Lean. Mean. Monitoring Machine.
Finally, dynamic monitoring that can keep up with your stack. AI features optimize your coverage and minimize noise, detecting issues before they arise.
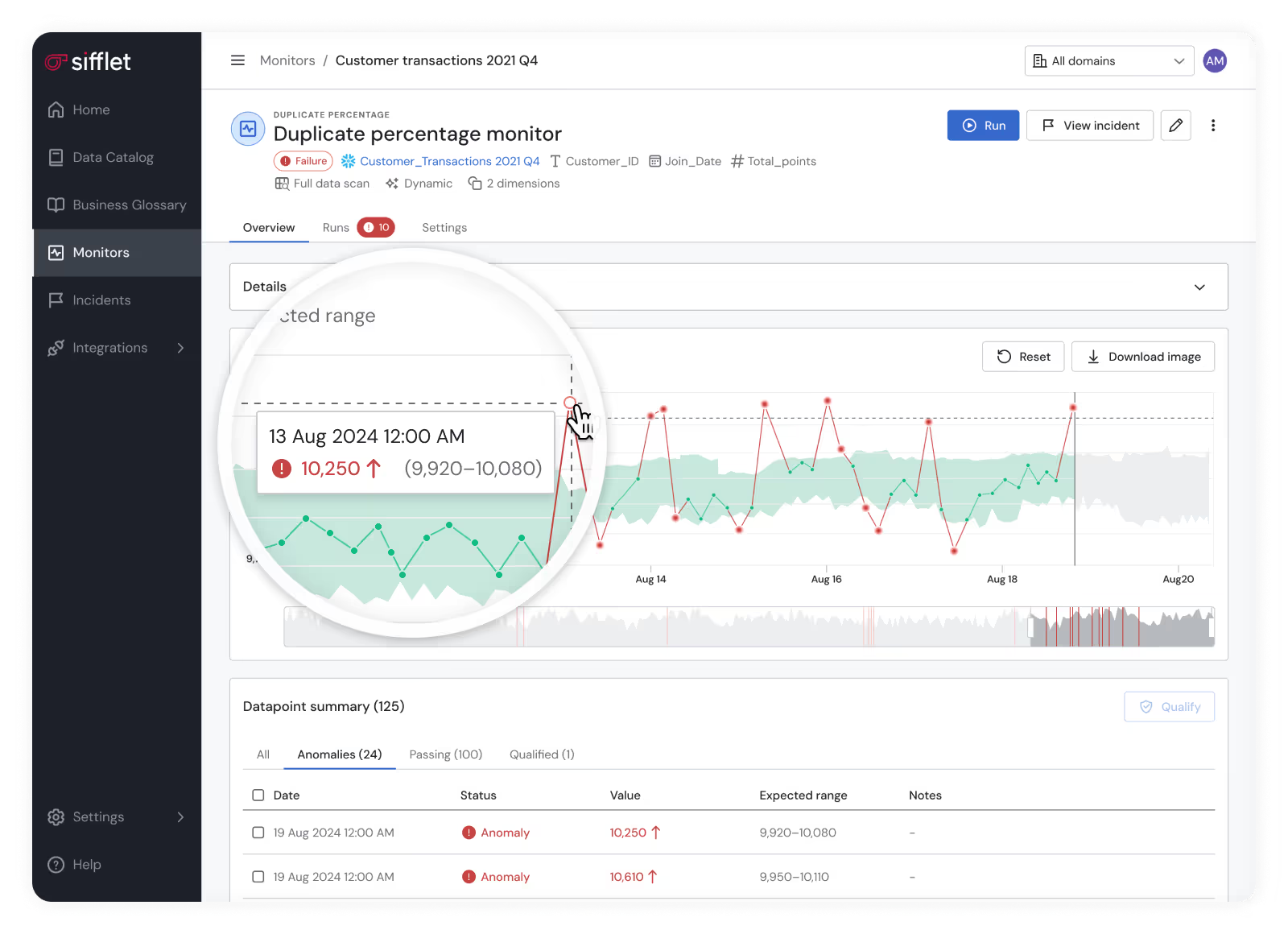

Customize to Your Heart’s Content
Sifflet offers both a robust library of out of the box monitors and customization capability. Your teams decide what needs monitoring and how to set it up.
Bye-Bye, Alert Fatigue
Data engineers don’t need more alerts, they need smarter alerts. Our AI learns adaptively as it goes to optimize coverage and minimize noise.
Hello, Data Reliability
Data reliability is reinforced with less manual work for technical teams, faster response times, and overall stronger performance.
Ready-to-Go Monitors
Quick set up and implementation means quicker results.
- See value instantly with pre-defined templates to check data at field and table levels
- Help your business users and technical teams meet their quality and reliability objectives thanks to ready-to-go monitors
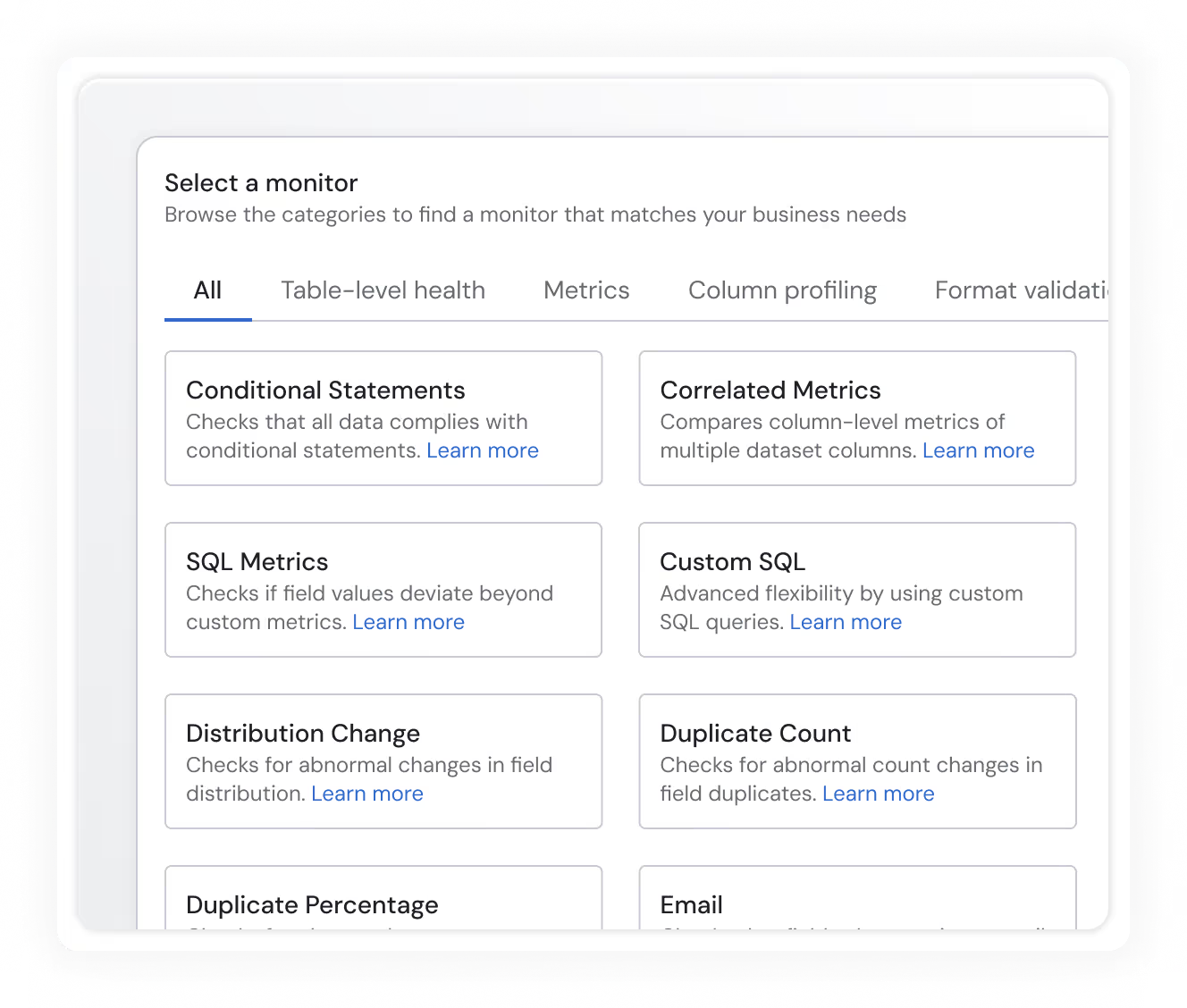
Lifecycle Monitoring
End-to-end coverage that never sleeps.
- Detect anomalies continuously thanks to ML models
- Give your business users ownership over monitors through LLM monitoring setup
- Maintain control and accuracy with optional manual setup and user feedback
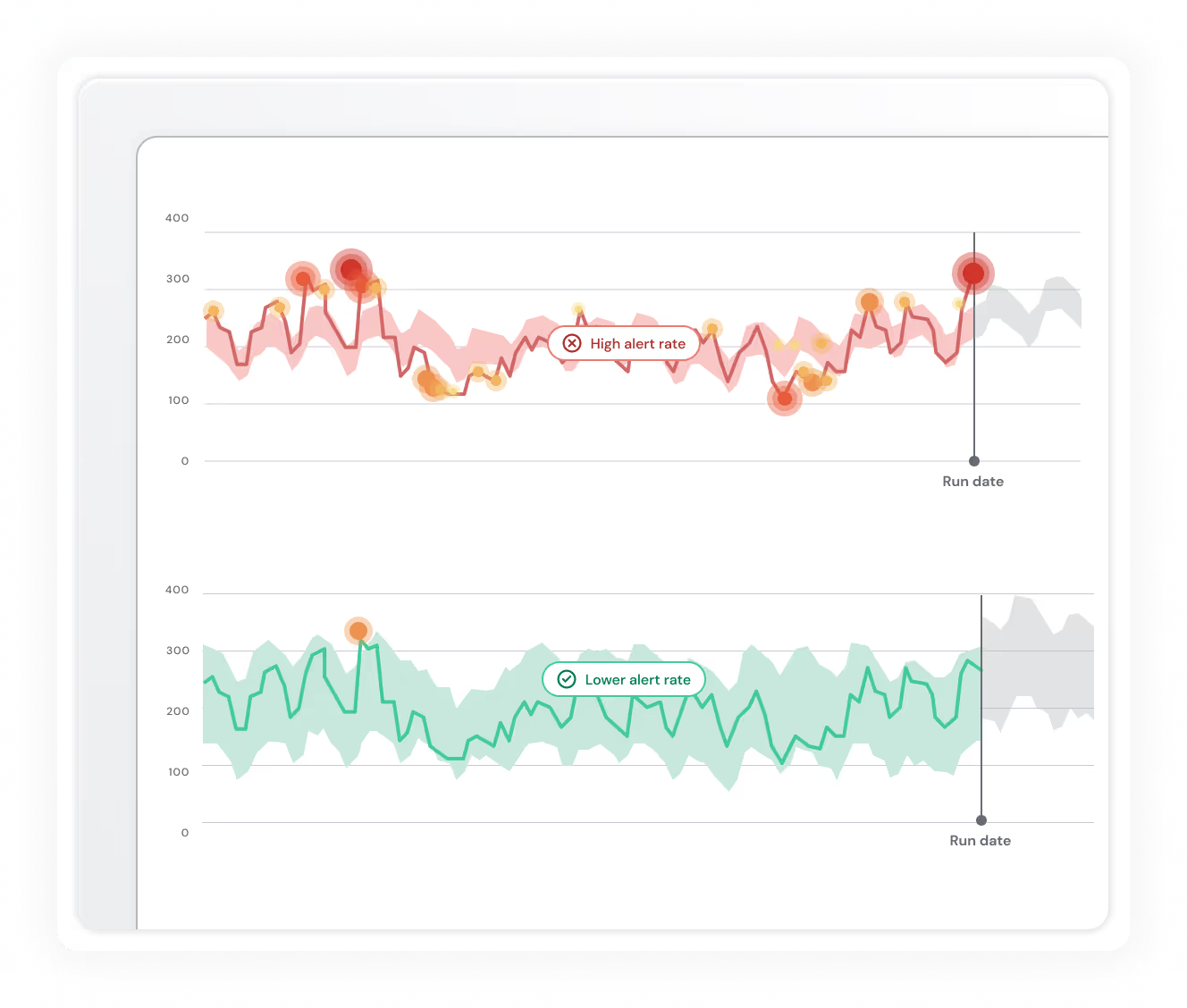
Scalability & Optimization
Monitoring that’s easy to maintain and coverage that’s just right.
- Optimize monitoring coverage and minimize noise levels with AI-powered suggestions and supervision
- Implement programmatic monitoring set up and maintenance with Data Quality as Code (DQaC)
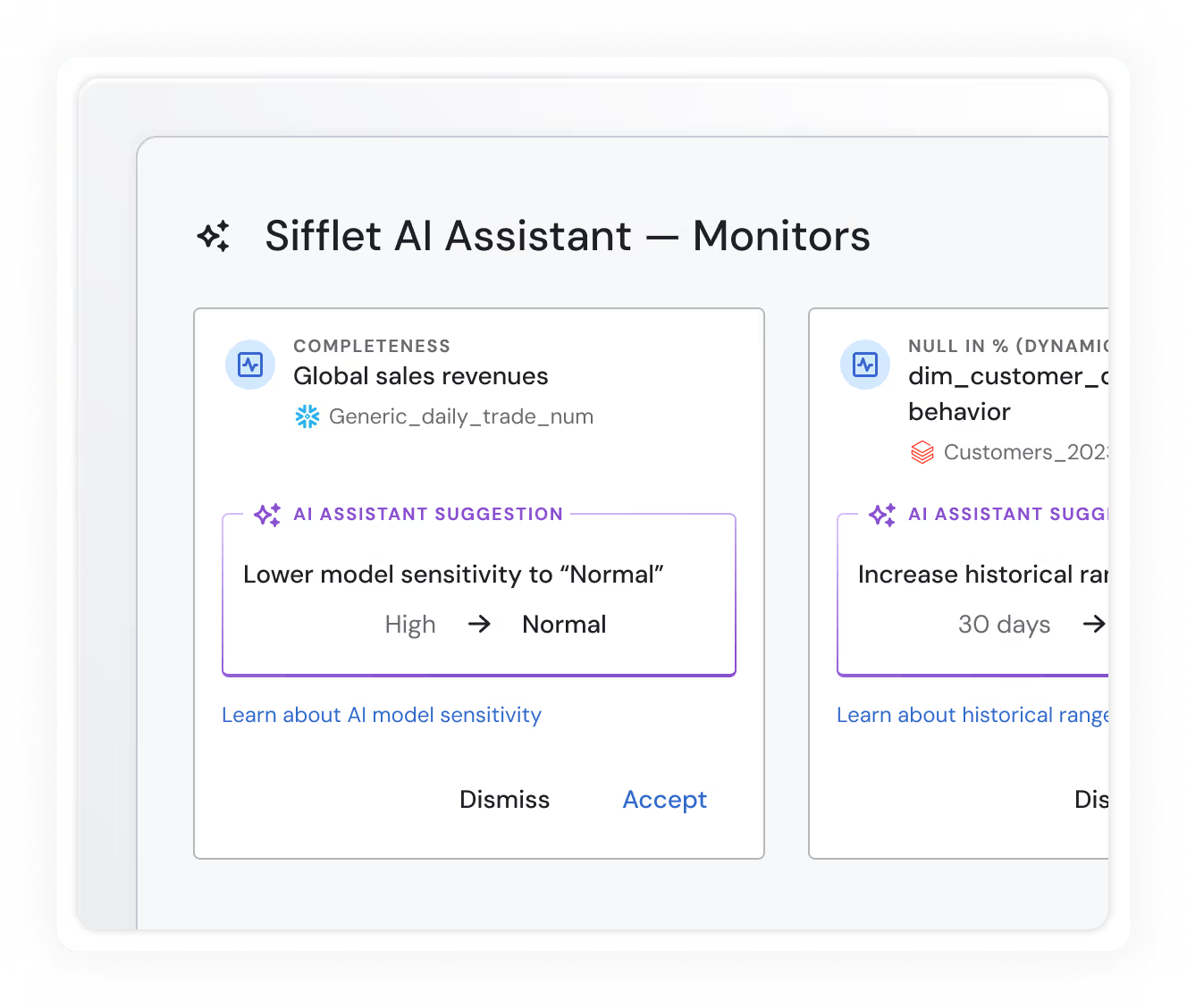
Reinforced Reliability
Built for Everyone
Sifflet’s monitoring features reinforce data reliability for all users, so business can deliver.
Looking for more?
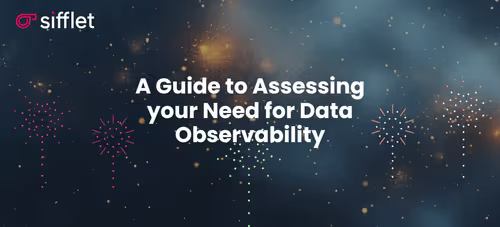
A Guide to Assessing your Need for Data Observability
Every data-driven team dreams of creating "fireworks" with their insights. But without control, the "fire" of the modern data stack can spark chaos.
Discover if you're fighting fires or igniting fireworks:

Data Quality Monitoring is Dead
In this blog, we’ll explore why data quality monitoring falls short and how observability is reshaping the future of data management. Ready to make the shift?

Data monitoring vs observability solutions
Data monitoring and data observability are often used interchangeably, but they serve distinct purposes in managing modern data systems. Understanding the difference is key to choosing the right solution for your organization.
Data Reliability, Reinforced
Sifflet’s monitoring features reinforce data reliability for all users, so business can deliver.
