Cloud migration monitoring
Mitigate disruption and risks
Optimize the management of data assets during each stage of a cloud migration.
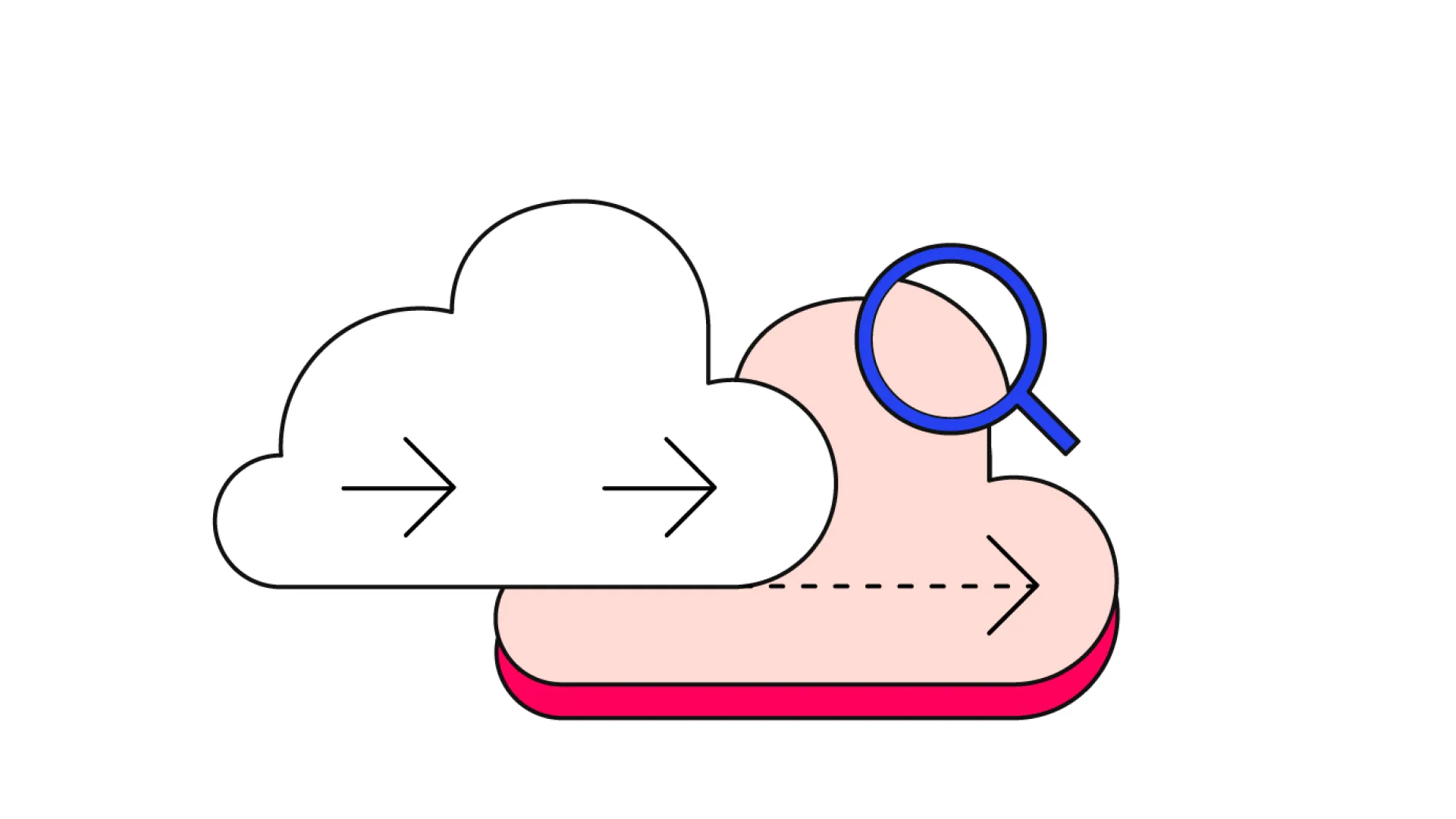
Before migration
- Go through an inventory of what needs to be migrated using the Data Catalog
- Identify the most critical assets to prioritize migration efforts based on actual asset usage
- Leverage lineage to identify downstream impact of the migration in order to plan accordingly
.webp)
During migration
- Use the Data Catalog to confirm all the data was backed up appropriately
- Ensure the new environment matches the incumbent via dedicated monitors

After migration
- Swiftly document and classify new pipelines thanks to Sifflet AI Assistant
- Define data ownership to improve accountability and simplify maintenance of new data pipelines
- Monitor new pipelines to ensure the robustness of data foundations over time
- Leverage lineage to better understand newly built data flows
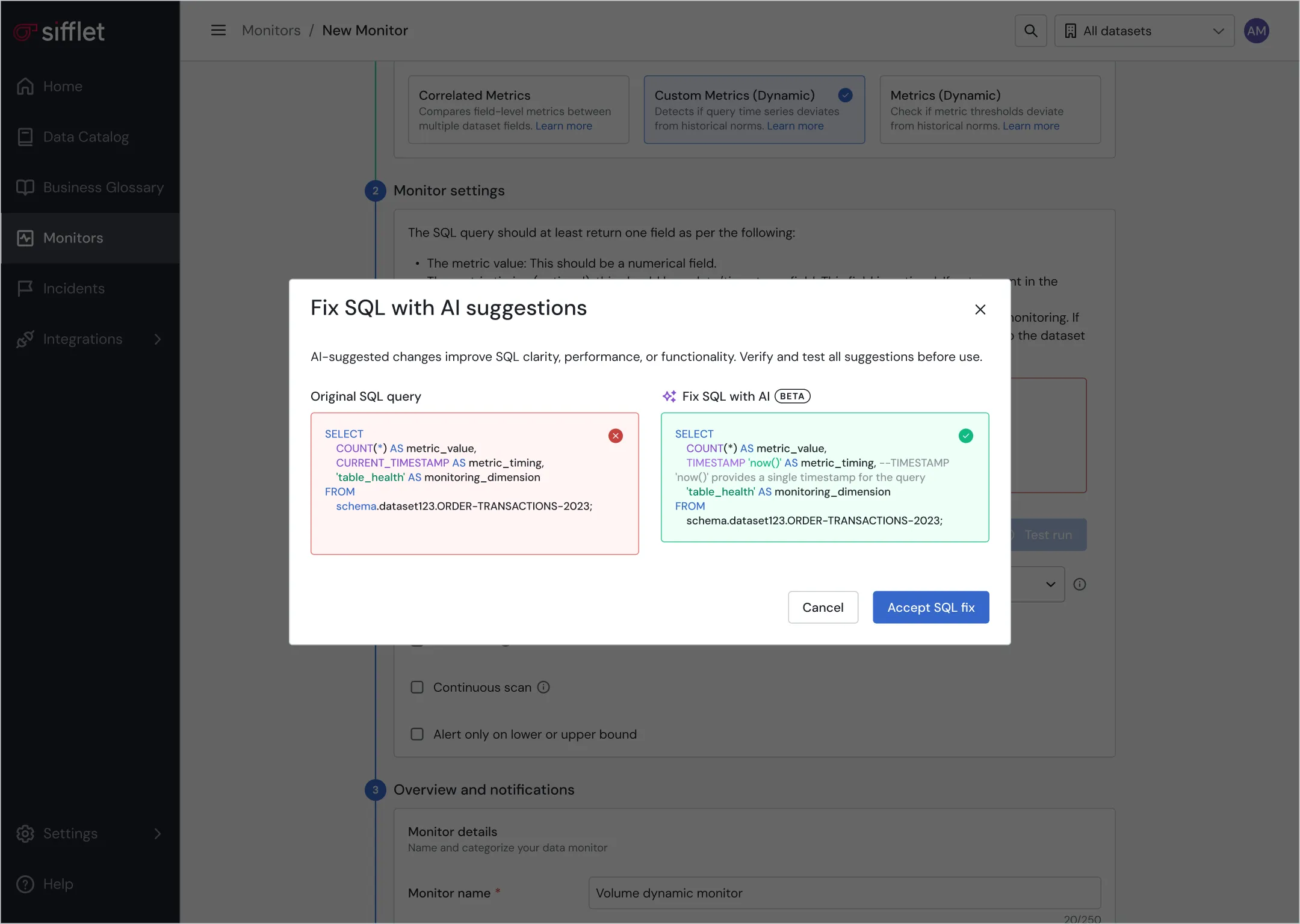

Frequently asked questions
When should companies start implementing data quality monitoring tools?
Ideally, data quality monitoring should begin as early as possible in your data journey. As Dan Power shared during Entropy, fixing issues at the source is far more efficient than tracking down errors later. Early adoption of observability tools helps you proactively catch problems, reduce manual fixes, and improve overall data reliability from day one.
What trends in data observability should we watch for in 2025?
In 2025, expect to see more focus on AI-driven anomaly detection, dynamic thresholding, and predictive analytics monitoring. Staying ahead means experimenting with new observability tools, engaging with peers, and continuously aligning your data strategy with evolving business needs.
How does Sifflet Insights help improve data quality in my BI dashboards?
Sifflet Insights integrates directly into your BI tools like Looker and Tableau, providing real-time alerts about upstream data quality issues. This ensures you always have accurate and reliable data for your reports, which is essential for maintaining data trust and improving data governance.
How can data observability support better hiring decisions for data teams?
When you prioritize data observability, you're not just investing in tools, you're building a culture of transparency and accountability. This helps attract top-tier Data Engineers and Analysts who value high-quality pipelines and proactive monitoring. Embedding observability into your workflows also empowers your team with root cause analysis and pipeline health dashboards, helping them work more efficiently and effectively.
What is SQL Table Tracer and how does it help with data observability?
SQL Table Tracer (STT) is a lightweight library that extracts table-level lineage from SQL queries. It plays a key role in data observability by identifying upstream and downstream tables, making it easier to understand data dependencies and track changes across your data pipelines.
What are some key features to look for in an observability platform for data?
A strong observability platform should offer data lineage tracking, real-time metrics, anomaly detection, and data freshness checks. It should also integrate with your existing tools like Airflow or Snowflake, and support alerting through Slack or webhook integrations. These capabilities help teams monitor data pipelines effectively and respond quickly to issues.
Why is a user-friendly interface important in an observability tool?
A user-friendly interface boosts adoption across teams and makes it easier to navigate complex datasets. For observability tools, especially those focused on data cataloging and data discovery, a clean UI enables faster insights and more efficient collaboration.
Why are retailers turning to data observability to manage inventory better?
Retailers are adopting data observability to gain real-time visibility into inventory across all channels, reduce stock inaccuracies, and avoid costly misalignments between supply and demand. With data observability tools, they can proactively detect issues, monitor data quality, and improve operational efficiency across their data pipelines.