Built for Scale: How Sifflet outperforms Metaplane
Sifflet offers a more complete and scalable approach to data observability than Metaplane, built for the needs of modern enterprises—not just lean, dbt-centric teams. With deeper lineage, smarter automation, and broader team support, Sifflet helps organizations turn data trust into business impact.
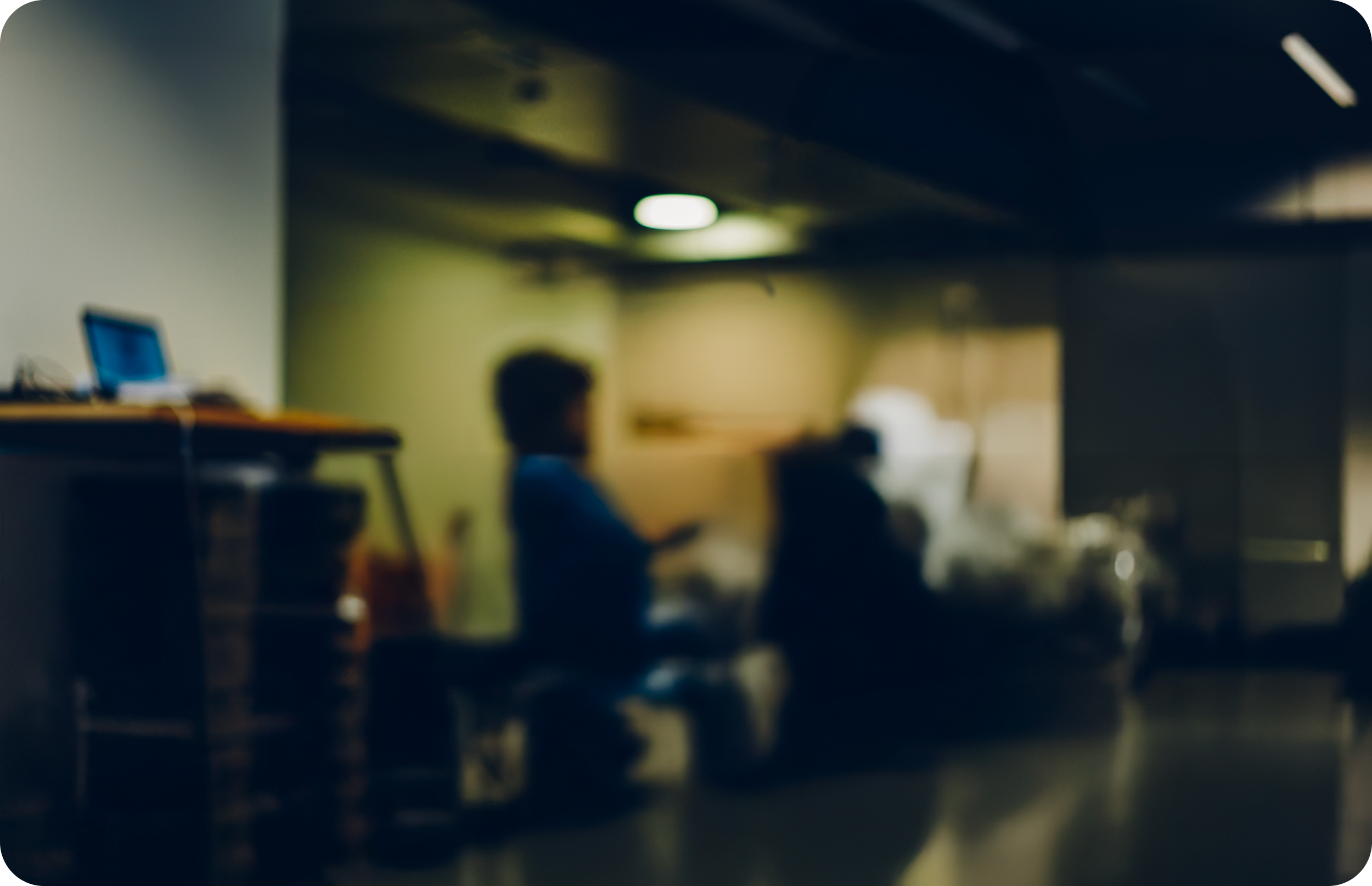
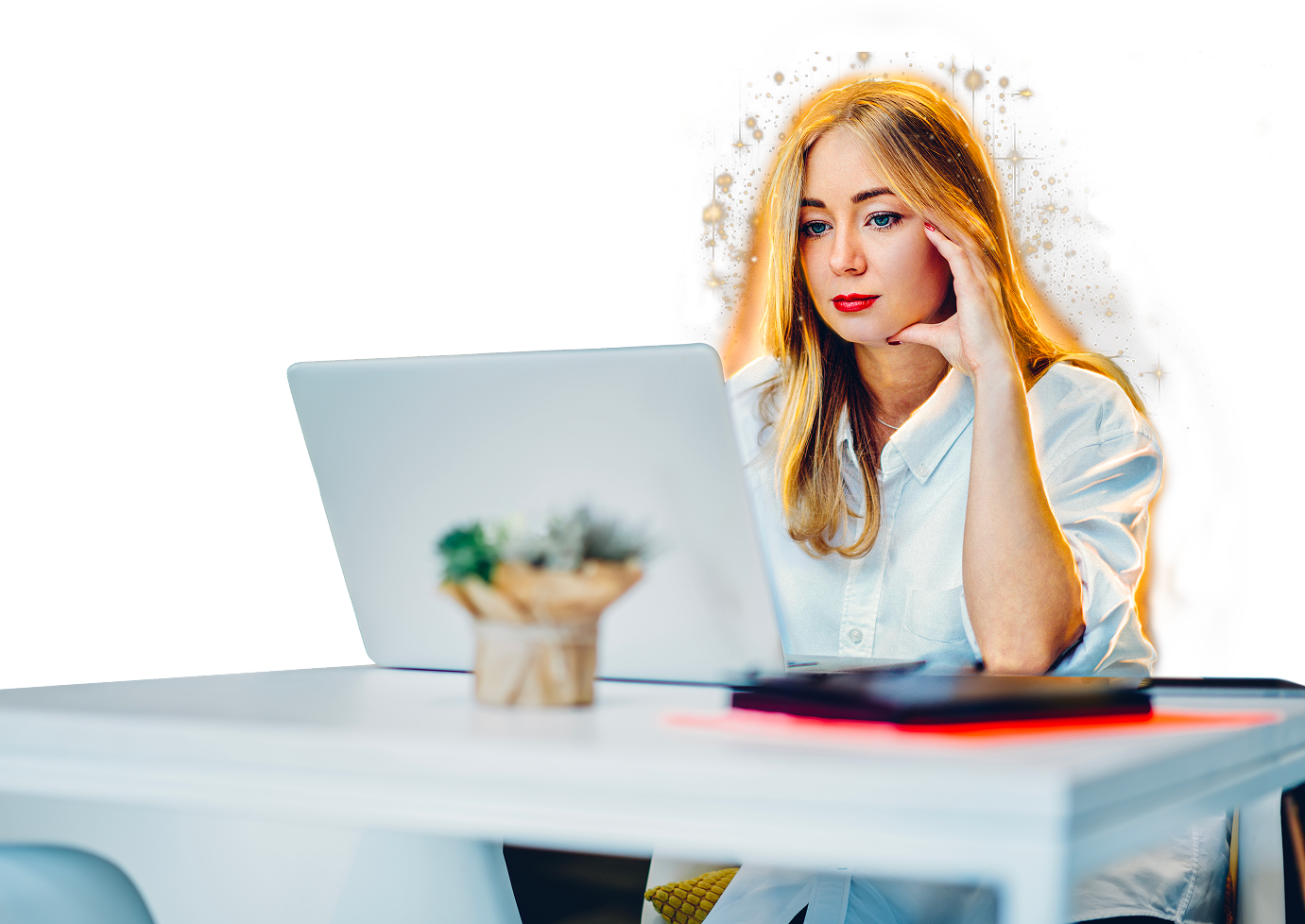

Augmented data quality for analytics and AI
Metaplane covers the basics of technical data quality: freshness, volume, and anomaly detection, mainly for dbt-centric teams. Sifflet goes further, layering rich metadata, lineage, and cataloging to give full visibility and faster resolution across complex data environments.
Built for scale, Sifflet supports both technical and business users with AI-powered automation, broad integrations, and an adaptive UX. It’s observability that drives trust, governance, and business value, not just detection.
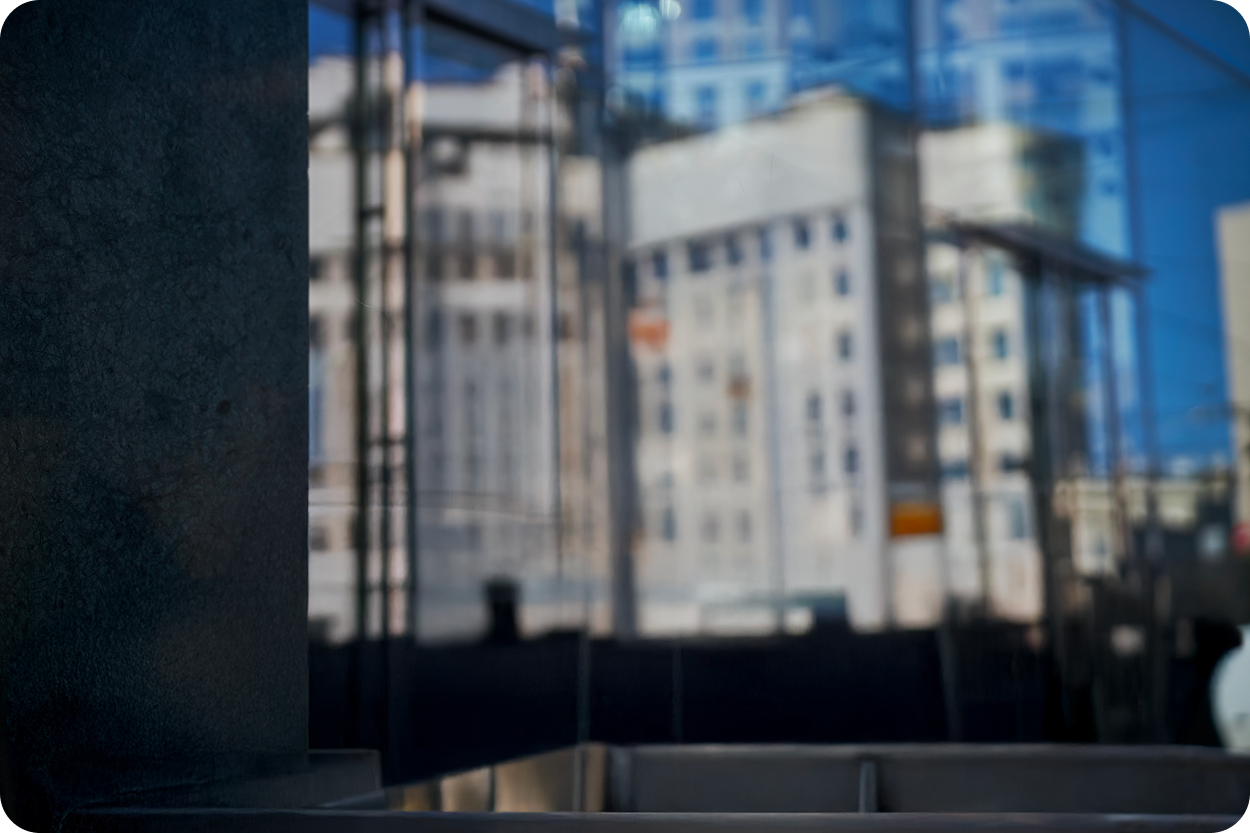
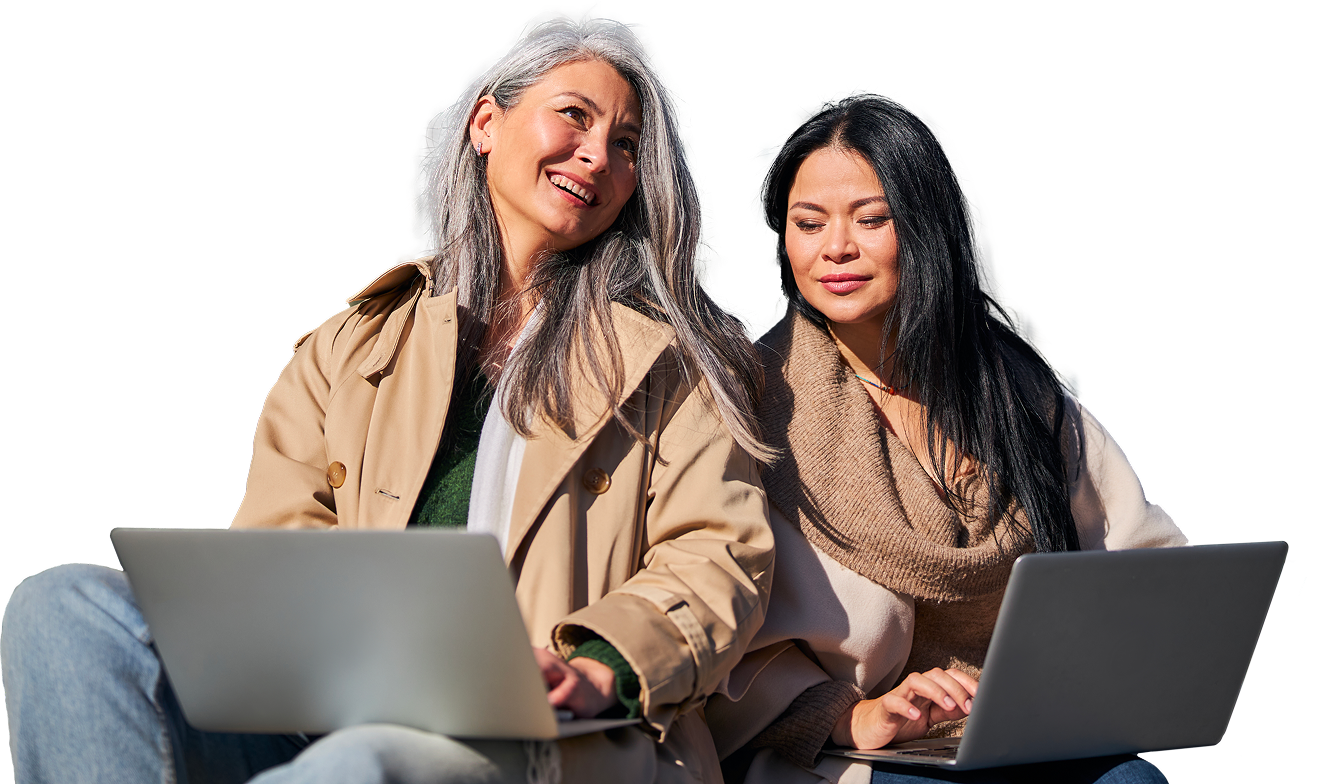

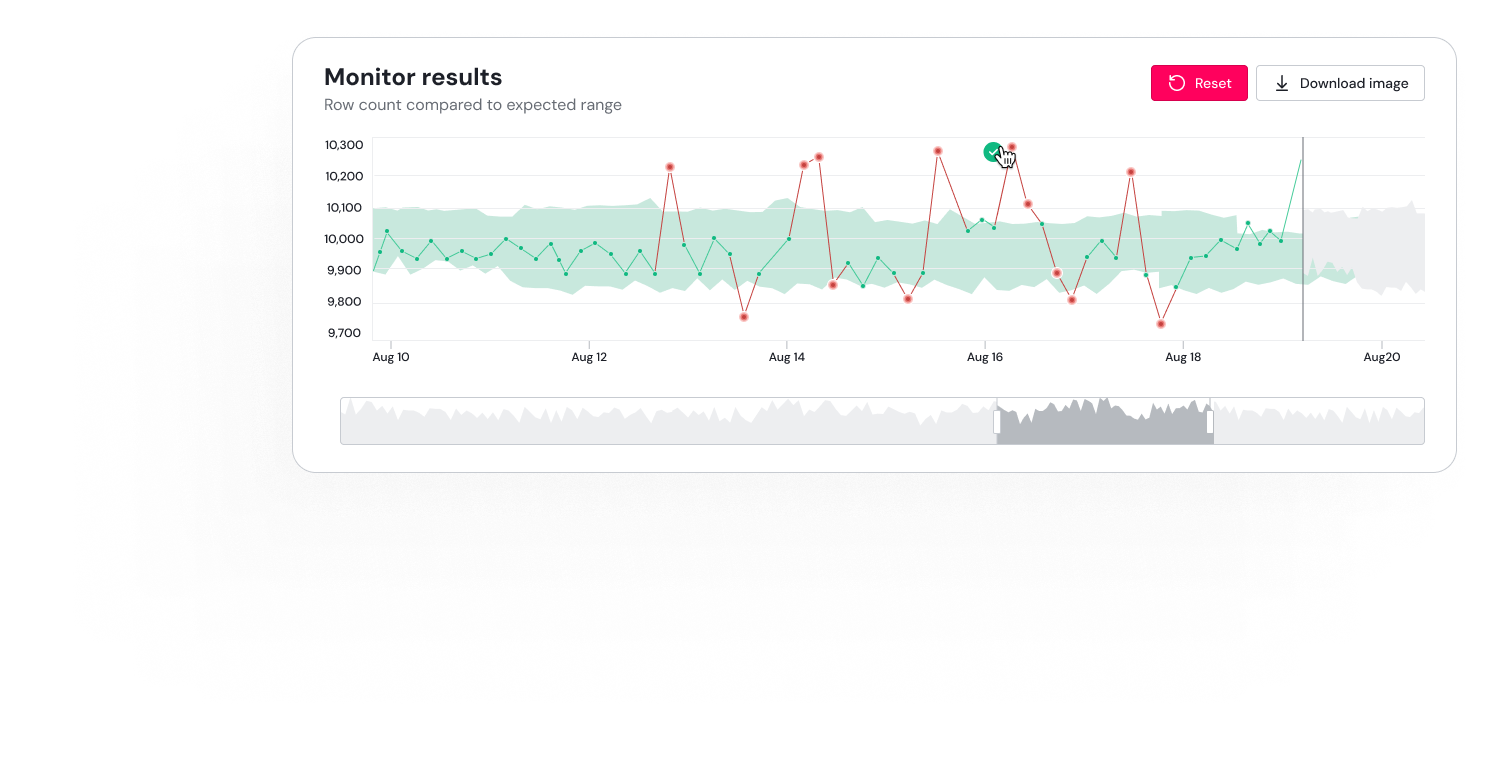
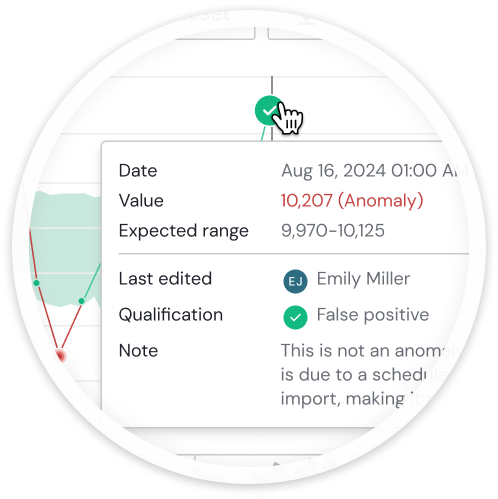
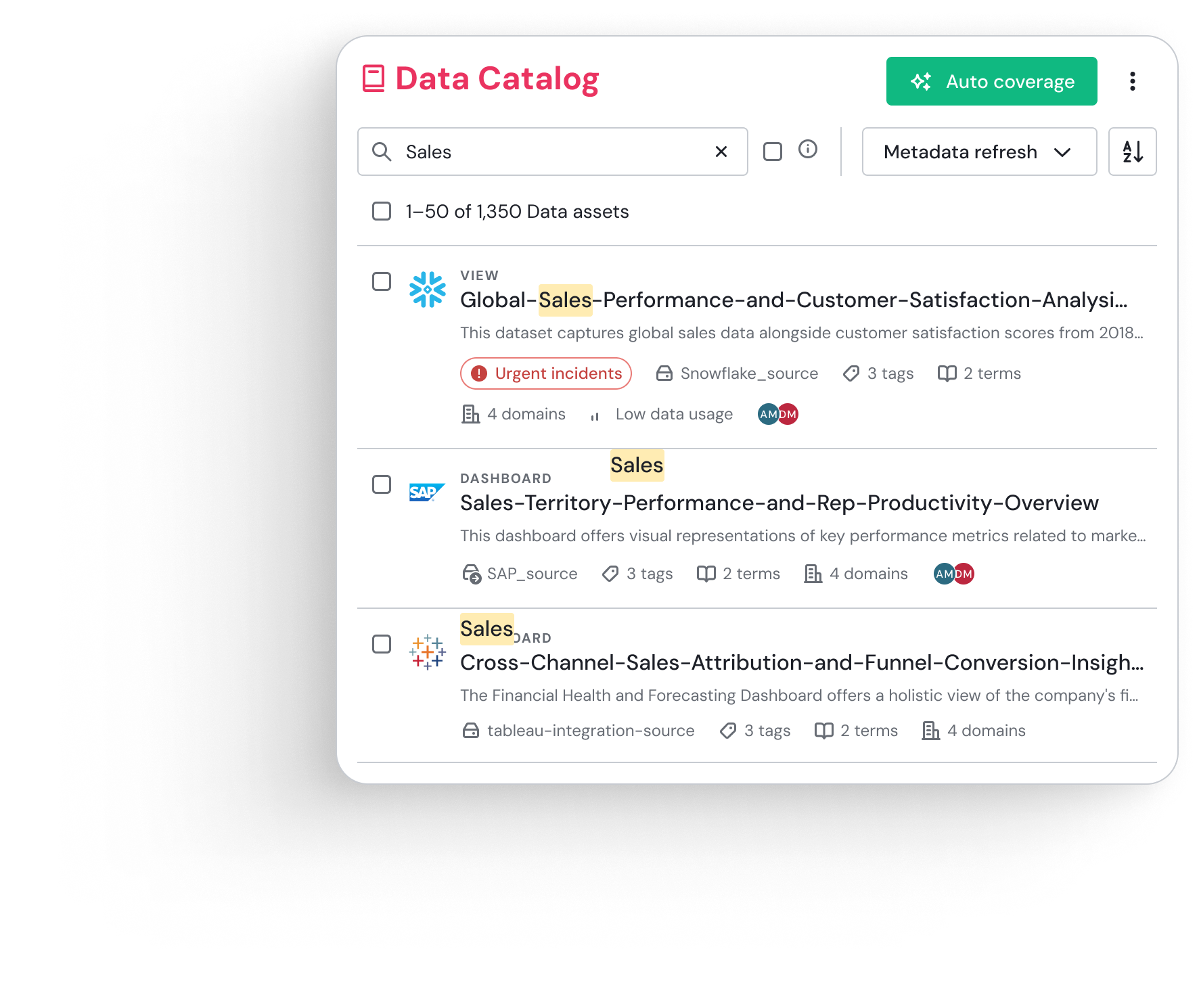
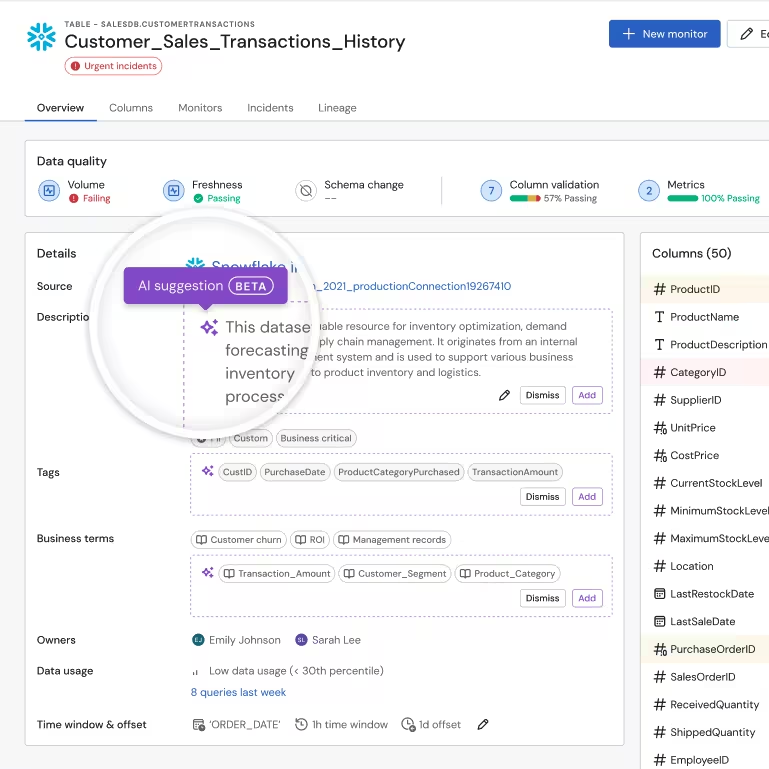

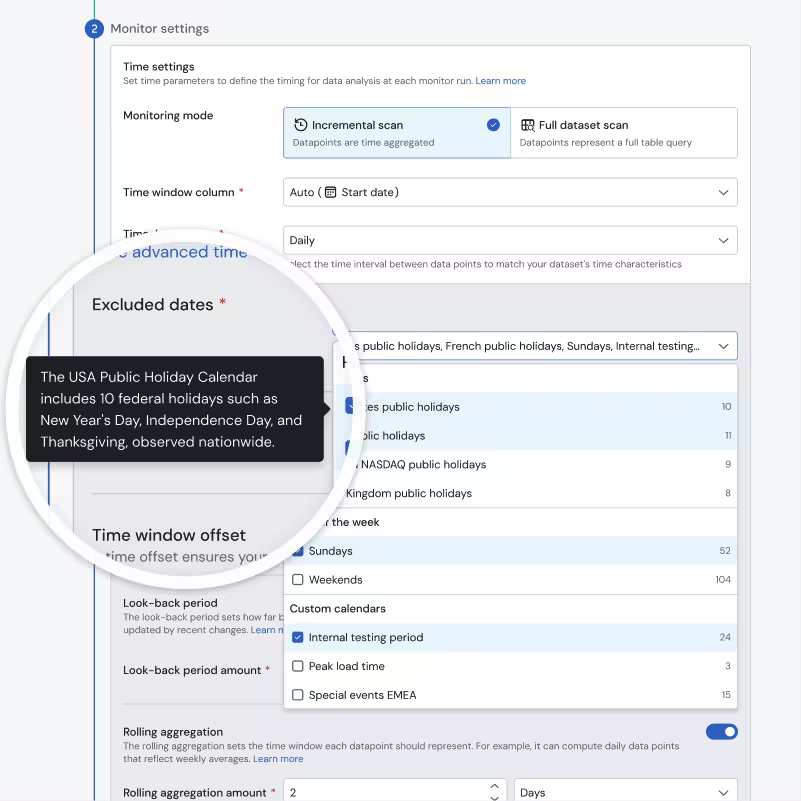
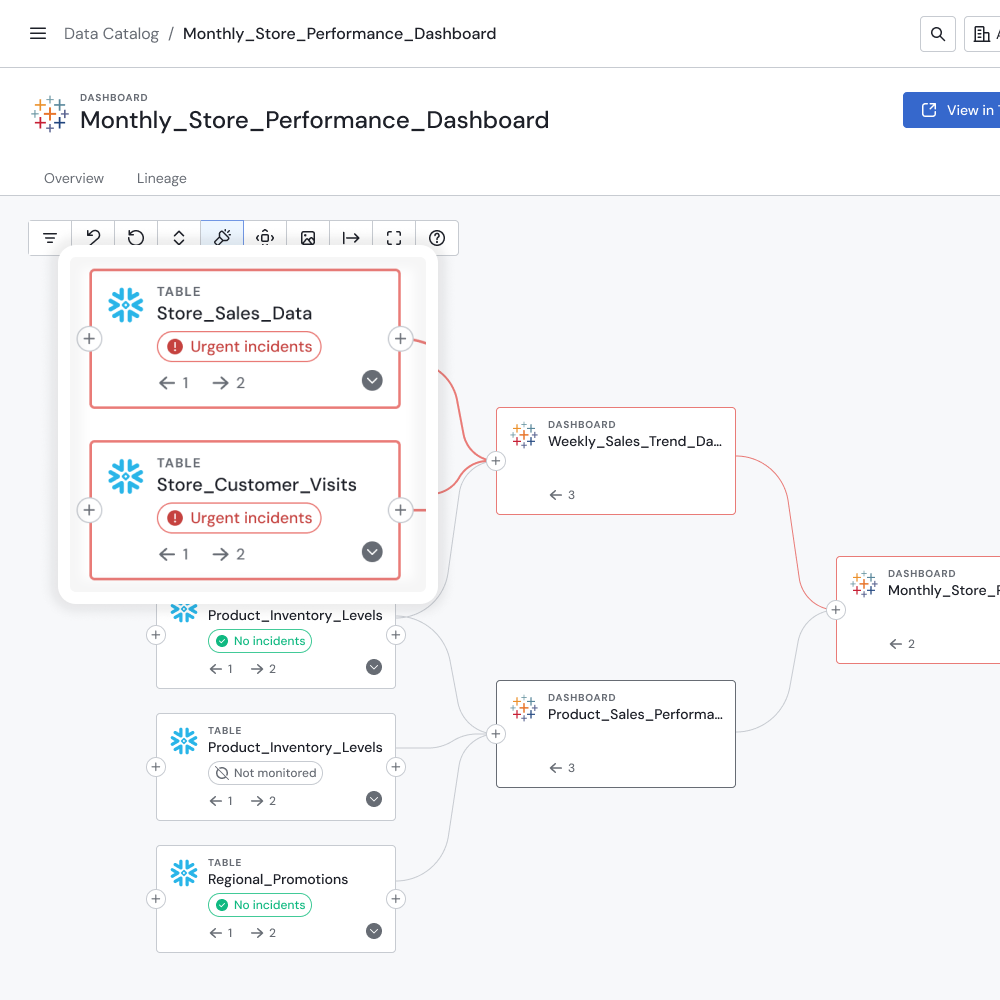
Don't Solve Half the Problem.
If you want to tackle data quality just from a technical perspective, Sifflet isn’t for you. But if you want to reach augmented data quality for analytics and AI that truly brings business value to downstream users, Sifflet is the right choice for today… and tomorrow.
OOTB monitors + SQL logic + NLP monitor wizard; scales across complex environments
Freshness, volume, null checks; dbt-aware
Automated RCA with health-aware lineage and pipeline insights
Manual triage with limited lineage context
End-to-end lineage from ingestion to BI, with health overlays
dbt metadata or warehouse schema-based; partial
Full catalog with glossary, usage tracking, and business context
No built-in catalog; limited metadata visualization
Alerts surface across tools—including BI dashboards via Chrome extension
Slack and email alerts
Adaptive UX for both technical and business users; built for large, decentralized orgs
Simple UI, CLI, fast setup; built for dbt-native, lean teams
Wide coverage across orchestration, warehouse, modeling, and BI tools
Strong in dbt and warehouse tools; limited elsewhere
There's no one size fits all.
When it comes to data observability platforms, there's no one size fits all.
Chat with one of our experts today to learn more about Sifflet and if it's the right option for you.
