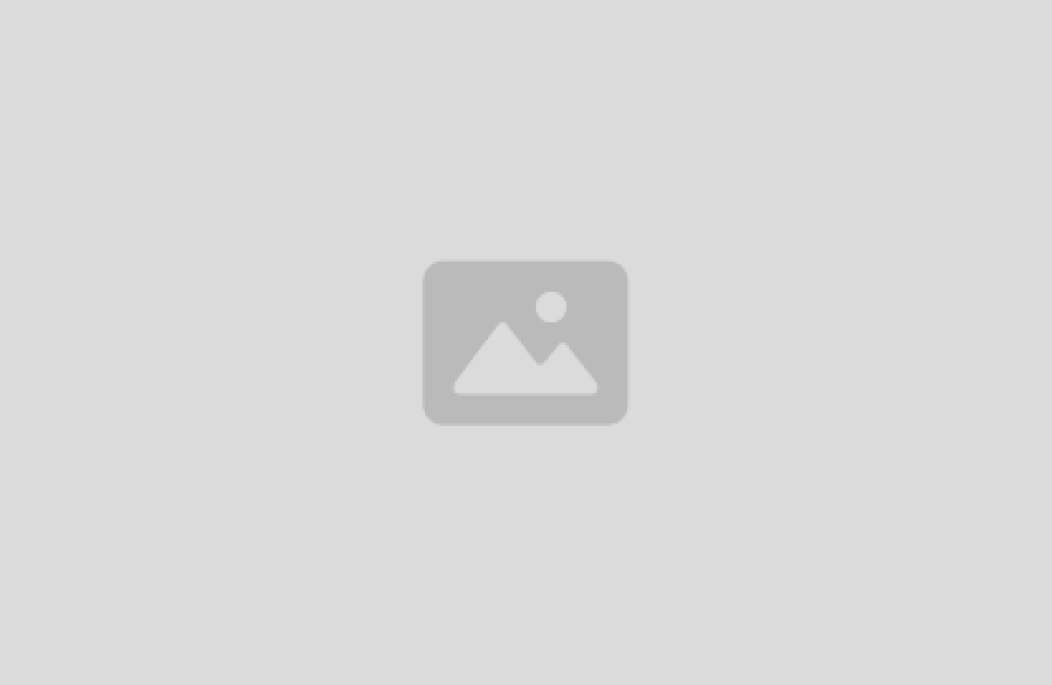
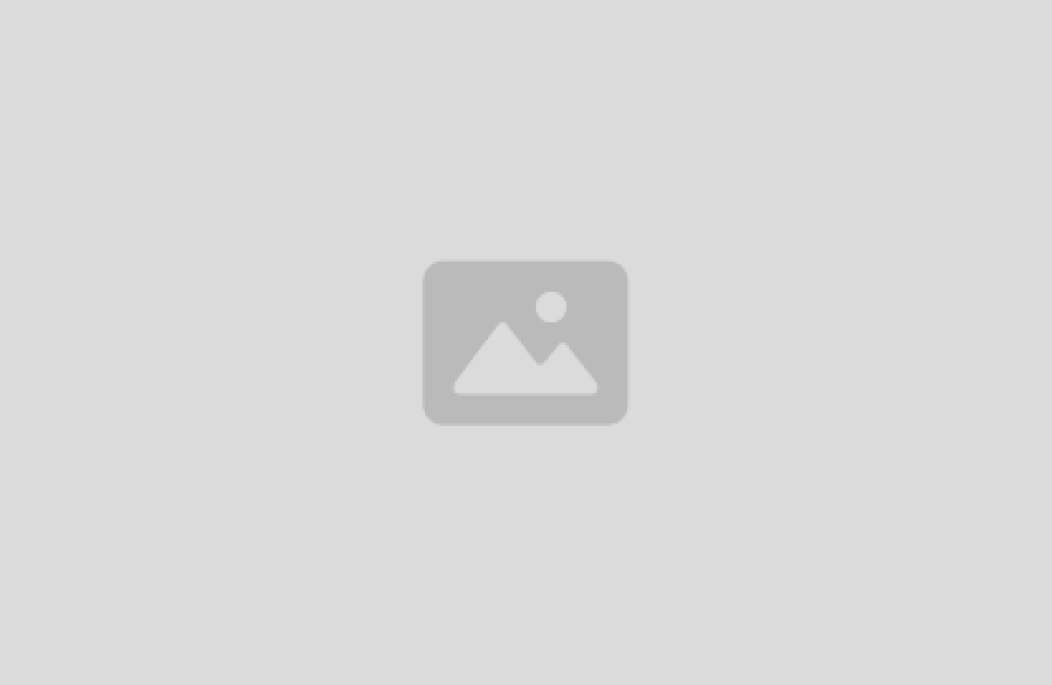
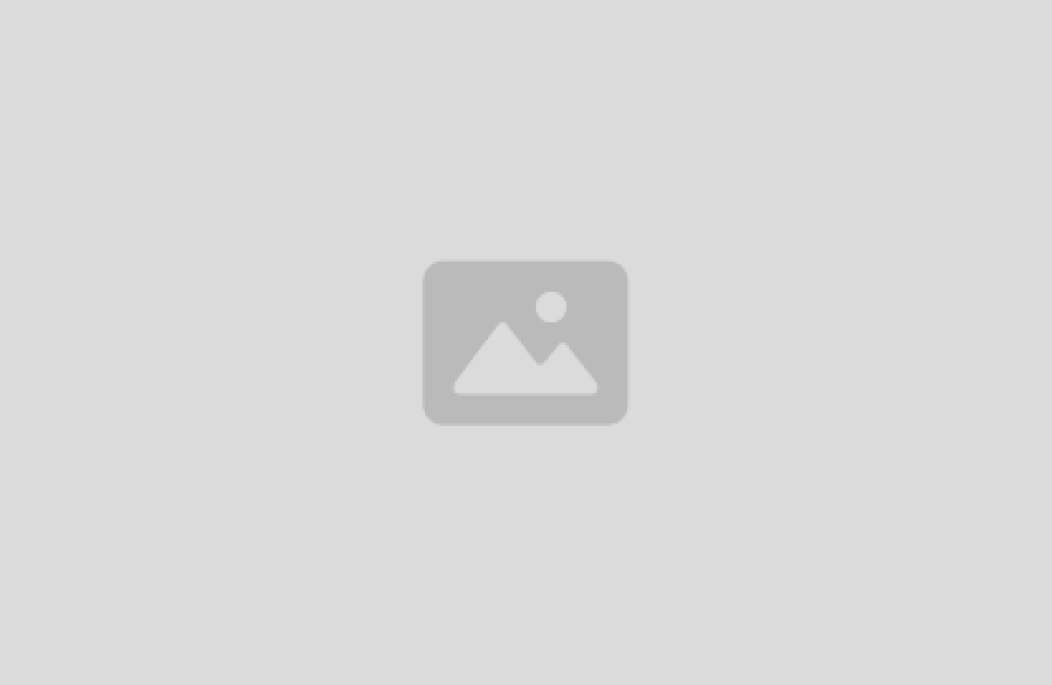
Discover more integrations
No items found.
Get in touch CTA Section
Lorem ipsum dolor sit amet, consectetur adipiscing elit, sed do eiusmod tempor incididunt ut labore et dolore magna aliqua.
Frequently asked questions
Who should use the data observability checklist?
This checklist is for anyone who relies on trustworthy data—from CDOs and analysts to DataOps teams and engineers. Whether you're focused on data governance, anomaly detection, or building resilient pipelines, the checklist gives you a clear path to choosing the right observability tools.
Can the Sifflet AI Assistant help non-technical users with data quality monitoring?
Absolutely! One of our goals is to democratize data observability. The Sifflet AI Assistant is designed to be accessible to both technical and non-technical users, offering natural language interfaces and actionable insights that simplify data quality monitoring across the organization.
What is SQL Table Tracer and how does it help with data observability?
SQL Table Tracer (STT) is a lightweight library that extracts table-level lineage from SQL queries. It plays a key role in data observability by identifying upstream and downstream tables, making it easier to understand data dependencies and track changes across your data pipelines.
How does Sifflet support collaboration across data teams?
Sifflet promotes un-siloed data quality by offering a unified platform where data engineers, analysts, and business users can collaborate. Features like pipeline health dashboards, data lineage tracking, and automated incident reports help teams stay aligned and respond quickly to issues.
How does data observability help ensure SLA compliance for data products?
Data observability plays a big role in SLA compliance by continuously monitoring data freshness, quality, and availability. With tools like Sifflet, teams can set alerts and track metrics that align with their SLAs, ensuring data products meet business expectations consistently.
What are the main challenges of implementing Data as a Product?
Some key challenges include ensuring data privacy and security, maintaining strong data governance, and investing in data optimization. These areas require robust monitoring and compliance tools. Leveraging an observability platform can help address these issues by providing visibility into data lineage, quality, and pipeline performance.
What role does data lineage tracking play in data governance?
Data lineage tracking is essential for understanding where data comes from, how it changes, and where it goes. It supports compliance efforts, improves root cause analysis, and reduces confusion in cross-functional teams. Combined with data governance, lineage tracking ensures transparency in data pipelines and builds trust in analytics and reporting.
What role does data lineage play in incident management and alerting?
Data lineage provides visibility into data dependencies, which helps teams assign, prioritize, and resolve alerts more effectively. In an observability platform like Sifflet, this means faster incident response, better alert correlation, and improved on-call management workflows.