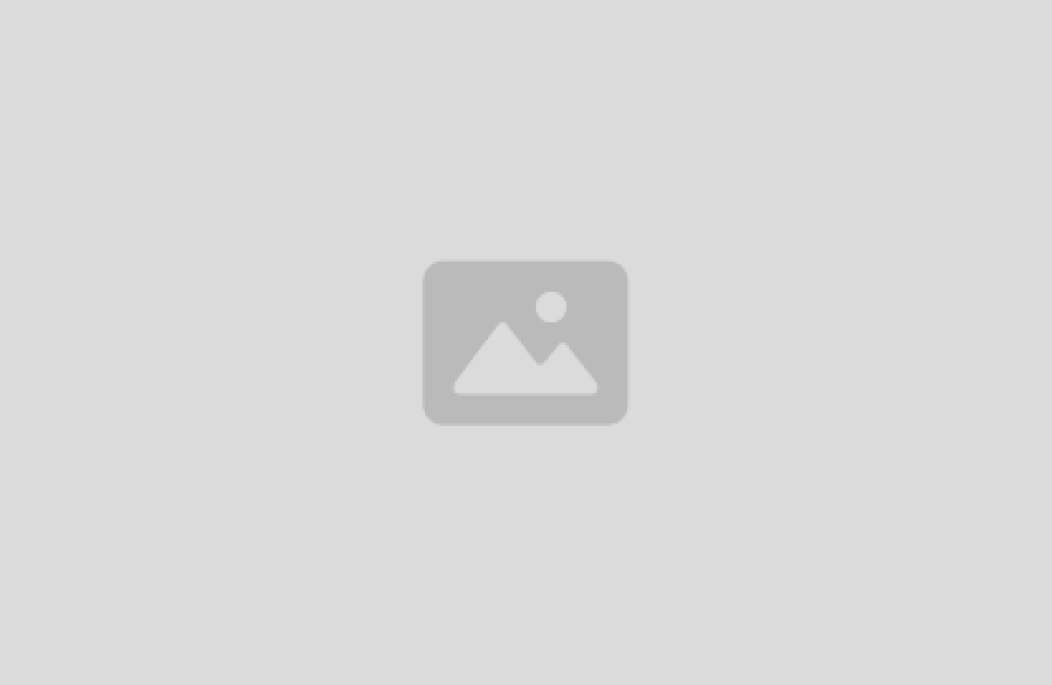
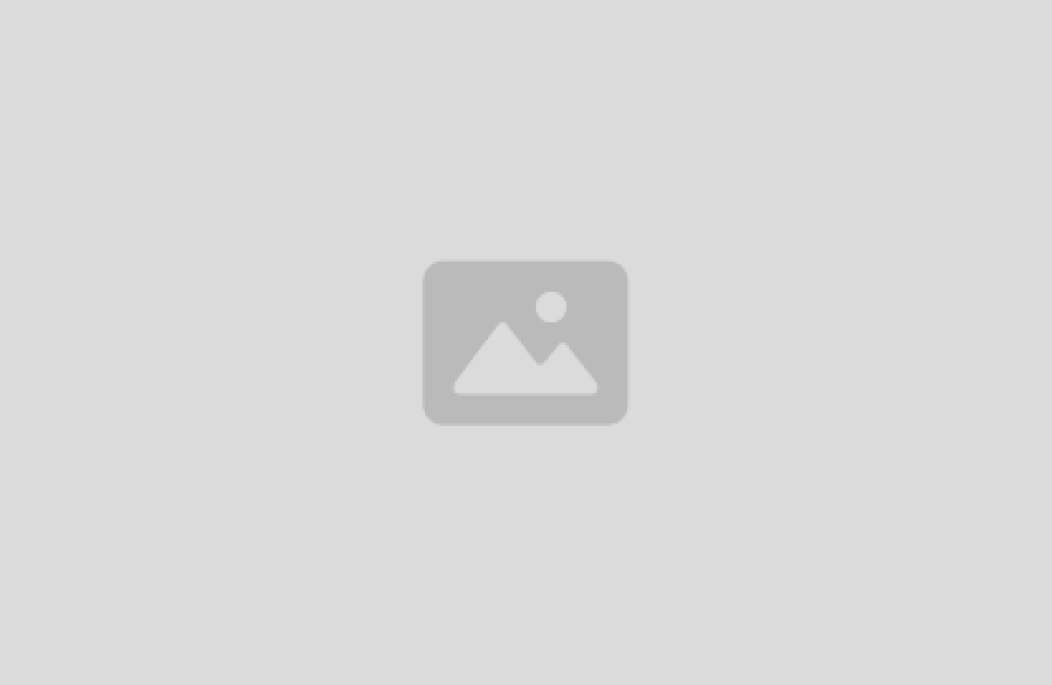
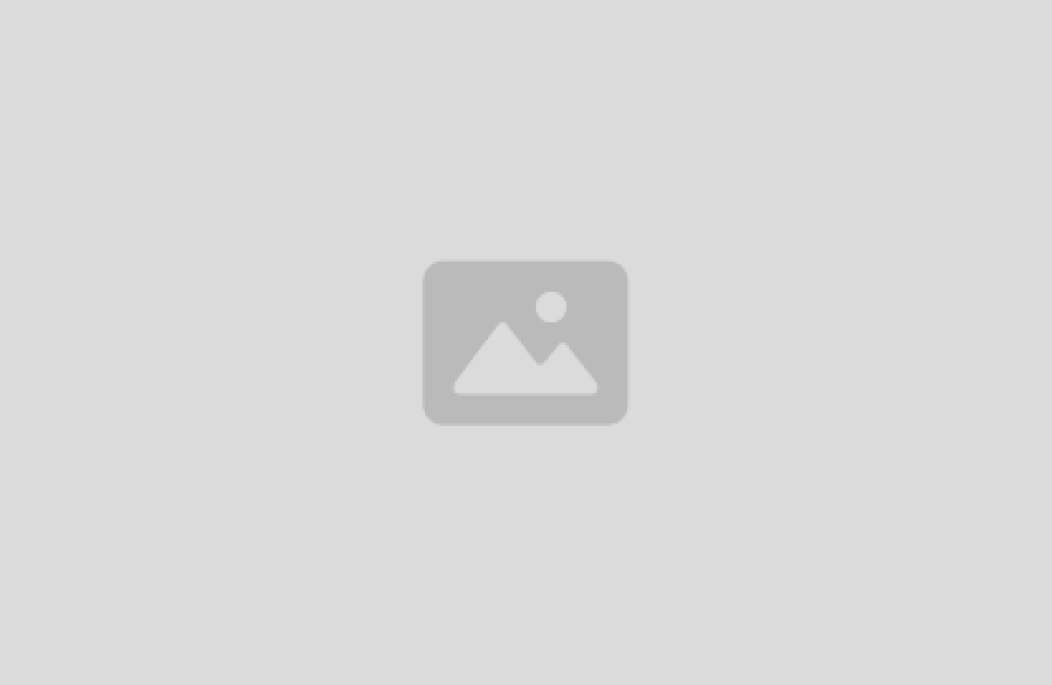
Discover more integrations
No items found.
Get in touch CTA Section
Lorem ipsum dolor sit amet, consectetur adipiscing elit, sed do eiusmod tempor incididunt ut labore et dolore magna aliqua.
Frequently asked questions
What features should we look for in scalable data observability tools?
When evaluating observability tools, scalability is key. Look for features like real-time metrics, automated anomaly detection, incident response automation, and support for both batch data observability and streaming data monitoring. These capabilities help teams stay efficient as data volumes grow.
Why should data teams care about data lineage tracking?
Data lineage tracking is a game-changer for data teams. It helps you understand how data flows through your systems and what downstream processes depend on it. When something breaks, lineage reveals the blast radius—so instead of just knowing a table is late, you’ll know it affects marketing campaigns or executive reports. It’s a critical part of any observability platform that wants to move from reactive to proactive.
Is Sifflet suitable for non-technical users who want to contribute to data quality?
Yes, and that’s one of the things we’re most excited about! Sifflet empowers non-technical users to define custom monitoring rules and participate in data quality efforts without needing to write dbt code. It’s all part of building a culture of shared responsibility around data governance and observability.
What is SQL Table Tracer and how does it help with data lineage tracking?
SQL Table Tracer (STT) is a lightweight library that automatically extracts table-level lineage from SQL queries. It identifies both destination and upstream tables, making it easier to understand data dependencies and build reliable data lineage workflows. This is a key component of any effective data observability strategy.
How does the improved test connection process for Snowflake observability help teams?
The revamped 'Test Connection' process for Snowflake observability now provides detailed feedback on missing permissions or policy issues. This makes setup and troubleshooting much easier, especially during onboarding. It helps ensure smooth data pipeline monitoring and reduces the risk of refresh failures down the line.
Why is a centralized Data Catalog important for data reliability and SLA compliance?
A centralized Data Catalog like Sifflet’s plays a key role in ensuring data reliability and SLA compliance by offering visibility into asset health, surfacing incident alerts, and providing real-time metrics. This empowers teams to monitor data pipelines proactively and meet service level expectations more consistently.
How does MCP improve root cause analysis in modern data systems?
MCP empowers LLMs to use structured inputs like logs and pipeline metadata, making it easier to trace issues across multiple steps. This structured interaction helps streamline root cause analysis, especially in complex environments where traditional observability tools might fall short. At Sifflet, we’re integrating MCP to enhance how our platform surfaces and explains data incidents.
What benefits does end-to-end data lineage offer my team?
End-to-end data lineage helps your team perform accurate impact assessments and faster root cause analysis. By connecting declared and built-in assets, you get full visibility into upstream and downstream dependencies, which is key for data reliability and operational intelligence.