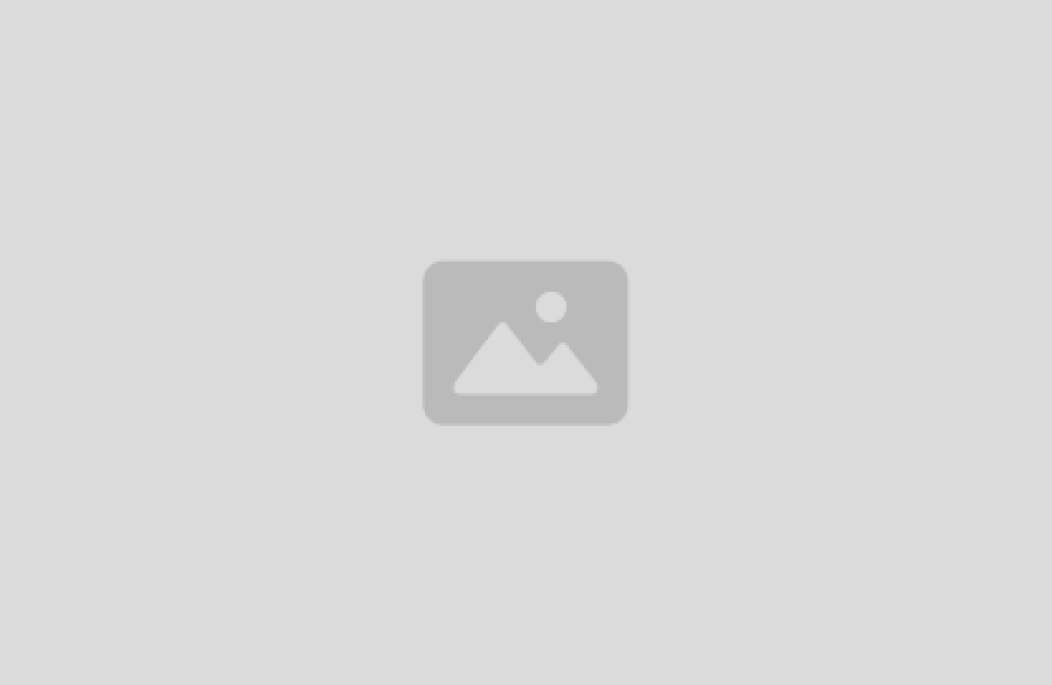
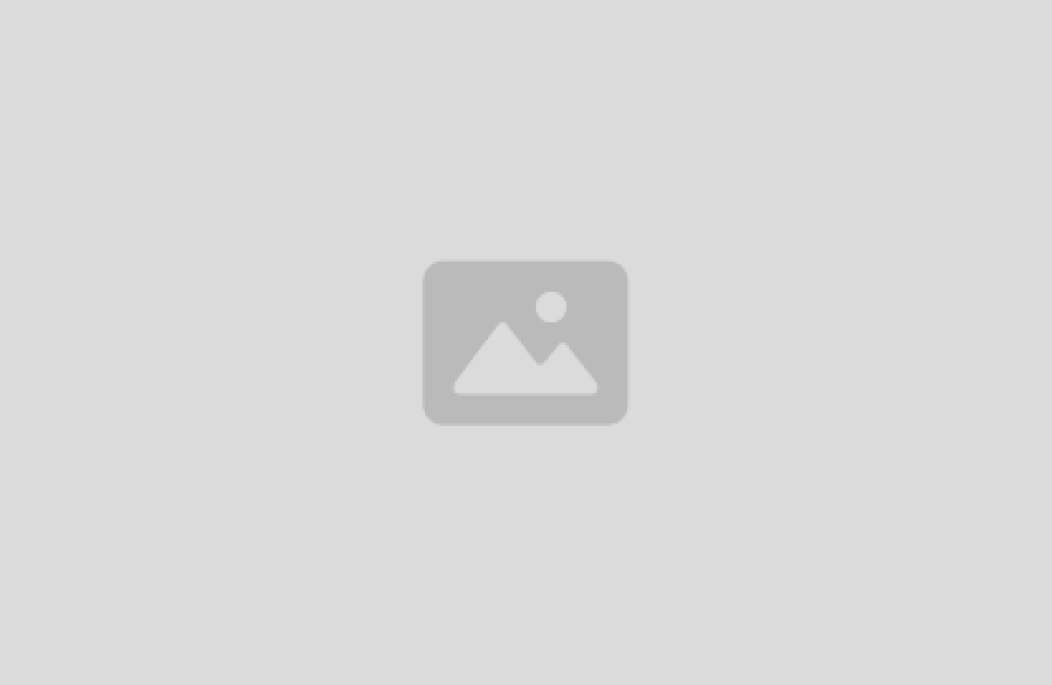
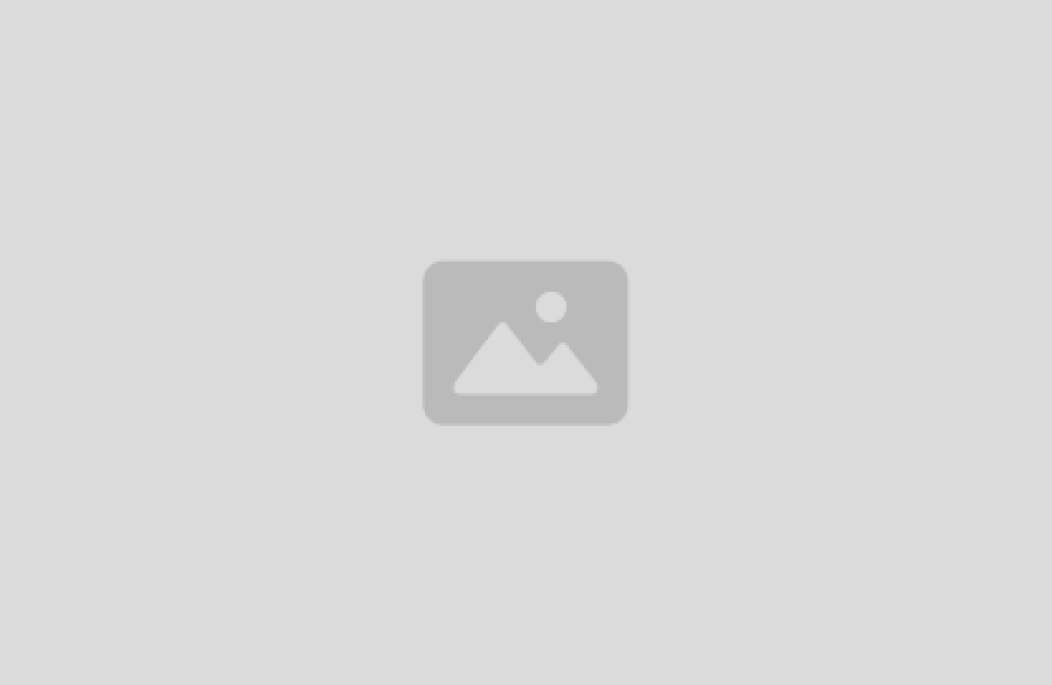
Discover more integrations
No items found.
Get in touch CTA Section
Lorem ipsum dolor sit amet, consectetur adipiscing elit, sed do eiusmod tempor incididunt ut labore et dolore magna aliqua.
Frequently asked questions
How does Sifflet use AI to improve data classification?
Sifflet leverages machine learning to provide AI Suggestions for classification tags, helping teams automatically identify and label key data characteristics like PII or low cardinality. This not only streamlines data management but also enhances data quality monitoring by reducing manual effort and human error.
How does Sifflet support SLA compliance and proactive monitoring?
With real-time metrics and intelligent alerting, Sifflet helps ensure SLA compliance by detecting issues early and offering root cause analysis. Its proactive monitoring features, like dynamic thresholding and auto-remediation suggestions, keep your data pipelines healthy and responsive.
How does Sifflet support root cause analysis when a deviation is detected?
Sifflet combines distribution deviation monitoring with field-level data lineage tracking. This means when an anomaly is detected, you can quickly trace it back to the source and resolve it efficiently. It’s a huge time-saver for teams managing complex data pipeline monitoring.
How did implementing a data observability platform impact Hypebeast’s operations?
After adopting Sifflet’s observability platform, Hypebeast saw a 204% improvement in data quality, a 178% increase in data product delivery, and a 75% boost in ad hoc request speed. These gains translated into faster, more reliable insights and better collaboration across departments.
What role do Common Table Expressions (CTEs) play in query optimization?
CTEs help simplify complex queries by breaking them into manageable parts. This boosts readability and performance, making it easier to identify issues during root cause analysis and enhancing your data quality monitoring efforts.
How can executive sponsorship help scale data governance efforts?
Executive sponsorship is essential for scaling data governance beyond grassroots efforts. As organizations mature, top-down support ensures proper budget allocation for observability tools, data pipeline monitoring, and team resources. When leaders are personally invested, it helps shift the mindset from reactive fixes to proactive data quality and governance practices.
Why is a user-friendly interface important in an observability tool?
A user-friendly interface boosts adoption across teams and makes it easier to navigate complex datasets. For observability tools, especially those focused on data cataloging and data discovery, a clean UI enables faster insights and more efficient collaboration.
How does Sifflet enhance data lineage tracking for dbt projects?
Sifflet enriches your data lineage tracking by visually mapping out your dbt models and how they connect across different projects. This is especially useful for teams managing multiple dbt repositories, as Sifflet brings everything together into a clear, centralized lineage view that supports root cause analysis and proactive monitoring.