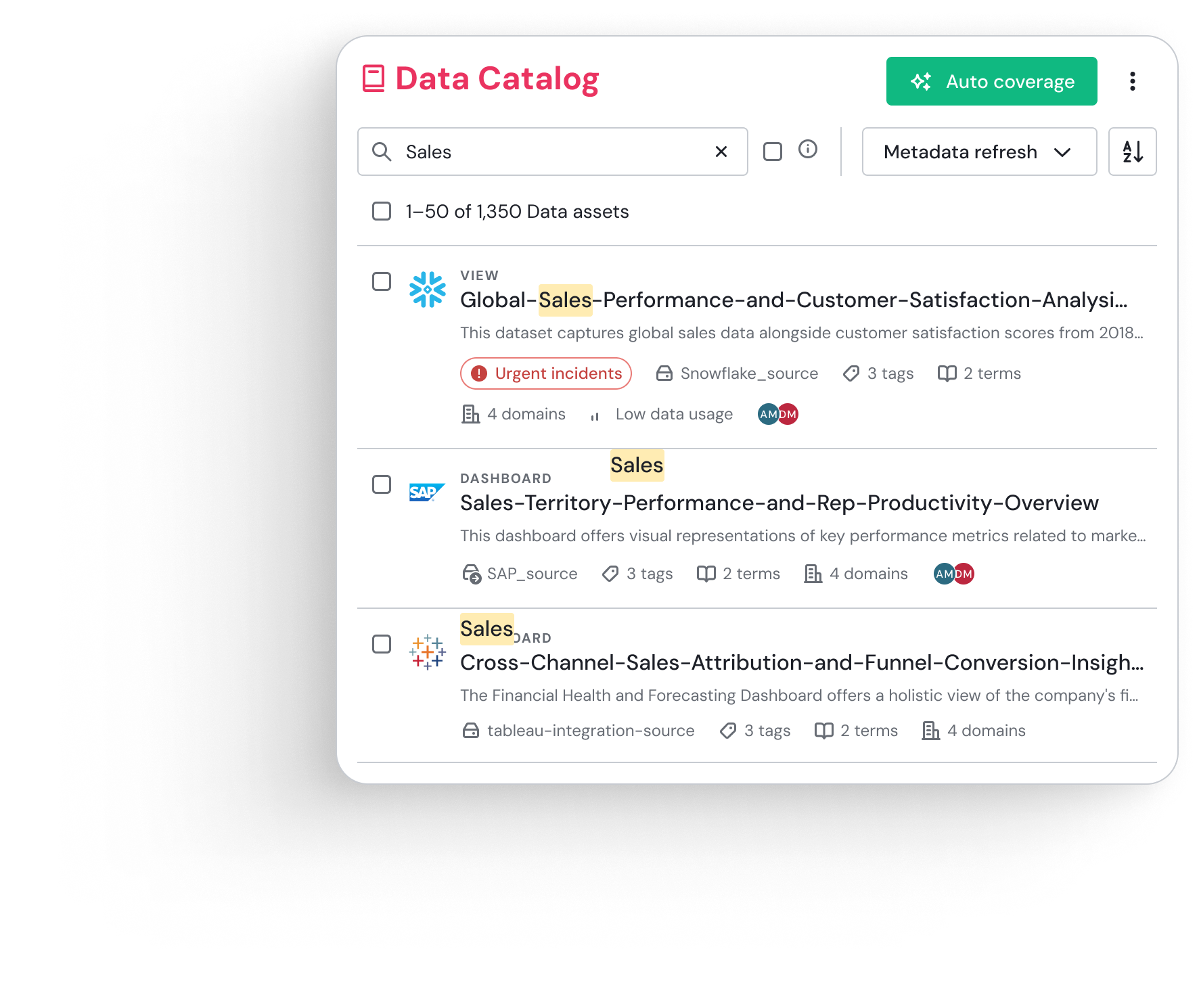
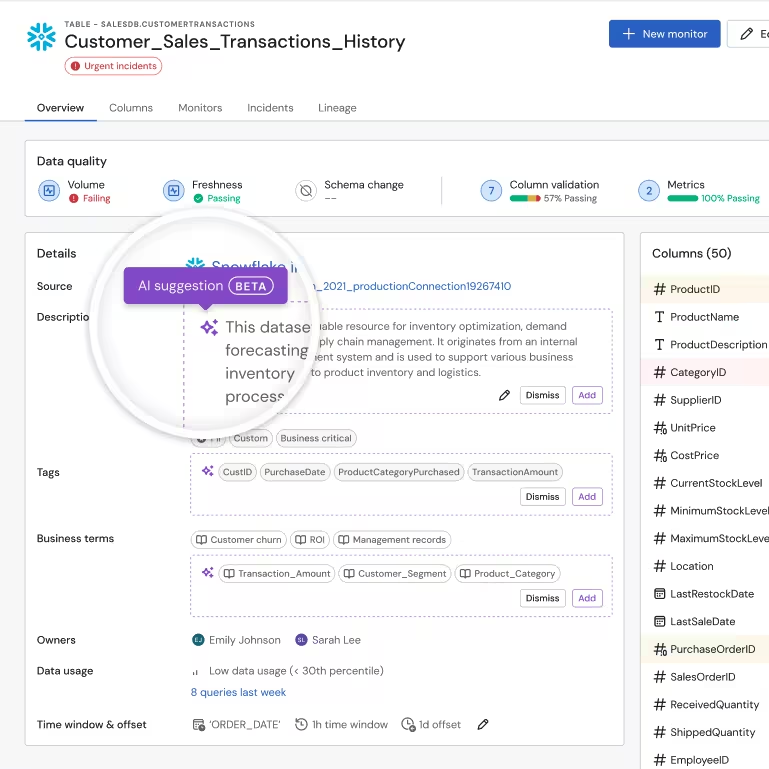

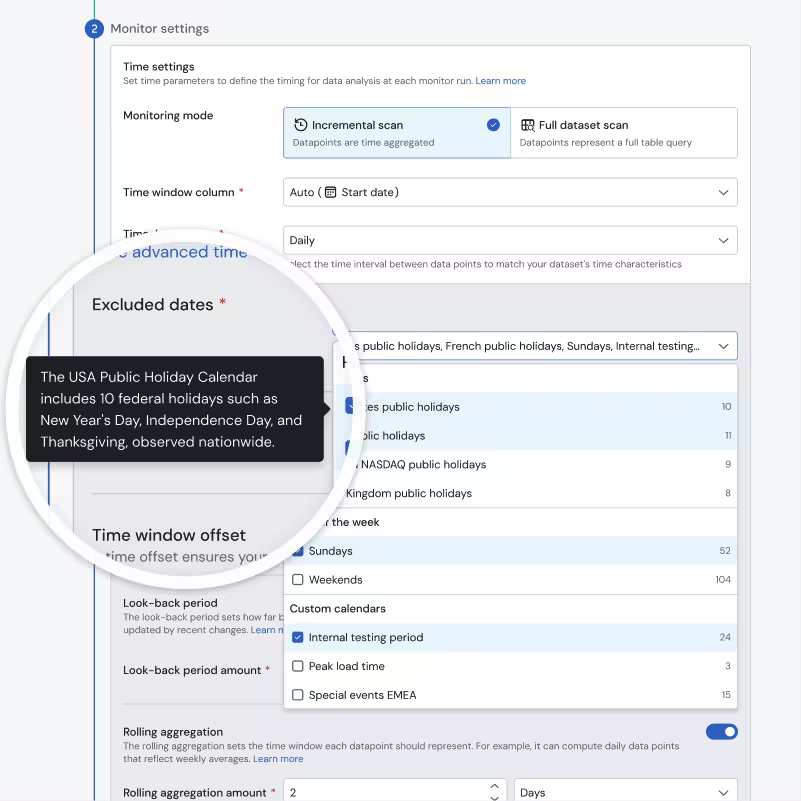
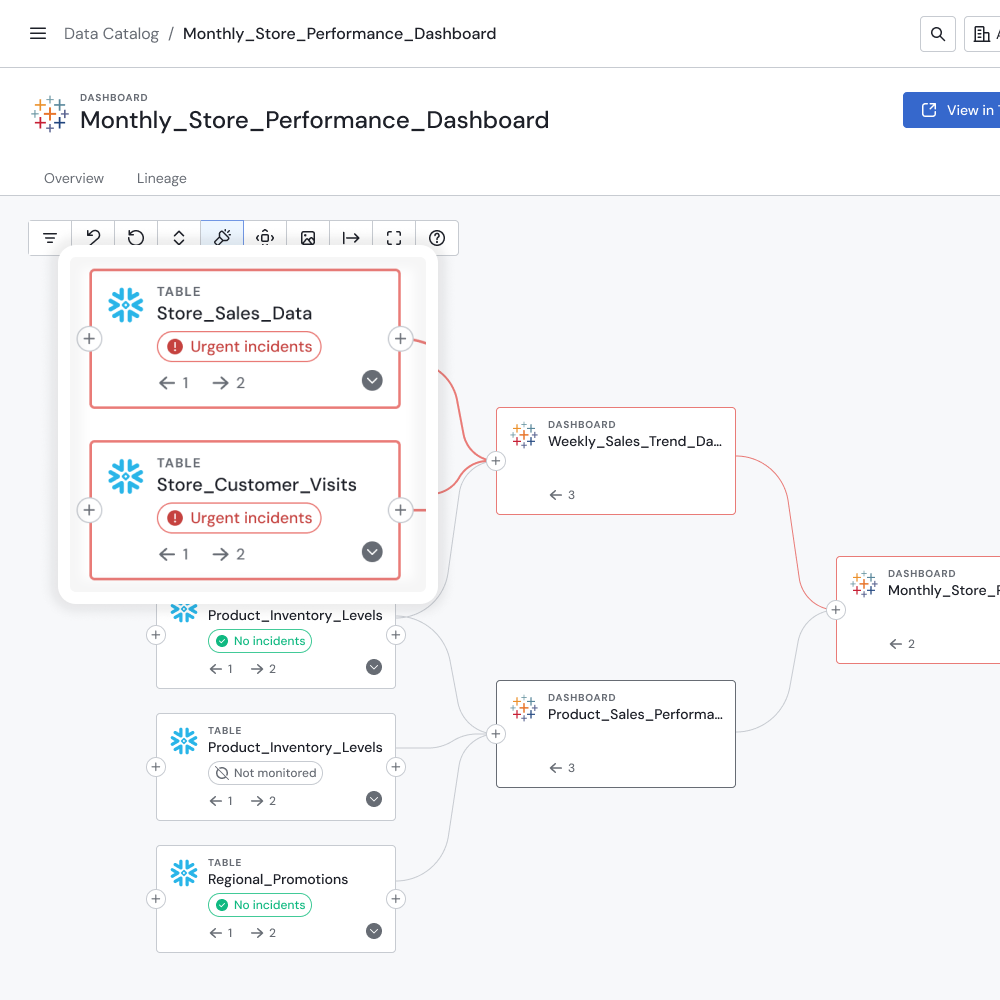
Don't Solve Half the Problem.
If you want to tackle data quality just from a technical perspective, Sifflet isn’t for you. But if you want to reach augmented data quality for analytics and AI that truly brings business value to downstream users, Sifflet is the right choice for today… and tomorrow.
There's no one size fits all.
When it comes to data observability platforms, there's no one size fits all.
Chat with one of our experts today to learn more about Sifflet and if it's the right option for you.
