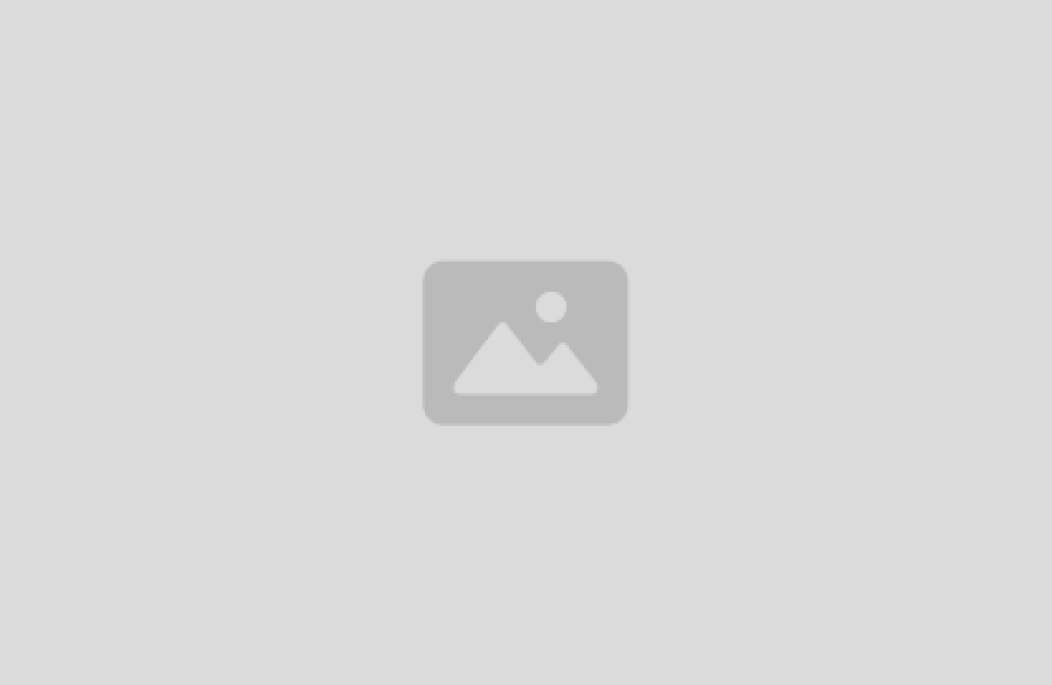
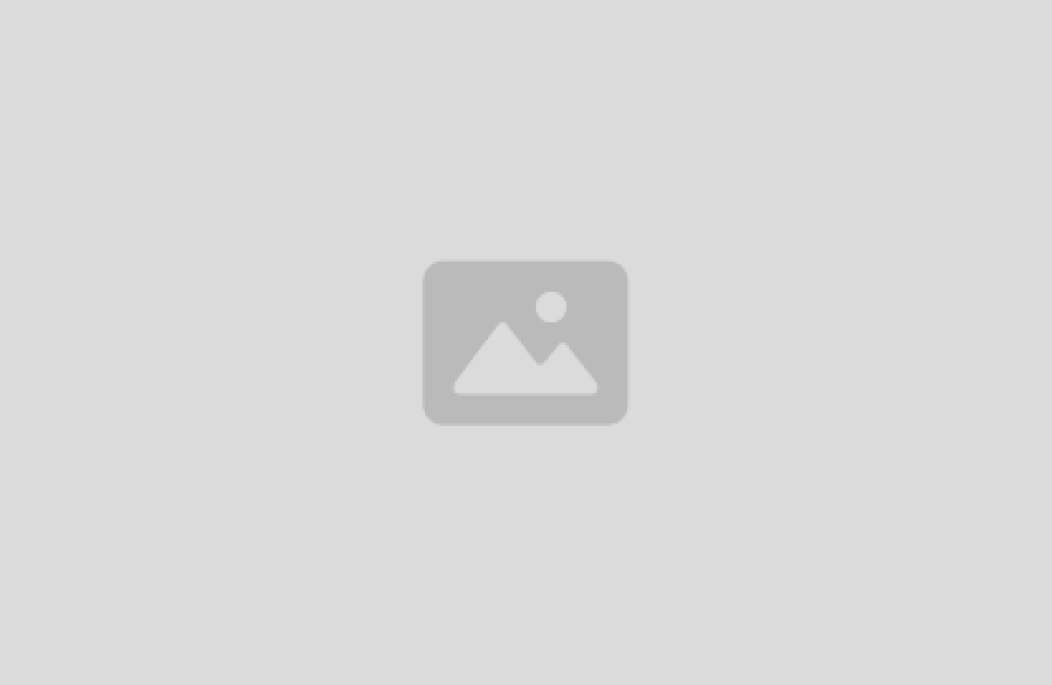
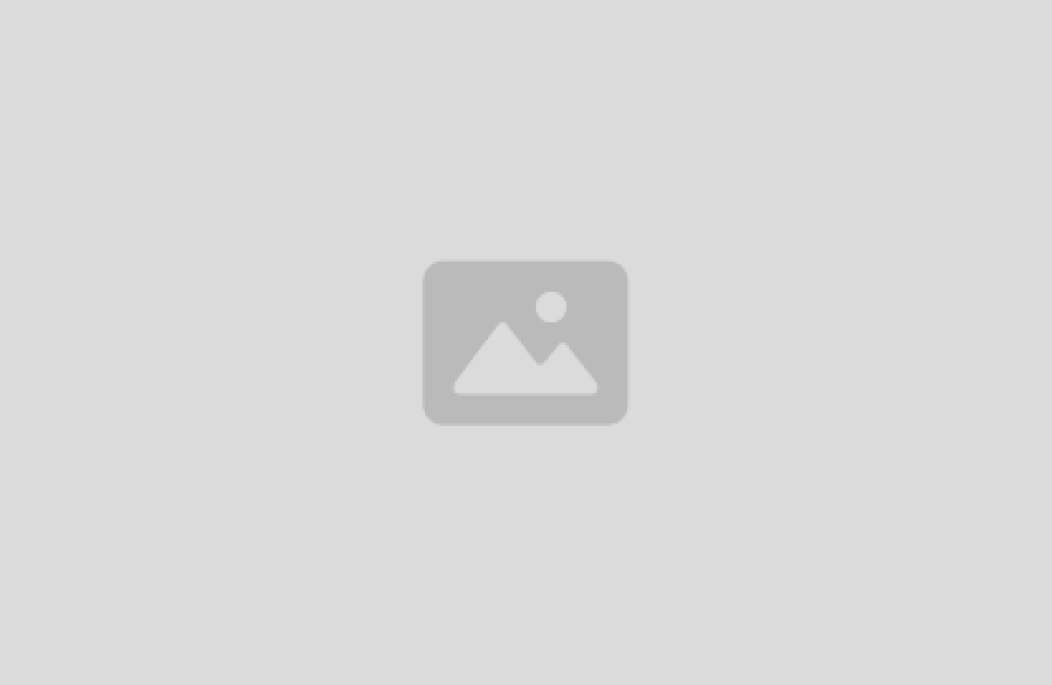
Discover more integrations
No items found.
Get in touch CTA Section
Lorem ipsum dolor sit amet, consectetur adipiscing elit, sed do eiusmod tempor incididunt ut labore et dolore magna aliqua.
Frequently asked questions
How did Sifflet help reduce onboarding time for new data team members at jobvalley?
Sifflet’s data catalog provided a clear and organized view of jobvalley’s data assets, making it much easier for new team members to understand the data landscape. This significantly cut down onboarding time and helped new hires become productive faster.
How does integrating data observability improve SLA compliance?
Integrating data observability helps you stay on top of data issues before they impact your users. With real-time metrics, pipeline error alerting, and dynamic thresholding, you can catch problems early and ensure your data meets SLA requirements. This proactive monitoring helps teams maintain trust and deliver consistent, high-quality data services.
How can I ensure SLA compliance during data integration?
To meet SLA compliance, it's crucial to monitor ingestion latency, data freshness checks, and throughput metrics. Implementing data observability dashboards can help you track these in real time and act quickly when something goes off track. Sifflet’s observability platform helps teams stay ahead of issues and meet their data SLAs confidently.
What benefits does end-to-end data lineage offer my team?
End-to-end data lineage helps your team perform accurate impact assessments and faster root cause analysis. By connecting declared and built-in assets, you get full visibility into upstream and downstream dependencies, which is key for data reliability and operational intelligence.
What role does data pipeline monitoring play in Dailymotion’s delivery optimization?
By rebuilding their pipelines with strong data pipeline monitoring, Dailymotion reduced storage costs, improved performance, and ensured consistent access to delivery data. This helped eliminate data sprawl and created a single source of truth for operational teams.
What makes a data observability platform truly end-to-end?
Great question! A true data observability platform doesn’t stop at just detecting issues. It guides you through the full lifecycle: monitoring, alerting, triaging, investigating, and resolving. That means it should handle everything from data quality monitoring and anomaly detection to root cause analysis and impact-aware alerting. The best platforms even help prevent issues before they happen by integrating with your data pipeline monitoring tools and surfacing business context alongside technical metrics.
Why is field-level lineage important in data observability?
Field-level lineage gives you a detailed view into how individual data fields move and transform through your pipelines. This level of granularity is super helpful for root cause analysis and understanding the impact of changes. A platform with strong data lineage tracking helps teams troubleshoot faster and maintain high data quality.
What’s new in Sifflet’s integration with dbt?
We’ve supercharged our dbt integration! Sifflet now offers deeper metadata visibility and powerful dbt impact analysis for both GitHub and GitLab. This helps you assess the downstream effects of model changes before deployment, boosting your confidence and control in data pipeline monitoring.