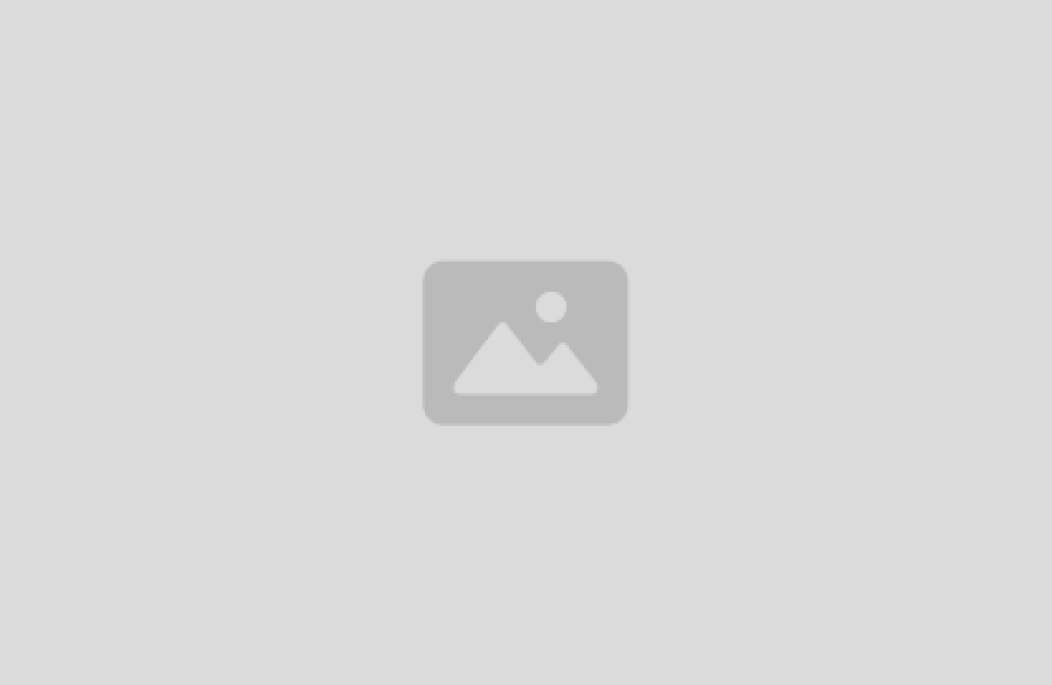
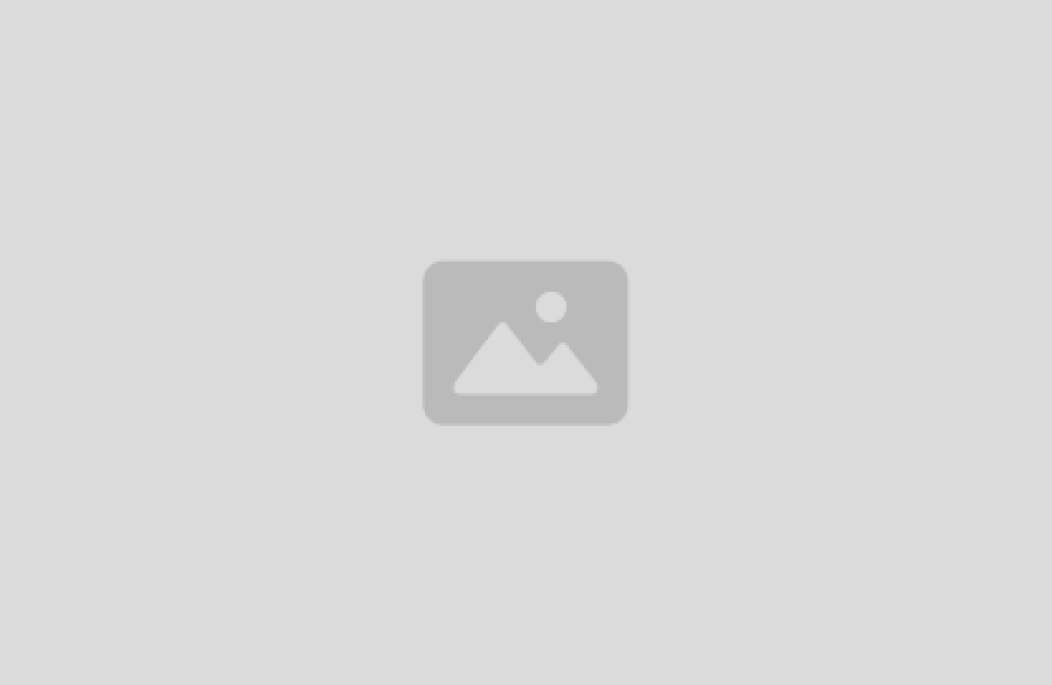
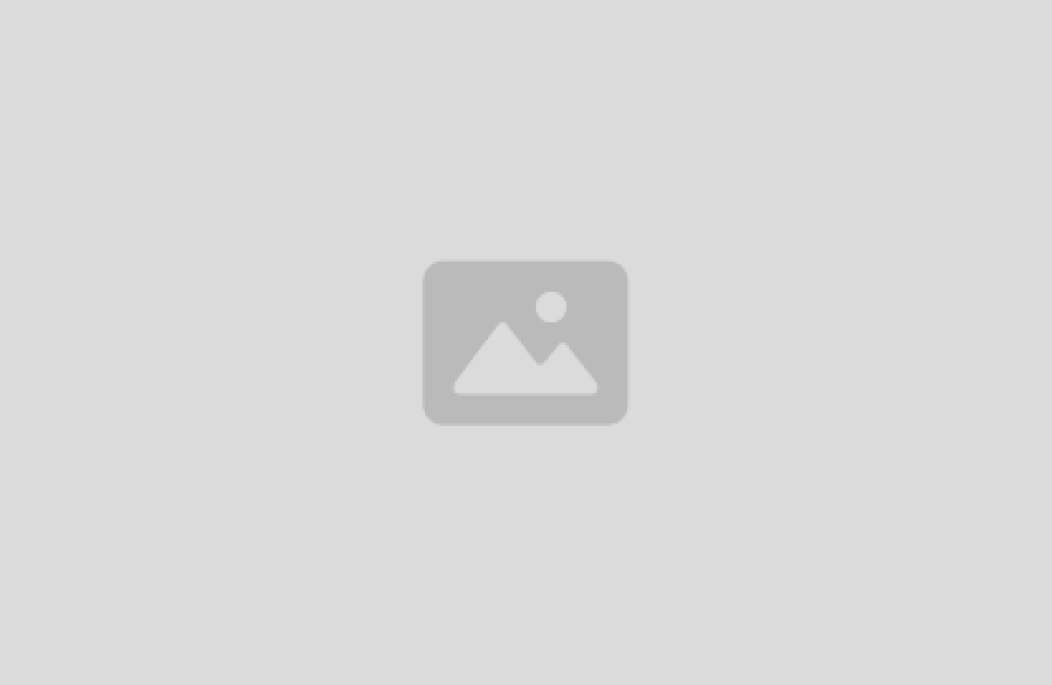
Discover more integrations
No items found.
Get in touch CTA Section
Lorem ipsum dolor sit amet, consectetur adipiscing elit, sed do eiusmod tempor incididunt ut labore et dolore magna aliqua.
Frequently asked questions
What’s the best way to prevent bad data from impacting our business decisions?
Preventing bad data starts with proactive data quality monitoring. That includes data profiling, defining clear KPIs, assigning ownership, and using observability tools that provide real-time metrics and alerts. Integrating data lineage tracking also helps you quickly identify where issues originate in your data pipelines.
Why is data observability becoming essential for modern data teams?
As data pipelines grow more complex, data observability provides the visibility needed to monitor and troubleshoot issues across the full stack. By adopting a robust observability platform, teams can detect anomalies, ensure SLA compliance, and maintain data reliability without relying on manual checks or reactive fixes.
How can integration and connectivity improve data pipeline monitoring?
When a data catalog integrates seamlessly with your databases, cloud storage, and data lakes, it enhances your ability to monitor data pipelines in real time. This connectivity supports better ingestion latency tracking and helps maintain a reliable observability platform.
Why are data consumers becoming more involved in observability decisions?
We’re seeing a big shift where data consumers—like analysts and business users—are finally getting a seat at the table. That’s because data observability impacts everyone, not just engineers. When trust in data is operationalized, it boosts confidence across the business and turns data teams into value creators.
How can data observability support better hiring decisions for data teams?
When you prioritize data observability, you're not just investing in tools, you're building a culture of transparency and accountability. This helps attract top-tier Data Engineers and Analysts who value high-quality pipelines and proactive monitoring. Embedding observability into your workflows also empowers your team with root cause analysis and pipeline health dashboards, helping them work more efficiently and effectively.
How does Sifflet use AI to enhance data observability?
Sifflet uses AI not just for buzzwords, but to genuinely improve your workflows. From AI-powered metadata generation to dynamic thresholding and intelligent anomaly detection, Sifflet helps teams automate data quality monitoring and make faster, smarter decisions based on real-time insights.
Why is data observability important during the data integration process?
Data observability is key during data integration because it helps detect issues like schema changes or broken APIs early on. Without it, bad data can flow downstream, impacting analytics and decision-making. At Sifflet, we believe observability should start at the source to ensure data reliability across the whole pipeline.
What can I expect from Sifflet at Big Data Paris 2024?
We're so excited to welcome you at Booth #D15 on October 15 and 16! You’ll get to experience live demos of our latest data observability features, hear real client stories like Saint-Gobain’s, and explore how Sifflet helps improve data reliability and streamline data pipeline monitoring.