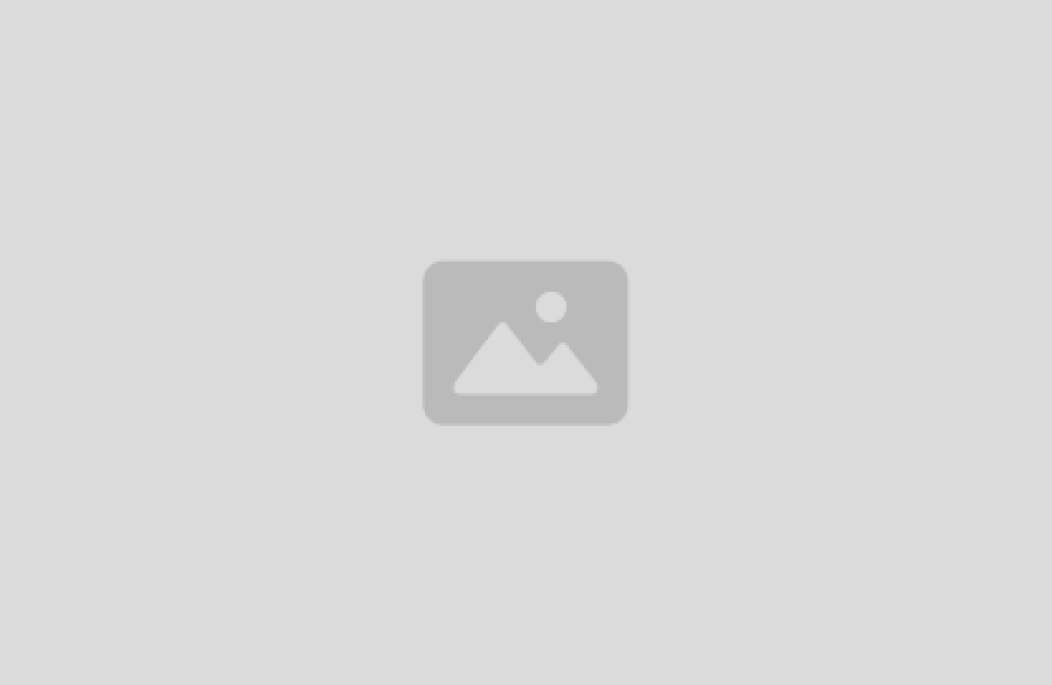
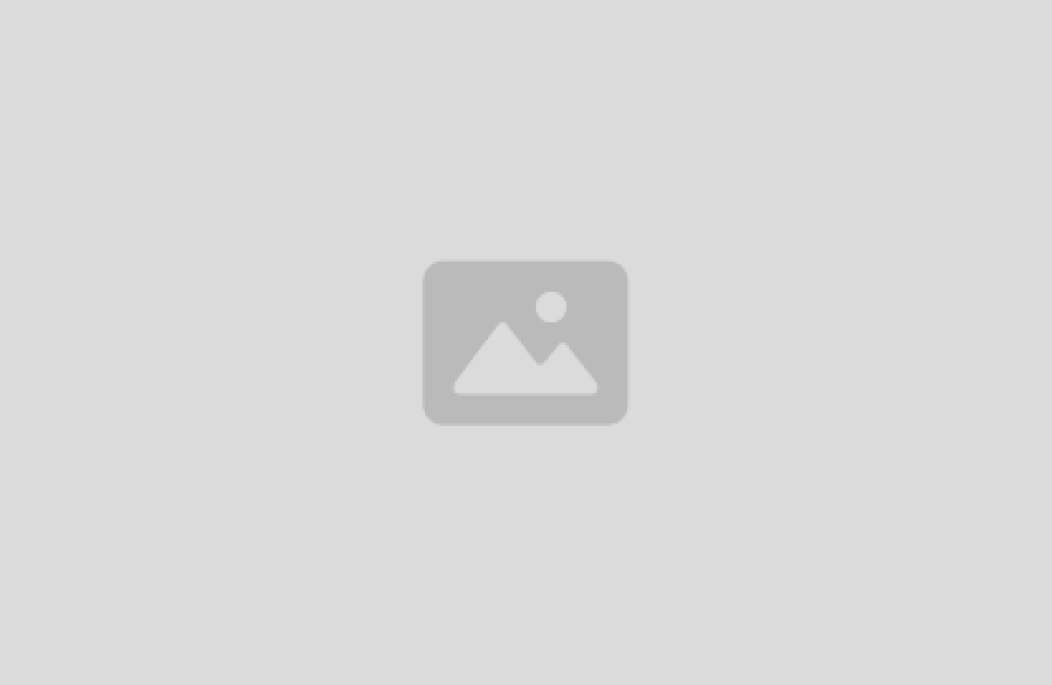
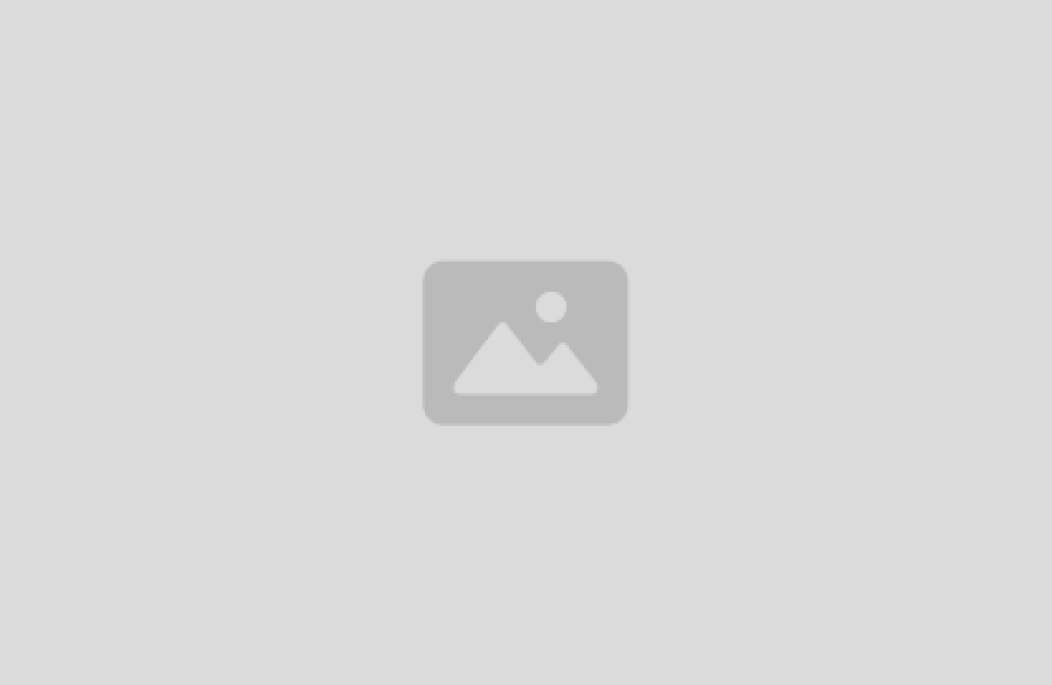
Discover more integrations
No items found.
Get in touch CTA Section
Lorem ipsum dolor sit amet, consectetur adipiscing elit, sed do eiusmod tempor incididunt ut labore et dolore magna aliqua.
Frequently asked questions
What makes a data observability platform truly end-to-end?
Great question! A true data observability platform doesn’t stop at just detecting issues. It guides you through the full lifecycle: monitoring, alerting, triaging, investigating, and resolving. That means it should handle everything from data quality monitoring and anomaly detection to root cause analysis and impact-aware alerting. The best platforms even help prevent issues before they happen by integrating with your data pipeline monitoring tools and surfacing business context alongside technical metrics.
How does Sifflet support reverse ETL and operational analytics?
Sifflet enhances reverse ETL workflows by providing data observability dashboards and real-time monitoring. Our platform ensures your data stays fresh, accurate, and actionable by enabling root cause analysis, data lineage tracking, and proactive anomaly detection across your entire pipeline.
What is the Universal Connector and how does it support data pipeline monitoring?
The Universal Connector lets you integrate Sifflet with any tool in your stack using YAML and API endpoints. It enables full-stack data pipeline monitoring and data lineage tracking, even for tools Sifflet doesn’t natively support, offering a more complete view of your observability workflows.
How does Sifflet help improve data reliability for modern organizations?
At Sifflet, we provide a full-stack observability platform that gives teams complete visibility into their data pipelines. From data quality monitoring to root cause analysis and real-time anomaly detection, we help organizations ensure their data is accurate, timely, and trustworthy.
How does Etam ensure pipeline health while scaling its data operations?
Etam uses observability tools like Sifflet to maintain a healthy data pipeline. By continuously monitoring real-time metrics and setting up proactive alerts, they can catch issues early and ensure their data remains trustworthy as they scale operations.
How does MCP improve root cause analysis in modern data systems?
MCP empowers LLMs to use structured inputs like logs and pipeline metadata, making it easier to trace issues across multiple steps. This structured interaction helps streamline root cause analysis, especially in complex environments where traditional observability tools might fall short. At Sifflet, we’re integrating MCP to enhance how our platform surfaces and explains data incidents.
Can I use Sifflet to detect bad-quality data in my Airflow pipelines?
Absolutely! With Sifflet’s data quality monitoring integrated into Airflow DAGs, you can detect and isolate bad-quality data before it impacts downstream processes. This helps maintain high data reliability and supports SLA compliance.
What exactly is data observability, and how is it different from traditional data monitoring?
Great question! Data observability goes beyond traditional data monitoring by not only detecting when something breaks in your data pipelines, but also understanding why it matters. While monitoring might tell you a pipeline failed, data observability connects that failure to business impact—like whether your CFO’s dashboard is now showing outdated numbers. It's about trust, context, and actionability.