Monitoring at Scale
Coverage without compromise.
Grow monitoring coverage intelligently as your stack scales and do more with less resources thanks to tooling that reduces maintenance burden, improves signal-to-noise, and helps you understand impact across interconnected systems.
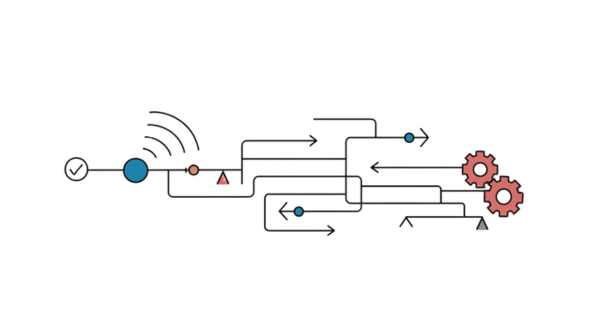
Don’t Let Scale Stop You
As your stack and data assets scale, so do monitors. Keeping rules updated becomes a full-time job, and tribal knowledge about monitors gets scattered, so teams struggle to sunset obsolete monitors while adding new ones. No more with Sifflet.
- Optimize monitoring coverage and minimize noise levels with AI-powered suggestions and supervision that adapt dynamically
- Implement programmatic monitoring set up and maintenance with Data Quality as Code (DQaC)
- Automated monitor creation and updates based on data changes
- Centralized monitor management reduces maintenance overhead

Get Clear and Consistent
Maintaining consistent monitoring practices across tools, platforms, and internal teams that work across different parts of the stack isn’t easy. Sifflet makes it a breeze.
- Set up consistent alerting and response workflows
- Benefit from unified monitoring across your platforms and tools
- Use automated dependency mapping to show system relationships and benefit from end-to-end visibility across the entire data pipeline
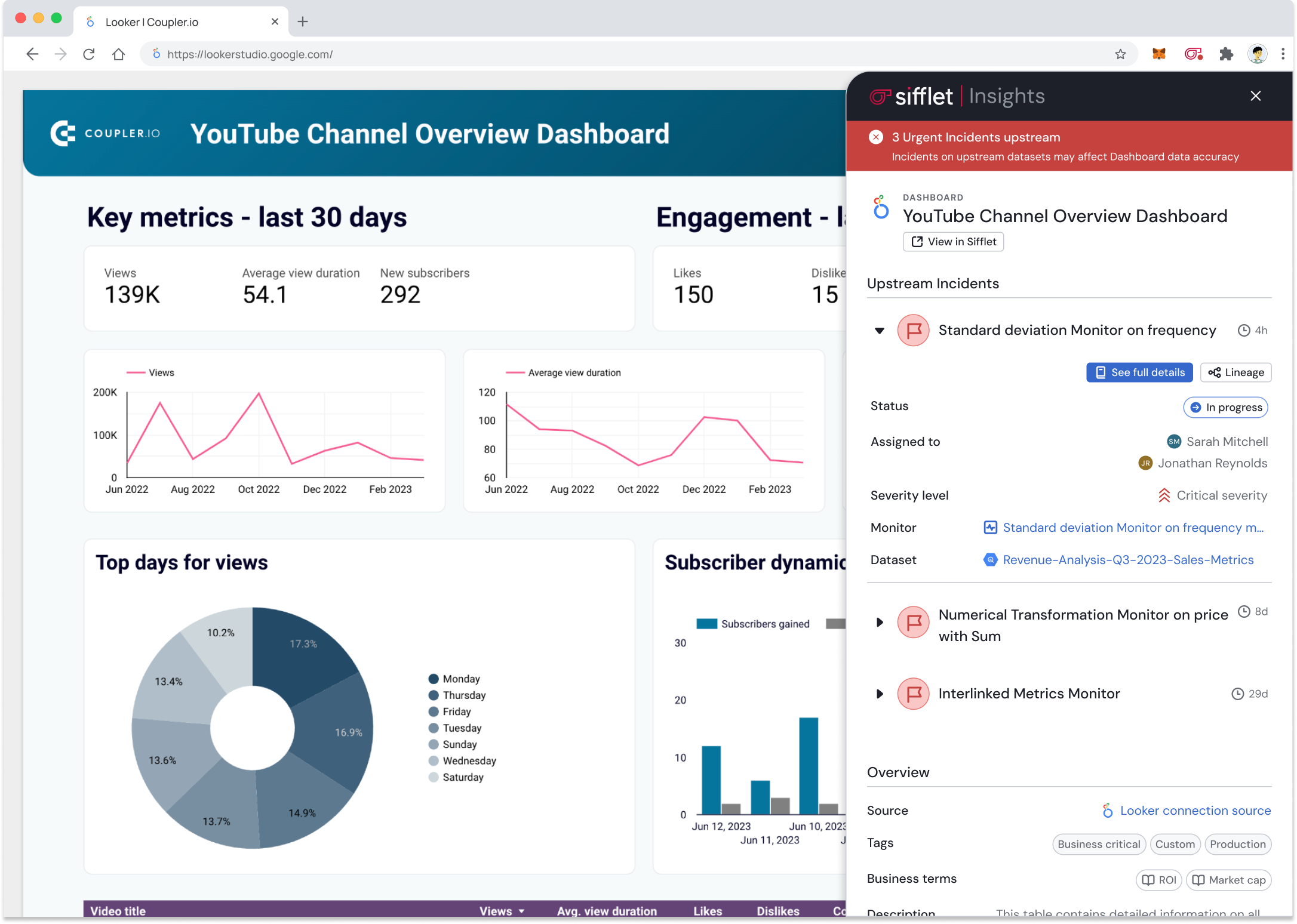

Frequently asked questions
How can I track the success of my data team?
Define clear success KPIs that support ROI, such as improvements in SLA compliance, reduction in ingestion latency, or increased data reliability. Using data observability dashboards and pipeline health metrics can help you monitor progress and communicate value to stakeholders. It's also important to set expectations early and maintain strong internal communication.
Where can I find Sifflet at Big Data LDN 2024?
You can find the Sifflet team at Booth Y640 during Big Data LDN on September 18-19. Stop by to learn more about our data observability platform and how we’re helping organizations like the BBC and Penguin Random House improve their data reliability.
What role does metadata play in a data observability platform?
Metadata provides context about your data, such as who created it, when it was modified, and how it's classified. In a data observability platform, strong metadata management enhances data discovery, supports compliance monitoring, and ensures consistent, high-quality data across systems.
Why is data observability important during the data integration process?
Data observability is key during data integration because it helps detect issues like schema changes or broken APIs early on. Without it, bad data can flow downstream, impacting analytics and decision-making. At Sifflet, we believe observability should start at the source to ensure data reliability across the whole pipeline.
How does Sifflet support data documentation in Airflow?
Sifflet centralizes documentation for all your data assets, including DAGs, models, and dashboards. This makes it easier for teams to search, explore dependencies, and maintain strong data governance practices.
What is SQL Table Tracer and how does it help with data observability?
SQL Table Tracer (STT) is a lightweight library that extracts table-level lineage from SQL queries. It plays a key role in data observability by identifying upstream and downstream tables, making it easier to understand data dependencies and track changes across your data pipelines.
What makes Sifflet’s approach to data observability unique?
Our approach stands out because we treat data observability as both an engineering and organizational concern. By combining telemetry instrumentation, root cause analysis, and business KPI tracking, we help teams align technical reliability with business outcomes.
How does Sifflet help reduce alert fatigue in data observability?
Sifflet uses AI-driven context and dynamic thresholding to prioritize alerts based on impact and relevance. Its intelligent alerting system ensures users only get notified when it truly matters, helping reduce alert fatigue and enabling faster, more focused incident response.