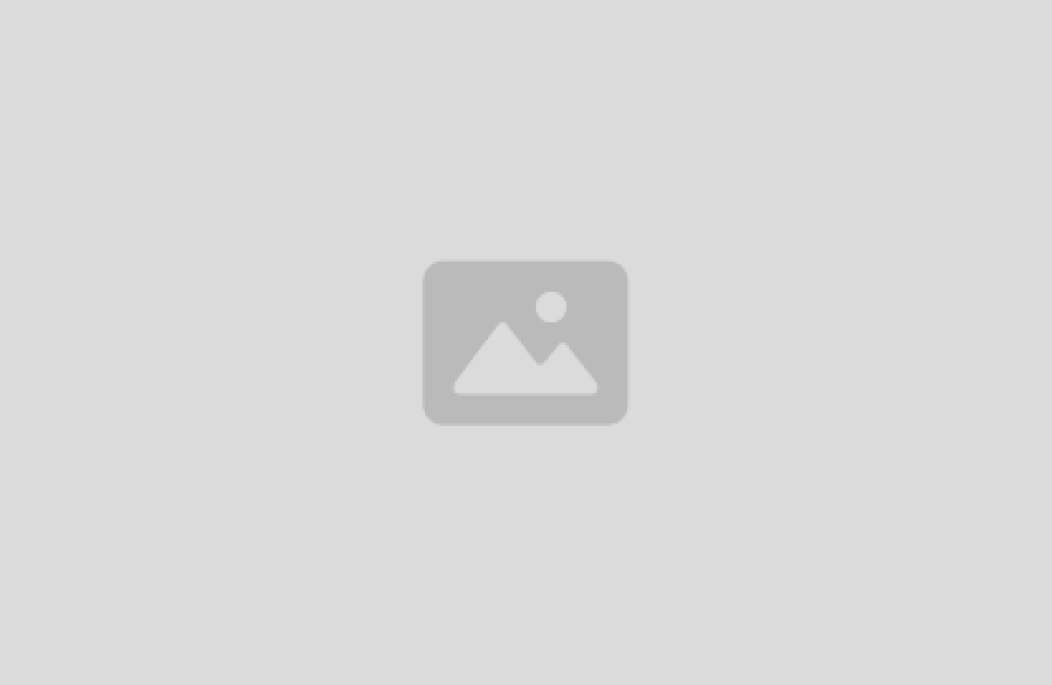
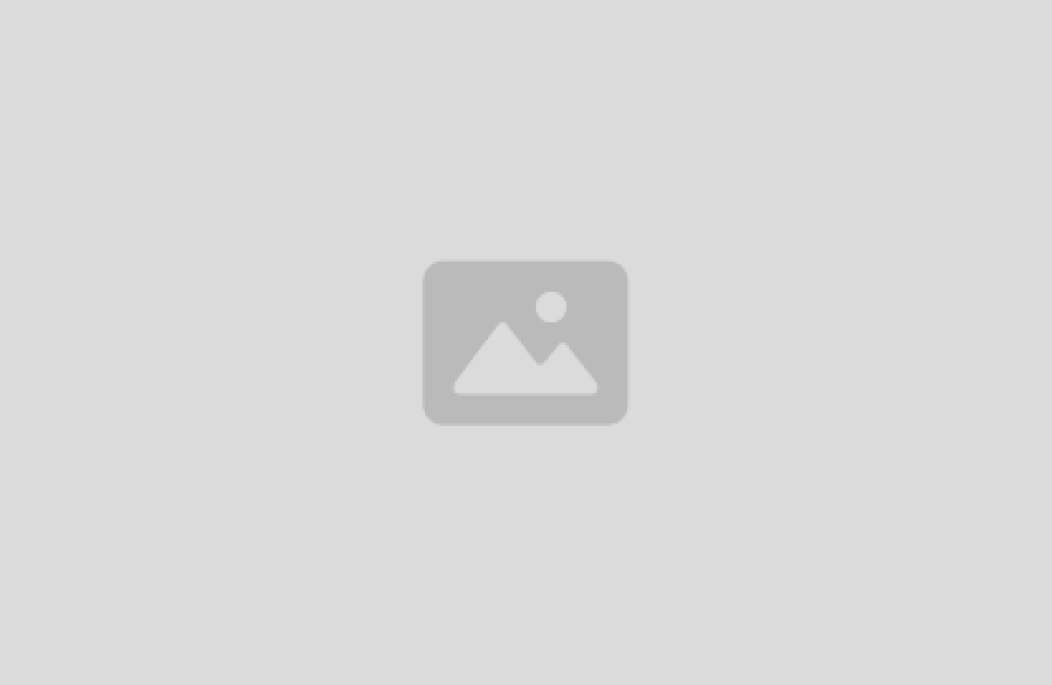
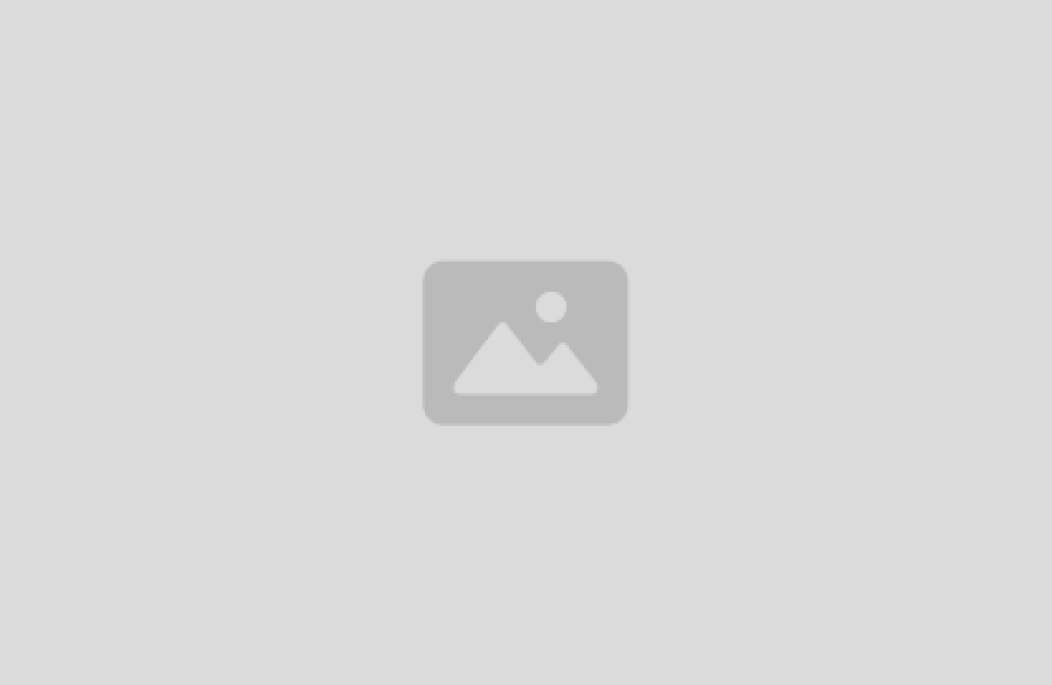
Discover more integrations
No items found.
Get in touch CTA Section
Lorem ipsum dolor sit amet, consectetur adipiscing elit, sed do eiusmod tempor incididunt ut labore et dolore magna aliqua.
Frequently asked questions
How can data observability help reduce data entropy?
Data entropy refers to the chaos and disorder in modern data environments. A strong data observability platform helps reduce this by providing real-time metrics, anomaly detection, and data lineage tracking. This gives teams better visibility across their data pipelines and helps them catch issues early before they impact the business.
Is this feature part of Sifflet’s larger observability platform?
Yes, dbt Impact Analysis is a key addition to Sifflet’s observability platform. It integrates seamlessly into your GitHub or GitLab workflows and complements other features like data lineage tracking and data quality monitoring to provide holistic data observability.
Is Sifflet suitable for non-technical users who want to contribute to data quality?
Yes, and that’s one of the things we’re most excited about! Sifflet empowers non-technical users to define custom monitoring rules and participate in data quality efforts without needing to write dbt code. It’s all part of building a culture of shared responsibility around data governance and observability.
Why are traditional data catalogs no longer enough for modern data teams?
Traditional data catalogs focus mainly on metadata management, but they don't actively assess data quality or track changes in real time. As data environments grow more complex, teams need more than just an inventory. They need data observability tools that provide real-time metrics, anomaly detection, and data quality monitoring to ensure reliable decision-making.
How does Sifflet help with data observability during the CI process?
Sifflet integrates directly with your CI pipelines on platforms like GitHub and GitLab to proactively surface issues before code is merged. By analyzing the impact of dbt model changes and running data quality monitors in testing environments, Sifflet ensures data reliability and minimizes production disruptions.
Why is data observability so important for AI-powered organizations in 2025?
Great question! As AI continues to evolve, the quality and reliability of the data feeding those models becomes even more critical. Data observability ensures that your AI systems are powered by clean, accurate, and up-to-date data. With platforms like Sifflet, organizations can detect issues like data drift, monitor real-time metrics, and maintain data governance, all of which help AI models stay accurate and trustworthy.
What is dbt Impact Analysis and how does it help with data observability?
dbt Impact Analysis is a new feature from Sifflet that automatically comments on GitHub or GitLab pull requests with a list of impacted assets when a dbt model is changed. This helps teams enhance their data observability by understanding downstream effects before changes go live.
How can organizations balance the need for data accuracy with the cost of achieving it?
That's a smart consideration! While 100% accuracy sounds ideal, it's often costly and unrealistic. A better approach is to define acceptable thresholds through data validation rules and data profiling. By using observability platforms that support threshold-based alerts and dynamic thresholding, teams can focus on what matters most without over-investing in perfection.