The Ultimate Observability Duo for the Modern Data Stack
With Sifflet fully integrated into your Databricks environment, your data teams gain end-to-end visibility, AI-powered monitoring, and business-context awareness, without compromising performance.
Why Choose Sifflet for Databricks?
Modern organizations rely on Databricks to unify data engineering, machine learning, and analytics. But as the platform grows in complexity, new risks emerge:
- Broken pipelines that go unnoticed
- Data quality issues that erode trust
- Limited visibility across orchestration and workflows
That’s where Sifflet comes in. Our native integration with Databricks ensures your data pipelines are transparent, reliable, and business-aligned, at scale.
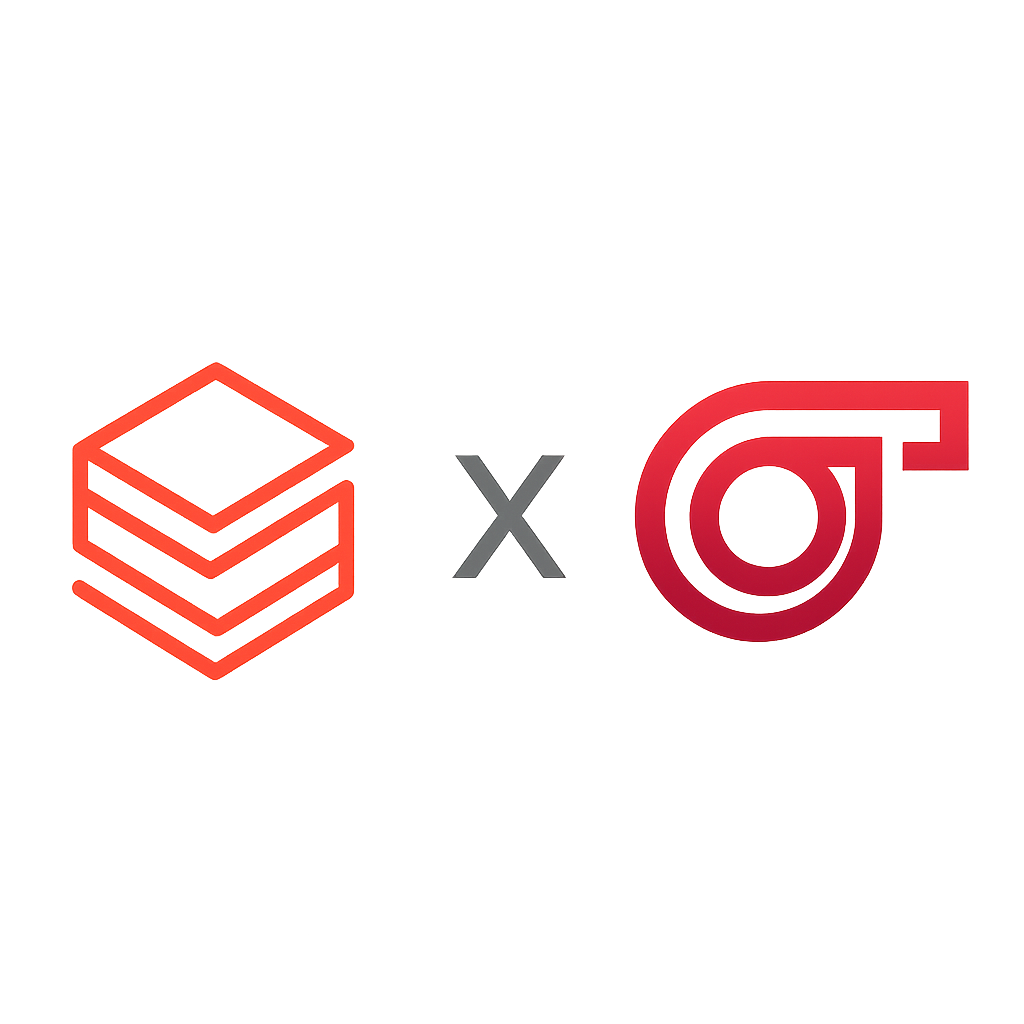
Deep Integration with Databricks
Sifflet enhances the observability of your Databricks stack across:
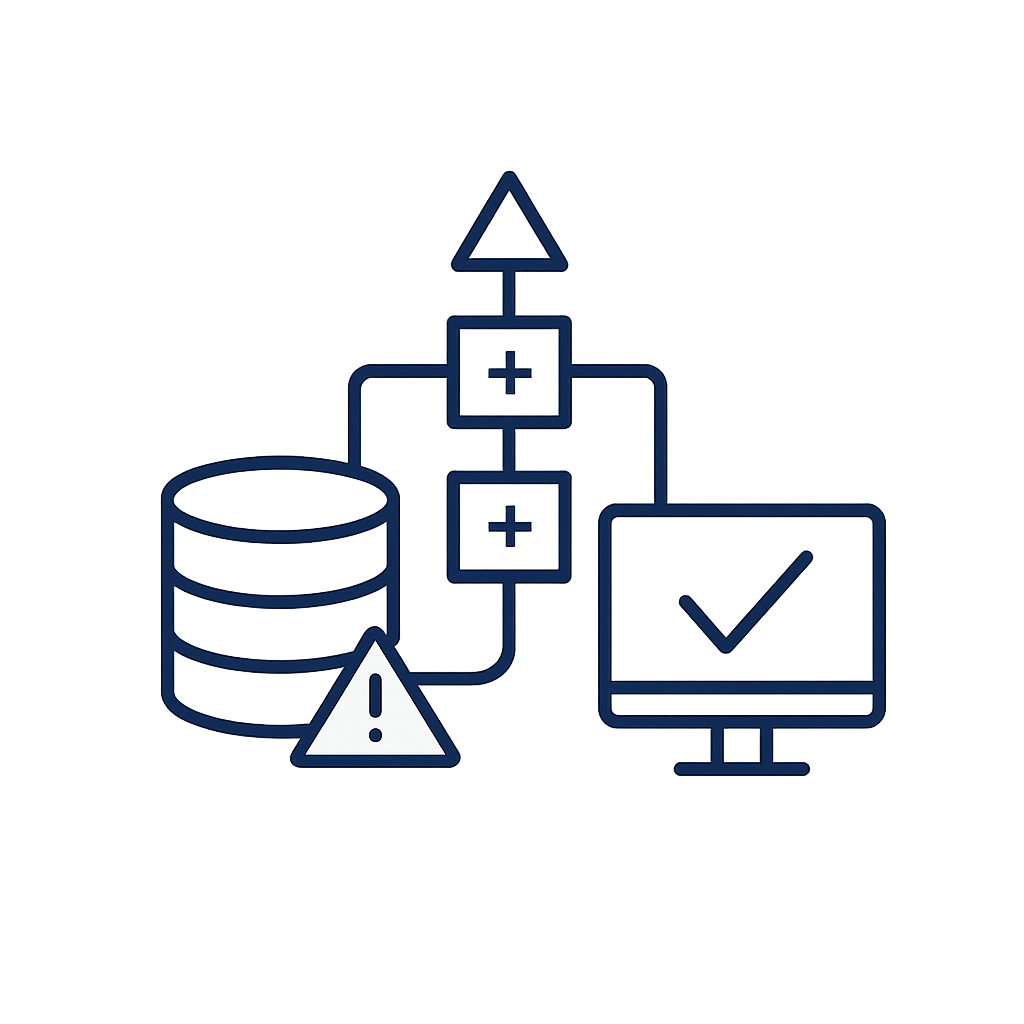
Delta Pipelines & DLT
Monitor transformation logic, detect broken jobs, and ensure SLAs are met across streaming and batch workflows.
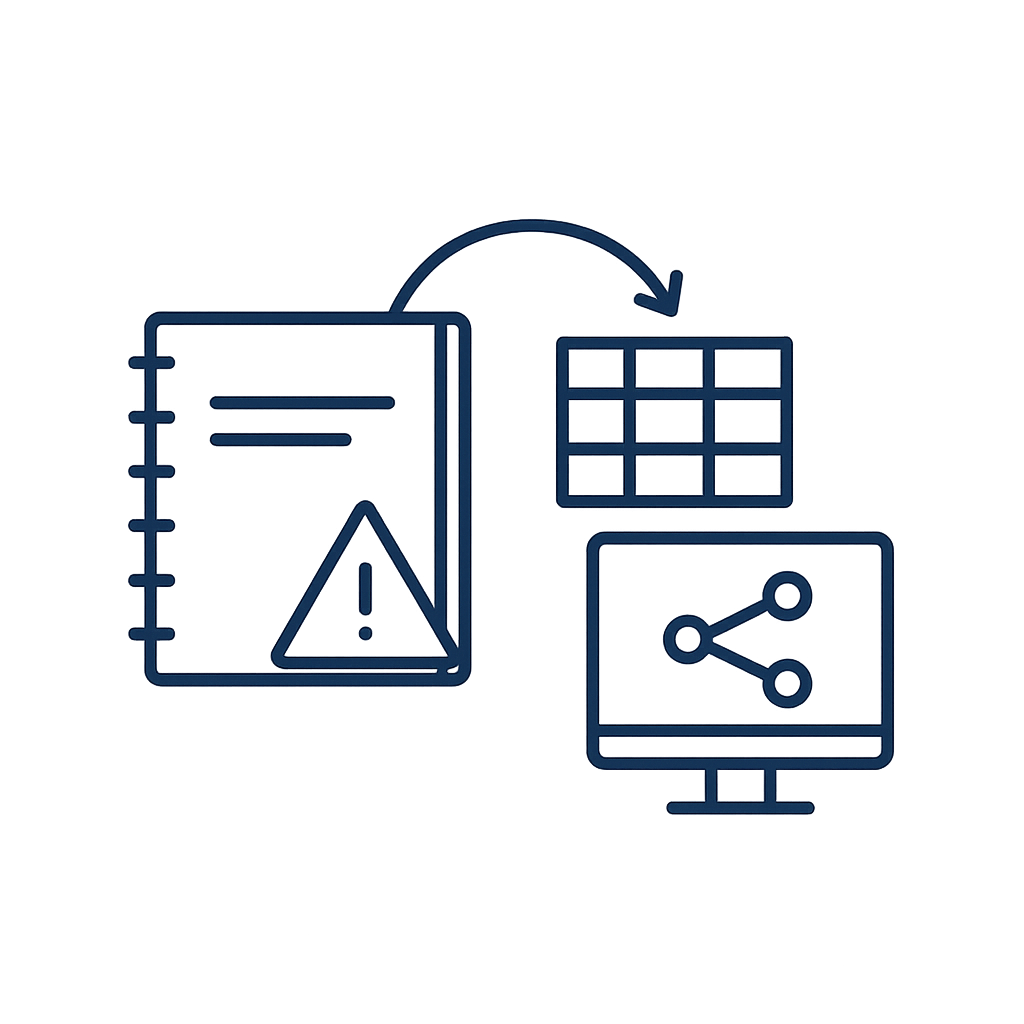
Notebooks & ML Models
Trace data quality issues back to the tables or features powering production models.
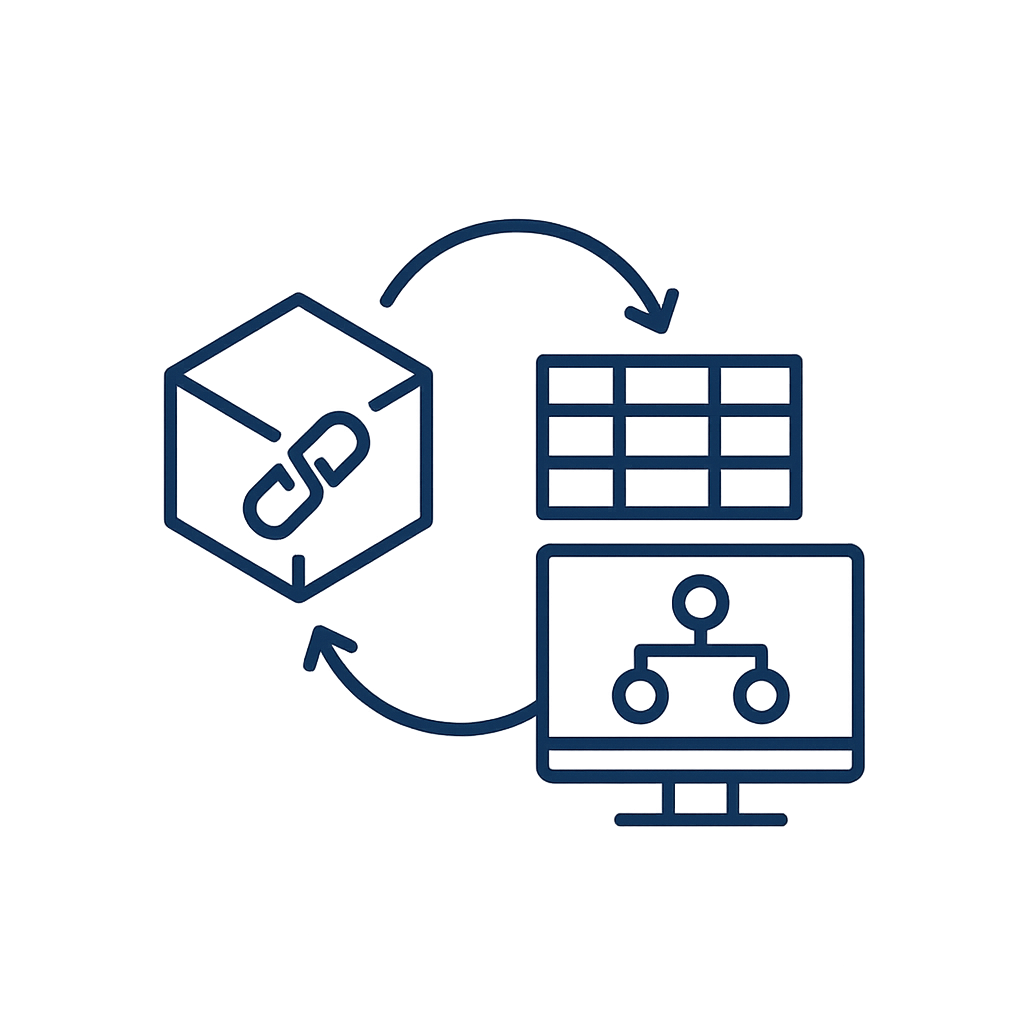
Unity Catalog & Lakehouse Metadata
Integrate catalog metadata into observability workflows, enriching alerts with ownership and context.
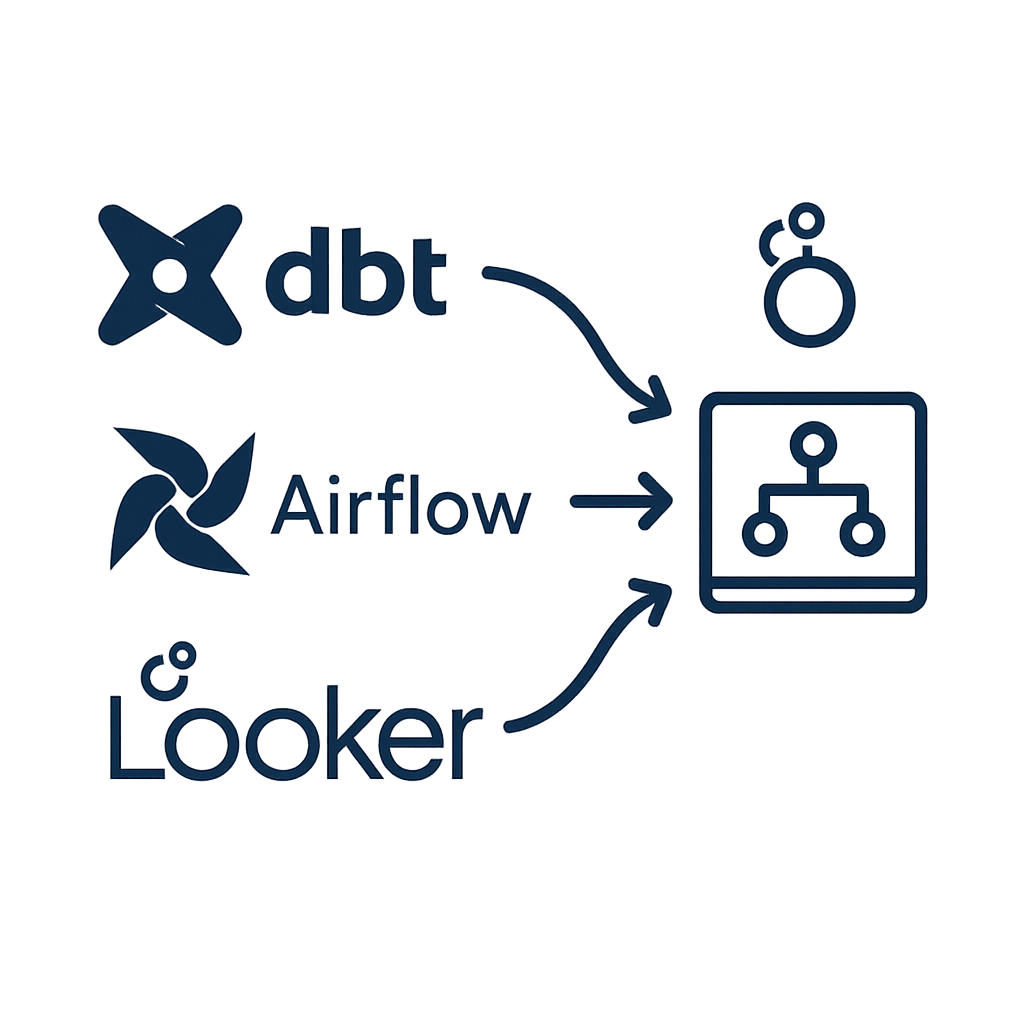
Cross-Stack Connectivity
Sifflet integrates with dbt, Airflow, Looker, and more, offering a single observability layer that spans your entire lakehouse ecosystem.
End-to-End Data Observability
- Full monitoring across the data lifecycle: from raw ingestion in Databricks to BI consumption
- Real-time alerts for freshness, volume, nulls, and schema changes
- AI-powered prioritization so teams focus on what really matters

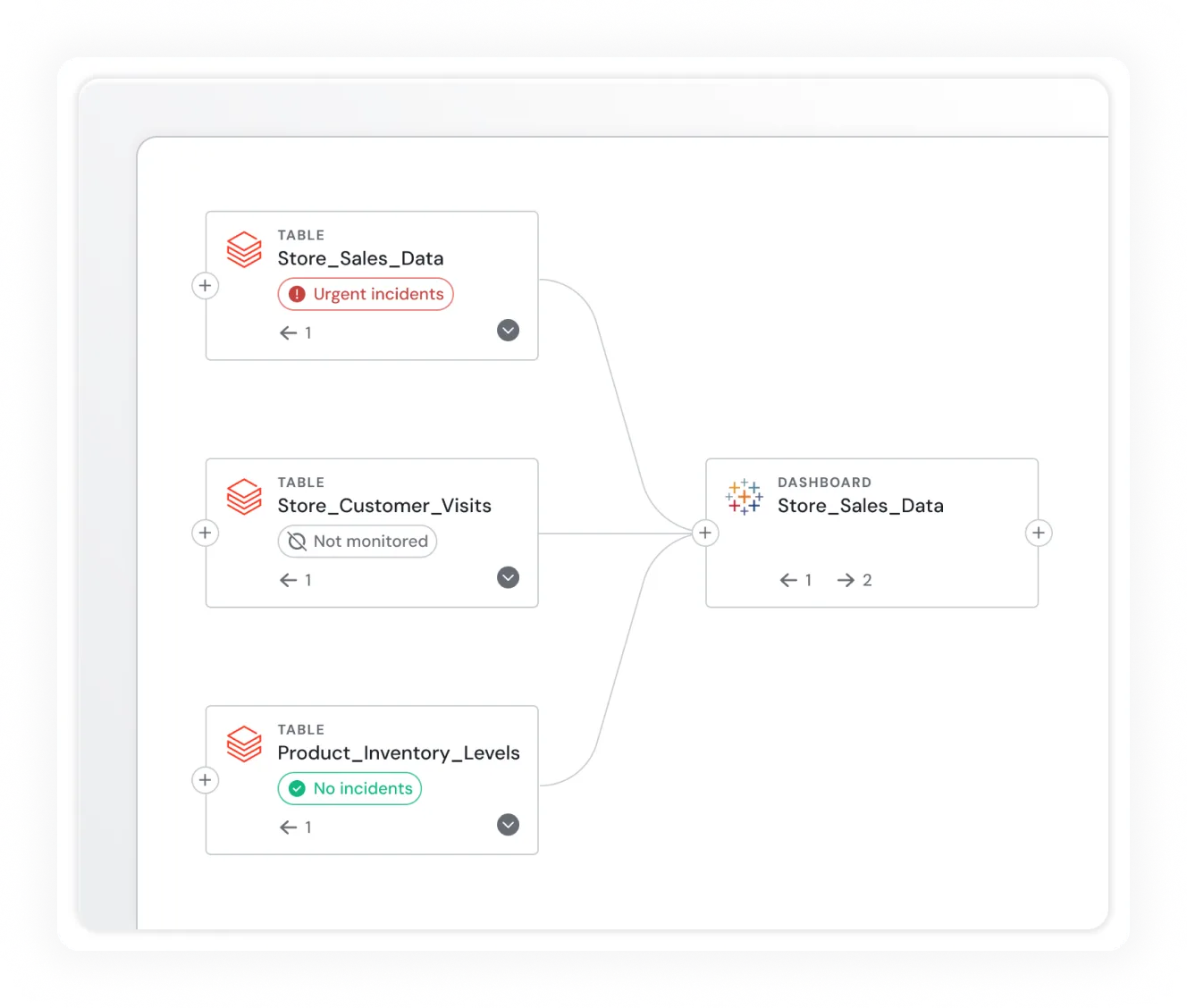
Deep Lineage & Root Cause Analysis
- Column-level lineage across tables, SQL jobs, notebooks, and workflows
- Instantly surface the impact of schema changes or upstream issues
- Native integration with Unity Catalog for a unified metadata view
Operational & Governance Insights
- Query-level telemetry, access logs, job runs, and system metadata
- All fully queryable and visualized in observability dashboards
- Enables governance, cost optimization, and security monitoring
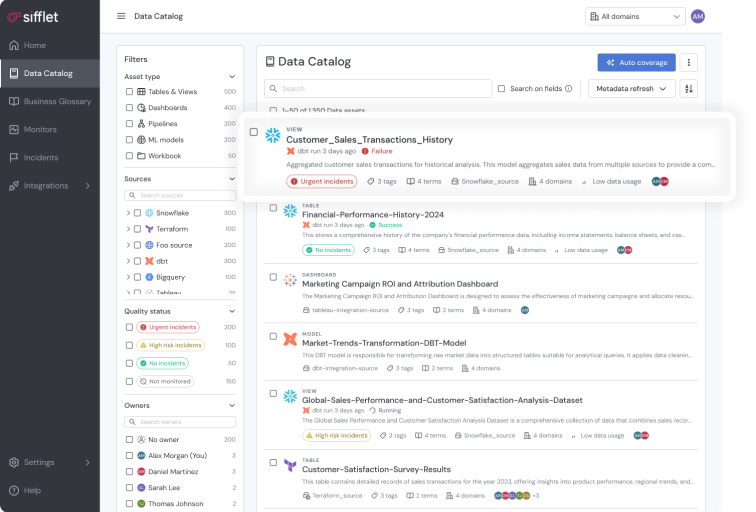

Native Integration with Databricks Ecosystem
- Tight integration with Databricks REST APIs and Unity Catalog
- Observability for Databricks Workflows from orchestration to execution
- Plug-and-play setup, no heavy engineering required
Built for Enterprise-Grade Data Teams
- Certified Databricks Technology Partner
- Deployed in production across global enterprises like St-Gobain and or Euronext
- Designed for scale, governance, and collaboration
“The real value isn’t just in surfacing anomalies. It’s in turning observability into a strategic advantage. Sifflet enables exactly that, on Databricks, at scale.”
Senior Data Leader, North American Enterprise (Anonymous by Choice but happy)
Perfect For…
- Data leaders scaling Databricks across teams
- Analytics teams needing trustworthy dashboards
- Governance teams requiring real lineage and audit trails
- ML teams who need reliable, explainable training data

Frequently asked questions
Want to try Sifflet on your Databricks Stack?
Get in touch now!