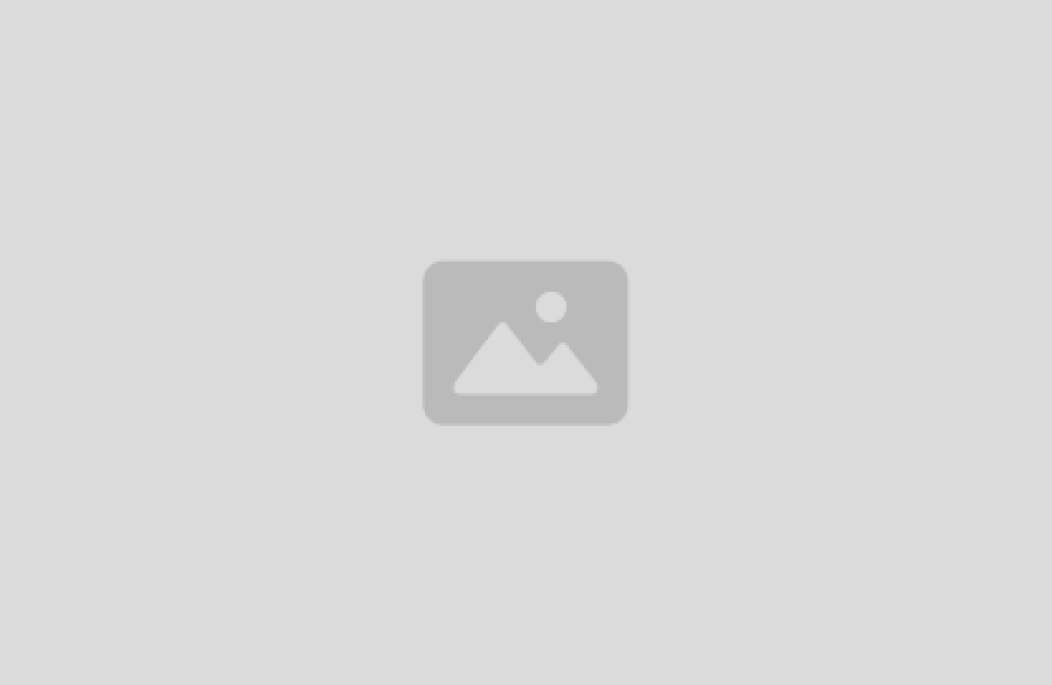
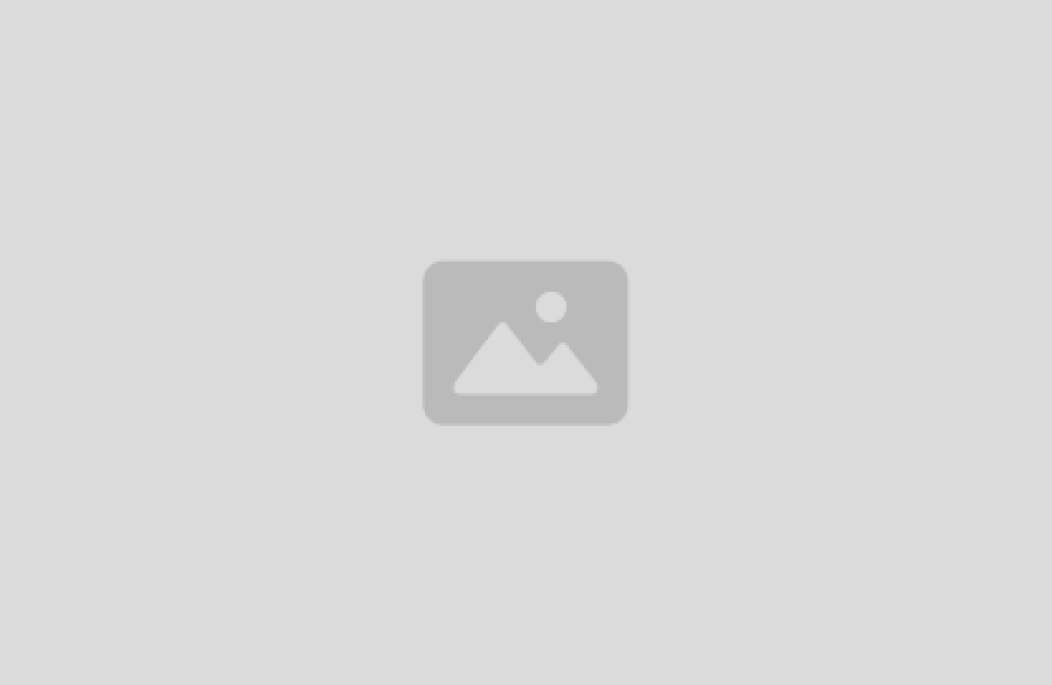
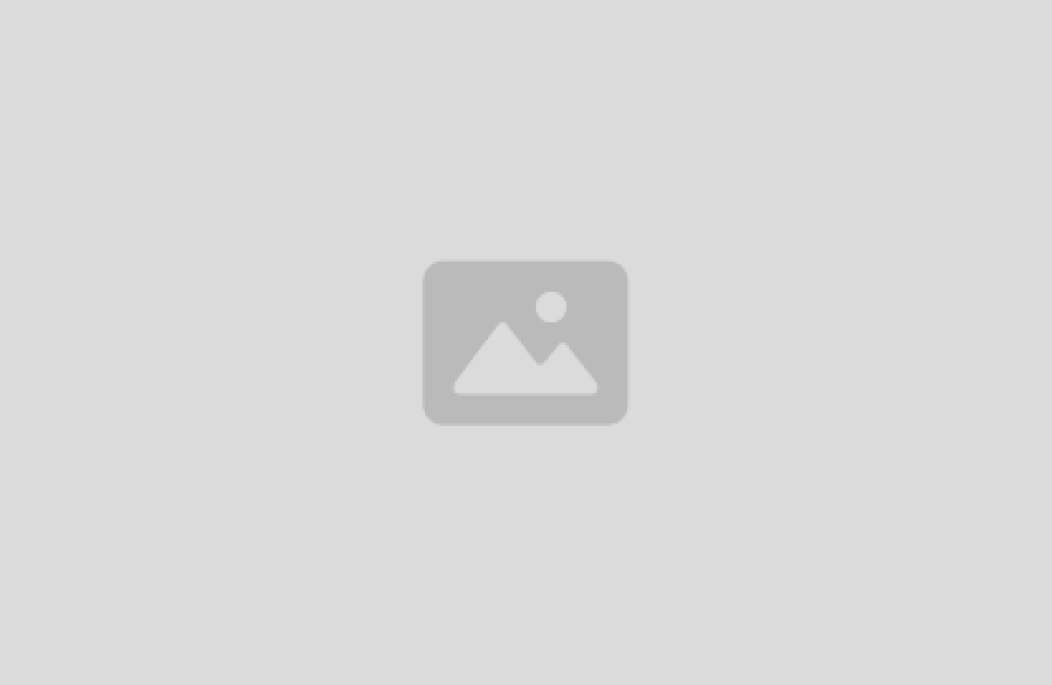
Discover more integrations
No items found.
Get in touch CTA Section
Lorem ipsum dolor sit amet, consectetur adipiscing elit, sed do eiusmod tempor incididunt ut labore et dolore magna aliqua.
Frequently asked questions
Why are traditional data catalogs no longer enough for modern data teams?
Traditional data catalogs focus mainly on metadata management, but they don't actively assess data quality or track changes in real time. As data environments grow more complex, teams need more than just an inventory. They need data observability tools that provide real-time metrics, anomaly detection, and data quality monitoring to ensure reliable decision-making.
What is the difference between data monitoring and data observability?
Great question! Data monitoring is like your car's dashboard—it alerts you when something goes wrong, like a failed pipeline or a missing dataset. Data observability, on the other hand, is like being the driver. It gives you a full understanding of how your data behaves, where it comes from, and how issues impact downstream systems. At Sifflet, we believe in going beyond alerts to deliver true data observability across your entire stack.
What role does data quality monitoring play in a data catalog?
Data quality monitoring ensures your data is accurate, complete, and consistent. A good data catalog should include profiling and validation tools that help teams assess data quality, which is crucial for maintaining SLA compliance and enabling proactive monitoring.
Why is data observability becoming more important than just monitoring?
As data systems grow more complex with cloud infrastructure and distributed pipelines, simple monitoring isn't enough. Data observability platforms like Sifflet go further by offering data lineage tracking, anomaly detection, and root cause analysis. This helps teams not just detect issues, but truly understand and resolve them faster—saving time and avoiding costly outages.
How does Sifflet enhance data lineage tracking for dbt projects?
Sifflet enriches your data lineage tracking by visually mapping out your dbt models and how they connect across different projects. This is especially useful for teams managing multiple dbt repositories, as Sifflet brings everything together into a clear, centralized lineage view that supports root cause analysis and proactive monitoring.
How does Sifflet ensure a user-friendly experience for data teams?
We prioritize user research and apply UX principles like Jacob’s Law to design familiar and intuitive workflows. This helps reduce friction for users working with tools like our Sifflet Insights plugin, which brings real-time metrics and data quality monitoring directly into BI dashboards like Looker and Tableau.
Will there be live demonstrations of Sifflet’s observability platform?
Absolutely! Our team will be offering hands-on demos that showcase how our observability tools integrate into your workflows. From real-time metrics to data quality monitoring, you’ll get a full picture of how Sifflet boosts data reliability across your stack.
What features should we look for in a data observability tool?
A great data observability tool should offer automated data quality checks like data freshness checks and schema change detection, field-level data lineage tracking for root cause analysis, and a powerful metadata search engine. These capabilities streamline incident response and help maintain data governance across your entire stack.