Incident Response Optimization
A Seriously Smart Upgrade.
Prevent, detect and resolve incidents faster than ever before. No matter what your data stack throws at you, your data quality will reach new levels of performance.

No More Over Reacting
Sifflet takes you from reactive to proactive, with real-time detection and alerts that help you to catch data disruptions, before they happen. Watch your mean time to detection fall rapidly. On even the most complex data stacks.
- Advanced capabilities such as multidimensional monitoring help you seize complex data quality issues, even before breaks
- ML-based monitors shield your most business-critical data, so essential KPIs are protected and you get notified before there is business impact
- OOTB and customizable monitors give you comprehensive, end-to-end coverage and AI helps them get smarter as they go, reducing your reactivity even more.

Resolutions in Record Time
Get to the root cause of incidents and resolve them in record time.
- Quickly understand the scope and impact of an incident thanks to detailed system visibility
- Trace data flow through your system, identify the start point of issues, and pinpoint downstream dependencies to enable a seamless experience for business users, all thanks to data lineage
- Halt the propagation of data quality anomalies with Sifflet’s Flow Stopper
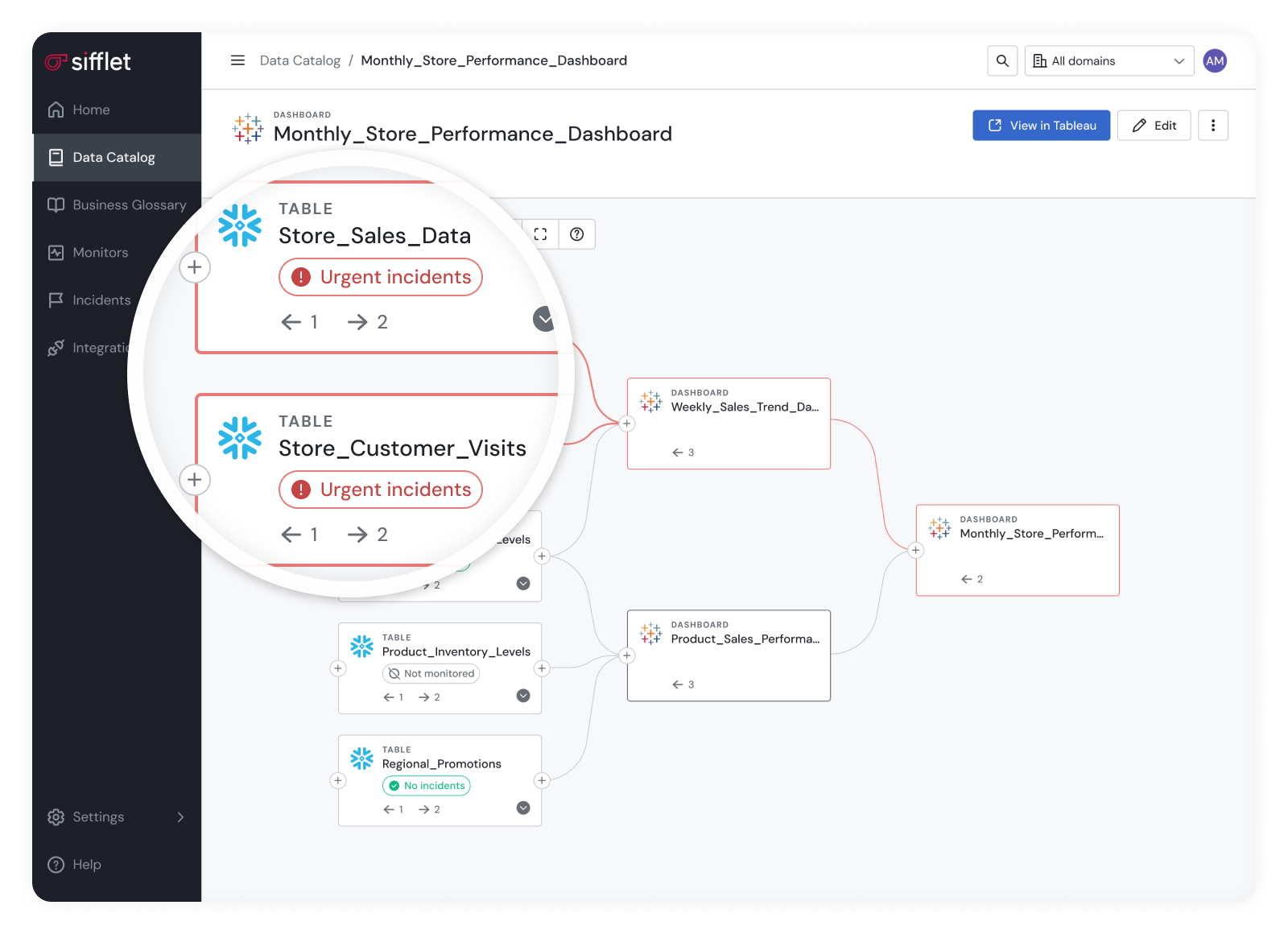

Frequently asked questions
When should companies start implementing data quality monitoring tools?
Ideally, data quality monitoring should begin as early as possible in your data journey. As Dan Power shared during Entropy, fixing issues at the source is far more efficient than tracking down errors later. Early adoption of observability tools helps you proactively catch problems, reduce manual fixes, and improve overall data reliability from day one.
How does Sifflet help with data drift detection in machine learning models?
Great question! Sifflet's distribution deviation monitoring uses advanced statistical models to detect shifts in data at the field level. This helps machine learning engineers stay ahead of data drift, maintain model accuracy, and ensure reliable predictive analytics monitoring over time.
What features should we look for in scalable data observability tools?
When evaluating observability tools, scalability is key. Look for features like real-time metrics, automated anomaly detection, incident response automation, and support for both batch data observability and streaming data monitoring. These capabilities help teams stay efficient as data volumes grow.
What are the key features to look for in a data observability platform?
When evaluating an observability platform, look for strong data lineage tracking, real-time metrics collection, anomaly detection capabilities, and broad integrations across your data stack. Features like field-level lineage, ease of setup, and user-friendly dashboards can make a big difference too. At Sifflet, we believe observability should empower both technical and business users with the context they need to trust and act on data.
How can a data observability tool help when my data is often incomplete or inaccurate?
Great question! If you're constantly dealing with missing values, duplicates, or inconsistent formats, a data observability platform can be a game-changer. It provides real-time metrics and data quality monitoring, so you can detect and fix issues before they impact your reports or decisions.
Can I monitor my BigQuery data with Sifflet?
Absolutely! Sifflet’s observability tools are fully compatible with Google BigQuery, so you can perform data quality monitoring, data lineage tracking, and anomaly detection right where your data lives.
Can data observability support better demand forecasting for retailers?
Absolutely. By integrating historical sales, real-time transactions, and external data sources like weather or social trends, data observability platforms enhance forecast accuracy. They use machine learning to evaluate and adjust predictions, helping retailers align inventory with actual consumer demand more effectively.
How did Sifflet help Meero reduce the time spent on troubleshooting data issues?
Sifflet significantly cut down Meero's troubleshooting time by enabling faster root cause analysis. With real-time alerts and automated anomaly detection, the data team was able to identify and resolve issues in minutes instead of hours, saving up to 50% of their time.