See the Whole Picture with Sifflet and Snowflake
Your Snowflake data powers decisions across your business, but when something breaks, it’s more than pipelines at risk. It’s dashboards, AI models, customer reporting, and trust. Sifflet brings business context into your observability layer so you can fix what matters, faster.
Why chose Sifflet for Snowflake?
Your Snowflake data powers decisions across teams, but when quality issues strike, it’s not just pipelines that break. It’s customer experiences, revenue reporting, AI model accuracy, and more.
That’s where Sifflet stands apart.
Sifflet brings business context into the heart of data observability, so you don’t just know what’s broken, you know what matters. Our platform weaves metadata, pipeline behavior, and usage patterns into a unified map of technical and business logic, helping your team spot, triage, and resolve issues before they become downstream disasters.

Deep Integration with Snowflake
Sifflet enhances the observability of your Snowflake stack by letting you:

Prioritize What Matters Most
Not every broken table is worth a PagerDuty alert. Sifflet identifies which anomalies impact key dashboards, SLAs, or ML models, so your team focuses where it counts.
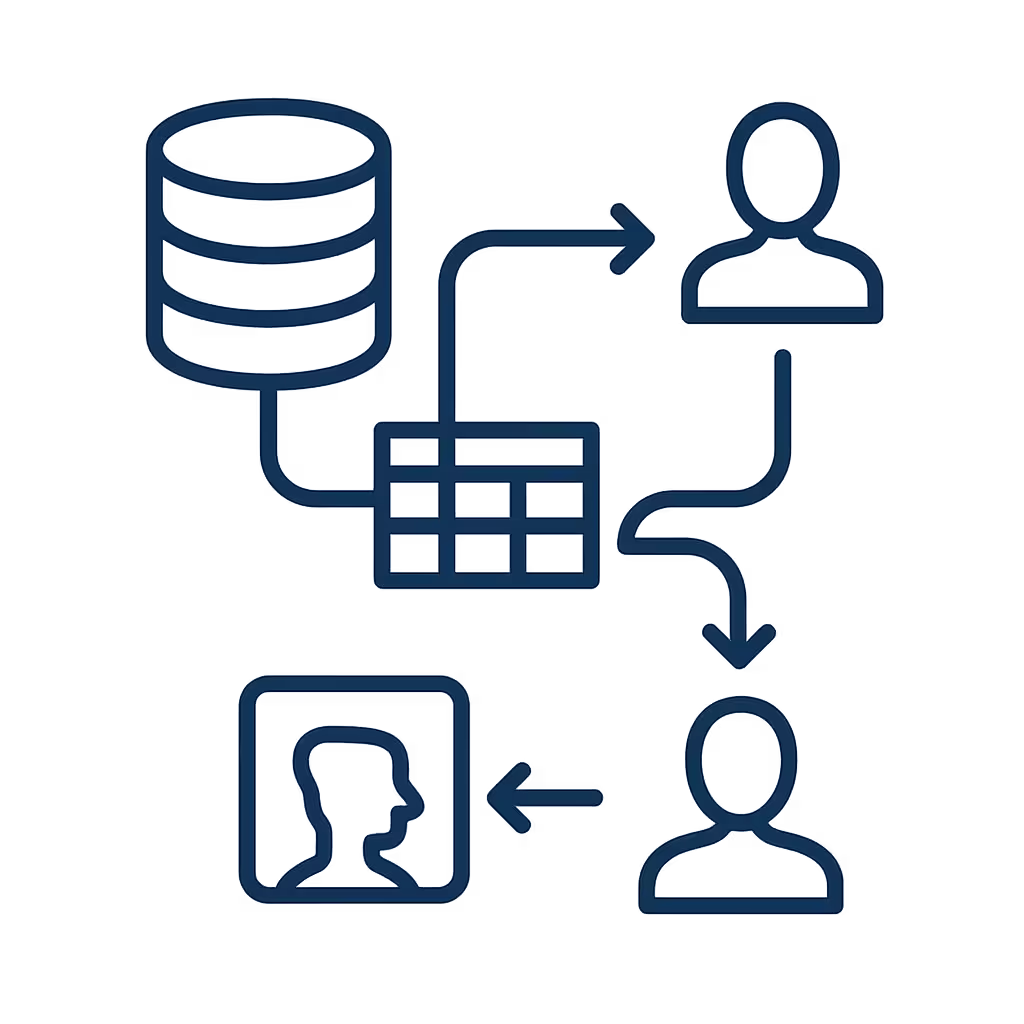
Map Lineage with Business Logic
See how data flows across your stack, not just pipelines, but people. Sifflet combines metadata and usage patterns to show who’s using what, and why. From column to customer.
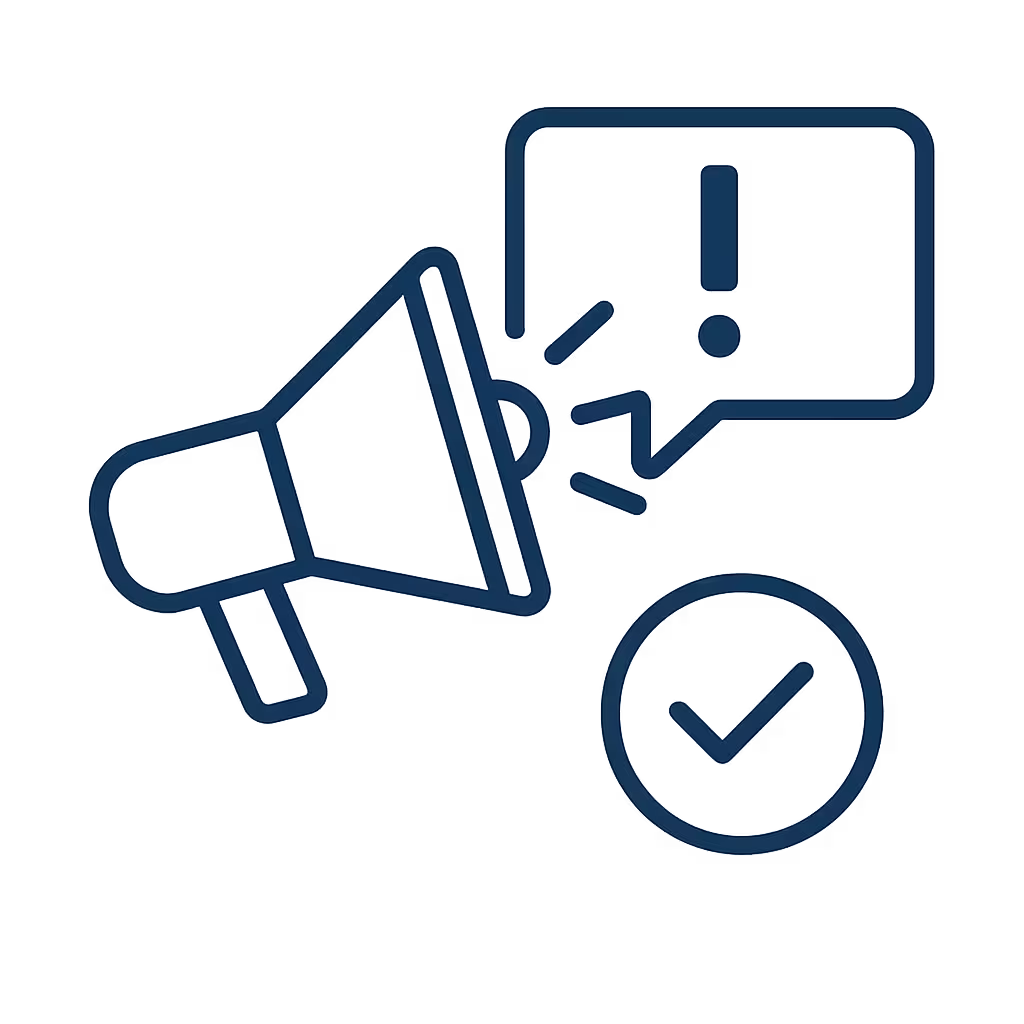
Cut Through the Noise
Sifflet delivers context-rich alerts that combine technical symptoms with business impact. Your team gets fewer false alarms, and faster resolution.

Leverage Time Travel for Smarter Detection
Historical snapshots enhance anomaly detection with temporal intelligence.
Snowflake-specific assets
Sifflet supports multiple Snowflake-specific objects, like streams and stages, for exhaustive coverage.
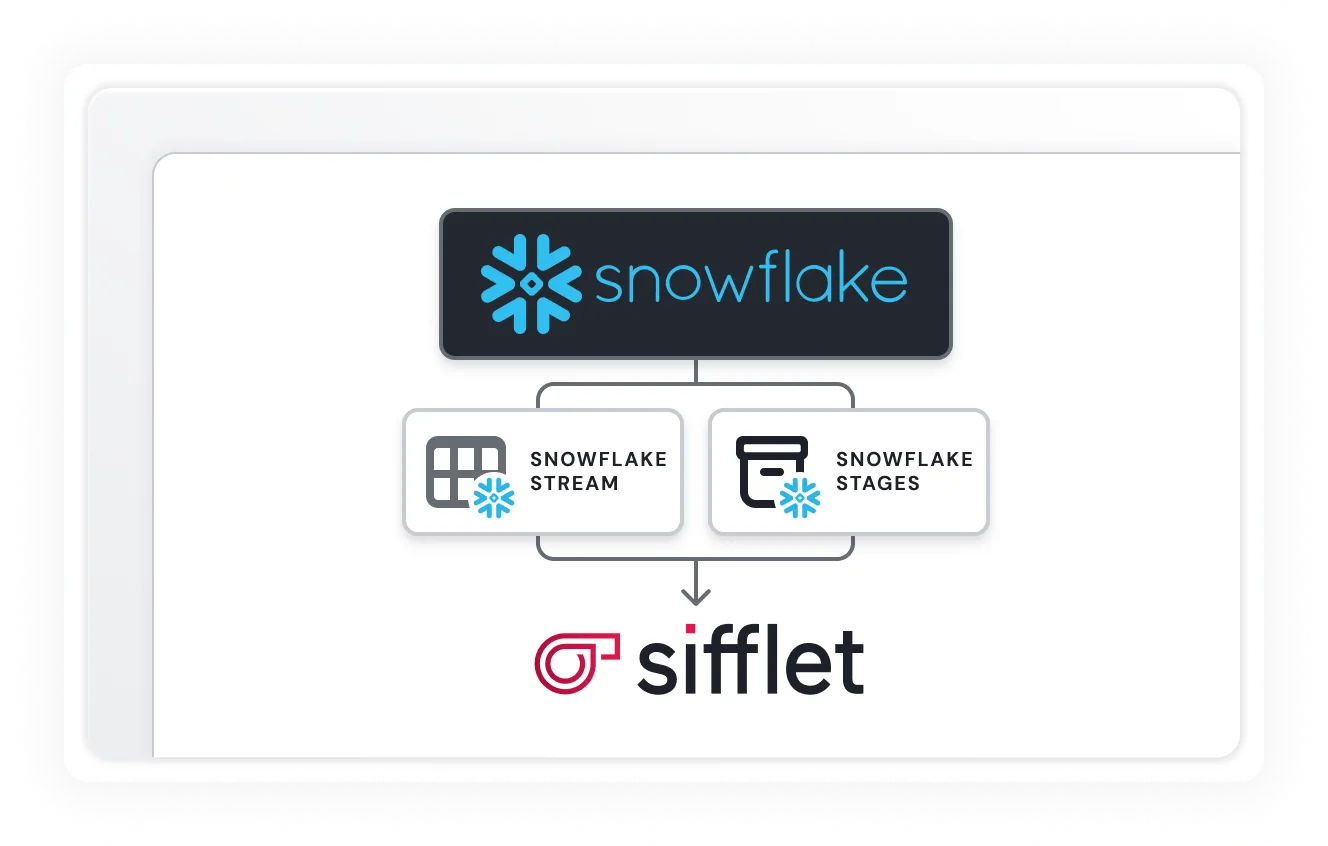
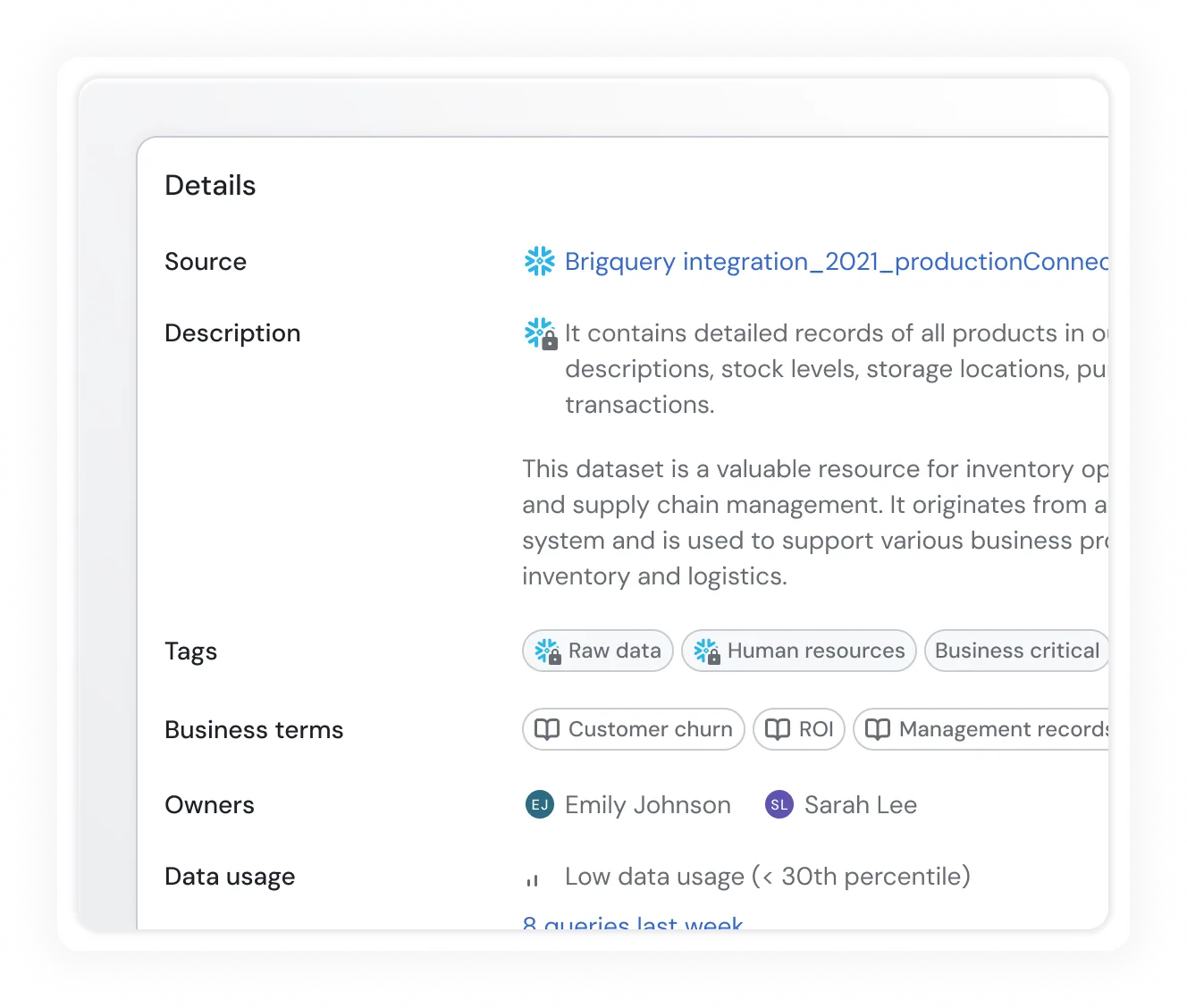
Usage and Snowflake metadata
Get detailed statistics about the usage of your Snowflake assets, in addition to various metadata (like tags, descriptions, and table sizes) retrieved directly from Snowflake.
Field-level lineage
Have a detailed understanding of how data flows through your platform via field-level end-to-end lineage for Snowflake.
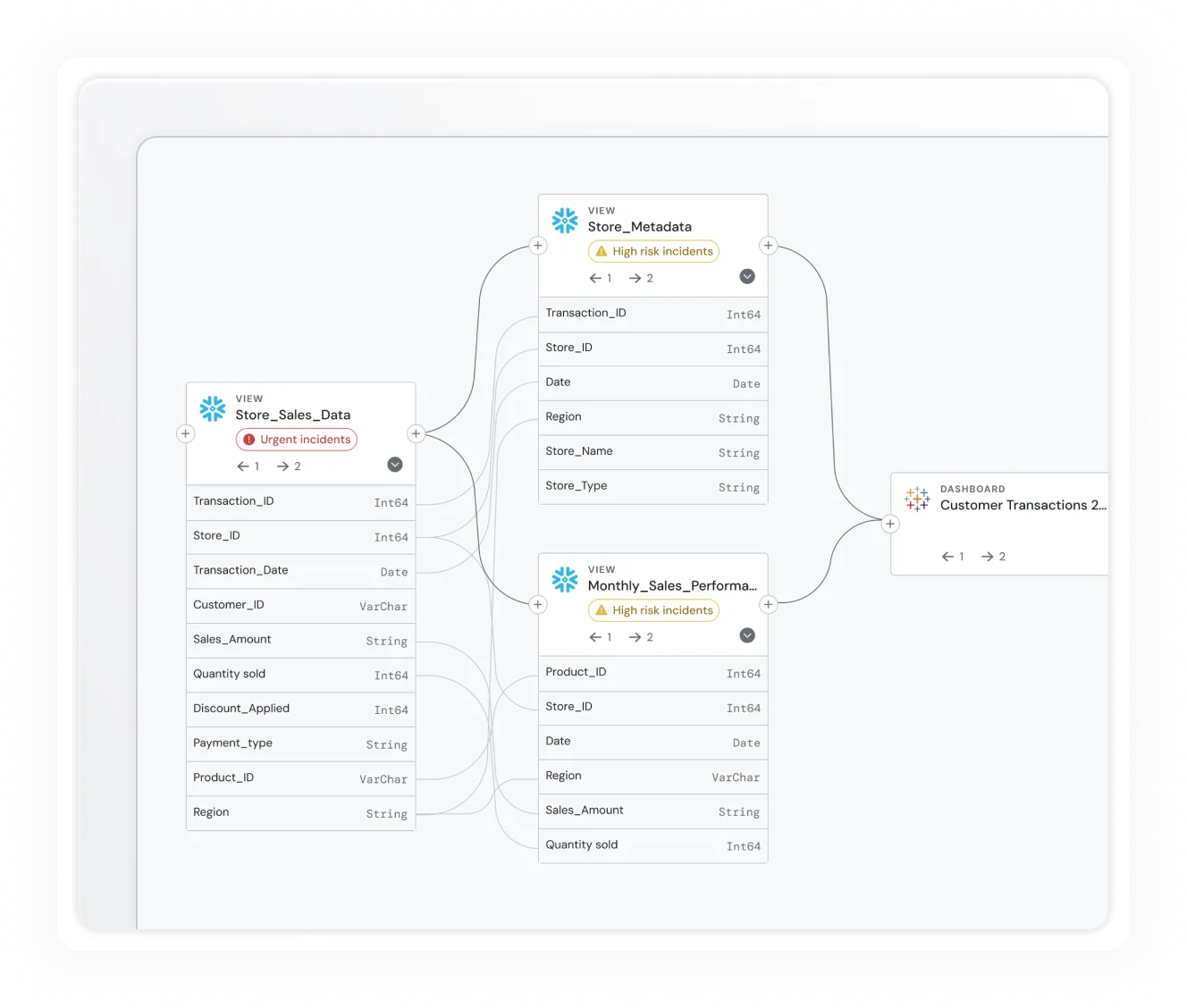
Built for Modern Data Teams on Snowflake
- Trusted by Snowflake-Centric Enterprises Across Europe and the U.S.
- Native integration with Snowflake’s metadata and query engine
- Designed for scale, trust, and business alignment
“With Sifflet, we don’t just detect anomalies in Snowflake. We understand their real-world impact, and we act before anyone downstream even notices.”
Head of Data Governance, European Retail Leader
Perfect For…
- Data Leaders deploying Snowflake as the central nervous system of their organization
- Analytics Teams needing reliable, self-serve dashboards and clear ownership
- Governance & Risk Teams looking to enforce data quality, lineage, and auditability
- AI & ML Teams training models on clean, explainable data they can trust
Go Further

Data Observability for your data stack with Snowflake
Discover the Core Capabilities That Ensure Data Quality in Snowflake
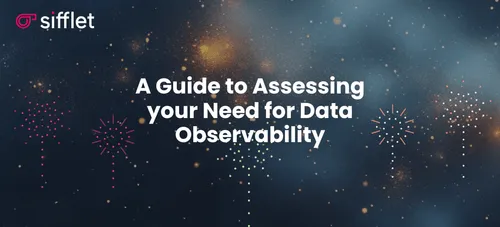
Do You need Data Observability?
Every data-driven team dreams of creating "fireworks" with their insights. But without control, the "fire" of the modern data stack can spark chaos.Discover if you're fighting fires or igniting fireworks:

Frequently asked questions
Want to try Sifflet on your Snowflake Stack?
Get in touch Now