Sifflet x DBT - Better Together
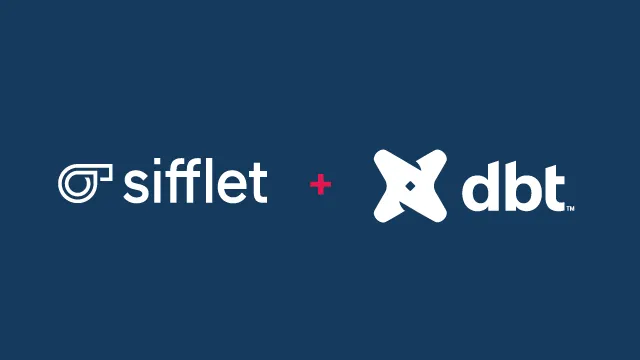
dbt’s Role in Modern Data Transformation
dbt has claimed its spot as a transformative force in data analytics, with a community and tools that have reshaped how data teams manage their workflows. It provides a robust framework that allows analysts to transform, test, and document their data with precision. Despite dbt's strengths in data transformation, visibility into how this data flows and behaves in production is critical. The combination of dbt with Sifflet's observability features addresses this gap, providing clarity and control over your data pipelines.
Your dbt documentation, straight to the Sifflet Catalog
Leveraging dbt's Documentation
Integrating dbt with the Sifflet catalog means your dbt documentation doesn't just sit idle; it becomes an active part of a larger data governance framework. Sifflet automatically pulls in dbt’s detailed metadata, enhancing catalog entries with rich annotations and tags. This creates a centralized knowledge base that enriches the documentation efforts already in place within dbt, making critical information more accessible and useful.
.webp)
.webp)
Integrating dbt Models into Sifflet's Lineage
Visualizing the interconnectedness of data models is essential for understanding their impact. By adding dbt models to the Sifflet lineage, your team gains a clear visual map of data workflows. This is especially powerful for teams using multiple dbt projects, as Sifflet brings coherence to potentially siloed project views, offering a unified lineage that showcases the entirety of your data ecosystem.
Extracting Maximum Value from Your dbt Tests
Shine a Spotlight on Your Data Quality
Tests in dbt are essential for ensuring data quality, but their effectiveness is multiplied when they're not siloed within the confines of development environments. By centralizing dbt tests in a platform accessible company-wide, the entire business can benefit from transparent quality assurance processes. Sifflet elevates this visibility, seamlessly integrating test results into your ecosystem, ensuring that stakeholders at every level are informed and engaged.
Sifflet's catalog enriches these benefits by allowing teams to classify and document dbt tests themselves, using features like tags, business terms, and rich descriptions.
.webp)
Immediate Alerts Where You Work and Collaborate
A robust dbt test suite isn’t enough. When tests fail, immediate action is vital to prevent poor data quality from affecting decision-making. Sifflet excels by delivering real-time alerts directly into the communication tools teams use daily, such as Slack, Microsoft Teams, and email, ensuring swift resolution of any issues detected by dbt tests.
.webp)
Trace the Impact with Sifflet’s Full Lineage and In-context Insights
Understanding the ramifications of a failed dbt test can be complex. Sifflet’s comprehensive data lineage functionality traces those effects, showing exactly which dashboards and reports could be impacted. With the innovative Sifflet Insights Chrome plugin, Sifflet doesn't just flag an issue; it illuminates the potential impact directly on Looker, Tableau, or PowerBI dashboards using the data in question, bringing insights in-context and in real-time.
.webp)
Augmenting Your dbt Test Coverage with Sifflet
Beyond Basic Tests: Monitor Data Drifts and Anomalies
While dbt tests are powerful, they're just the beginning. Sifflet’s suite of over 50 monitoring templates allows you to extend beyond the edge of dbt's capabilities. Monitor unexpected drifts in data behavior without the hassle of setting manual thresholds. Is there an uncharacteristic drop in row counts on Monday morning? Sifflet’s intelligent monitoring catches it, no manual alarm setting required.
.webp)
Unveiling the Hidden Anomalies
Data often hides its secrets in the fine details. A global view may miss the mark, especially if an anomaly is localized, like the data from a single store behaving oddly. Sifflet’s sensitive anomaly detection identifies issues within data segments, considering the unique seasonality of each. For stores not open on Sunday, no data is business as usual—but what if your Sunday-operating store is suddenly silent?
.webp)
.webp)
Democratizing Test Creation for Broader Teams
Not everyone is a dbt model wizard. Sifflet empowers less technical users to contribute to data quality by allowing them to define their own monitoring rules and tests without deep-diving into dbt's inner workings. This inclusive approach extends the responsibility and capability for data quality across the entire organization, fostering a culture where everyone plays a part in data’s truth and reliability.
What's Coming For DBT + Sifflet?
As the collaboration between dbt and Sifflet strengthens, here are two exciting developments to look out for:
In-DBT Monitor Declarations
Soon, you'll be able to declare Sifflet monitors directly in dbt, streamlining your workflow and reinforcing data quality from within your dbt models.
DBT Model Performance Alerts
Ensure your dbt models run efficiently and get alerted when a model's execution time deviates from the norm.