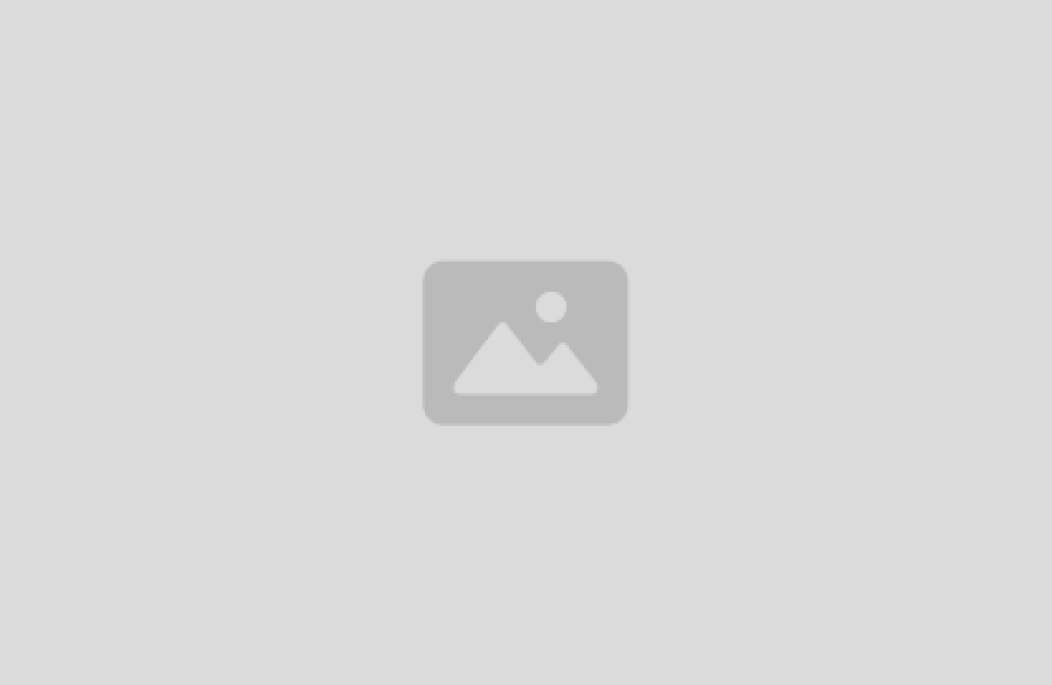
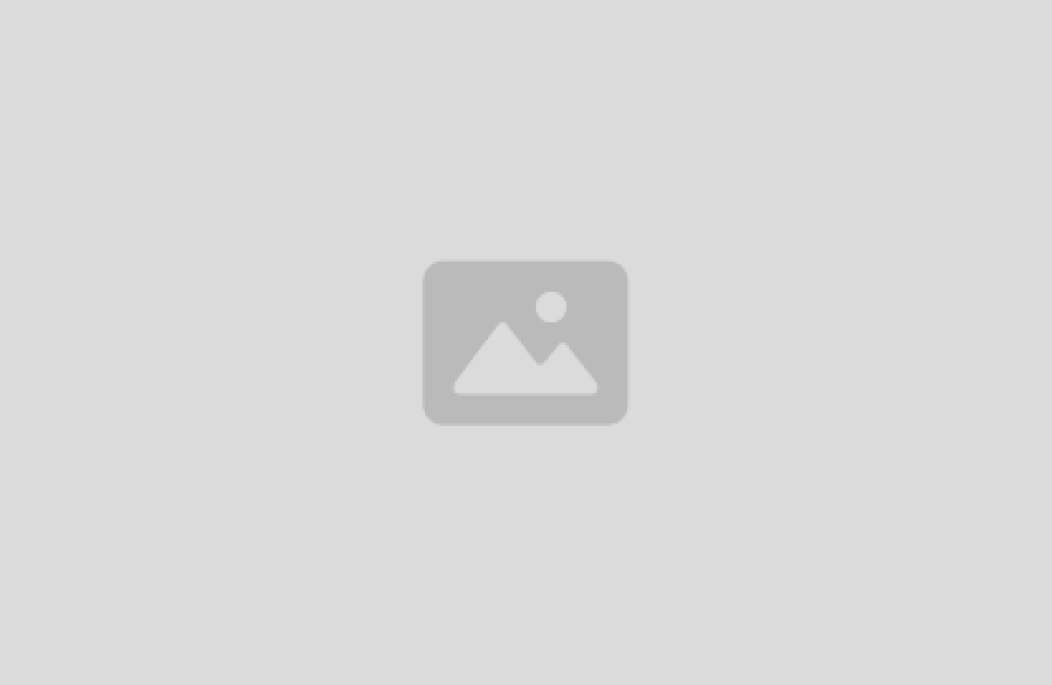
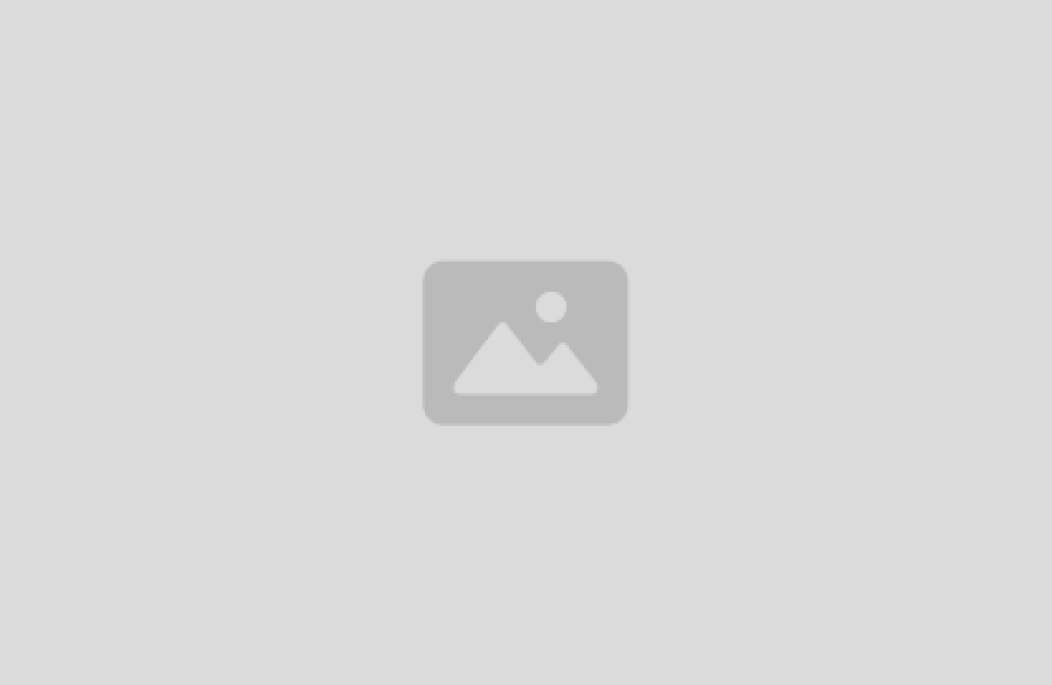
Discover more integrations
No items found.
Get in touch CTA Section
Lorem ipsum dolor sit amet, consectetur adipiscing elit, sed do eiusmod tempor incididunt ut labore et dolore magna aliqua.
Frequently asked questions
How does Sifflet support local development workflows for data teams?
Sifflet is integrating deeply with local development tools like dbt and the Sifflet CLI. Soon, you'll be able to define monitors directly in dbt YAML files and run them locally, enabling real-time metrics checks and anomaly detection before deployment, all from your development environment.
Why are traditional data catalogs no longer enough for modern data teams?
Traditional data catalogs focus mainly on metadata management, but they don't actively assess data quality or track changes in real time. As data environments grow more complex, teams need more than just an inventory. They need data observability tools that provide real-time metrics, anomaly detection, and data quality monitoring to ensure reliable decision-making.
How can data lineage tracking help with root cause analysis?
Data lineage tracking shows how data flows through your systems and how different assets depend on each other. This is incredibly helpful for root cause analysis because it lets you trace issues back to their source quickly. With Sifflet’s lineage capabilities, you can understand both upstream and downstream impacts of a data incident, making it easier to resolve problems and prevent future ones.
What makes Sifflet's data catalog more useful for data discovery?
Sifflet's data catalog is enriched with metadata, schema versions, usage stats, and even health status indicators. This makes it easy for users to search, filter, and understand data assets in context. Plus, it integrates seamlessly with your data sources, so you always have the most up-to-date view of your data ecosystem.
What is data governance and why does it matter for modern businesses?
Data governance is a framework of policies, roles, and processes that ensure data is accurate, secure, and used responsibly across an organization. It brings clarity and accountability to data management, helping teams trust the data they use, stay compliant with regulations, and make confident decisions. When paired with data observability tools, governance ensures data remains reliable and actionable at scale.
Why is data quality so critical for businesses today?
Great question! Data quality is essential because it directly influences decision-making, customer satisfaction, and operational efficiency. Poor data quality can lead to faulty insights, wasted resources, and even reputational damage. That's why many teams are turning to data observability platforms to ensure their data is accurate, complete, and trustworthy across the entire pipeline.
How does Sifflet support SLA compliance and proactive monitoring?
With real-time metrics and intelligent alerting, Sifflet helps ensure SLA compliance by detecting issues early and offering root cause analysis. Its proactive monitoring features, like dynamic thresholding and auto-remediation suggestions, keep your data pipelines healthy and responsive.
What role does data governance play in a data observability platform?
Data governance is a core component of any robust data observability solution. Look for platforms that offer features like audit logging, access controls, and encryption. These capabilities help ensure your organization stays compliant with regulations like GDPR, while also protecting sensitive data and maintaining transparency across teams.