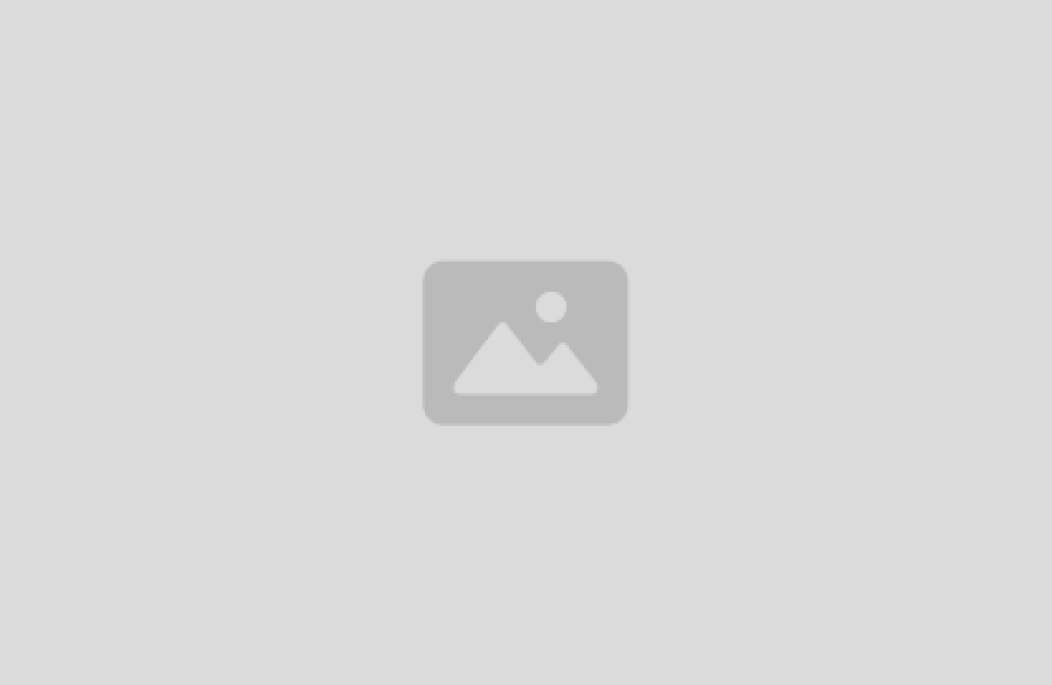
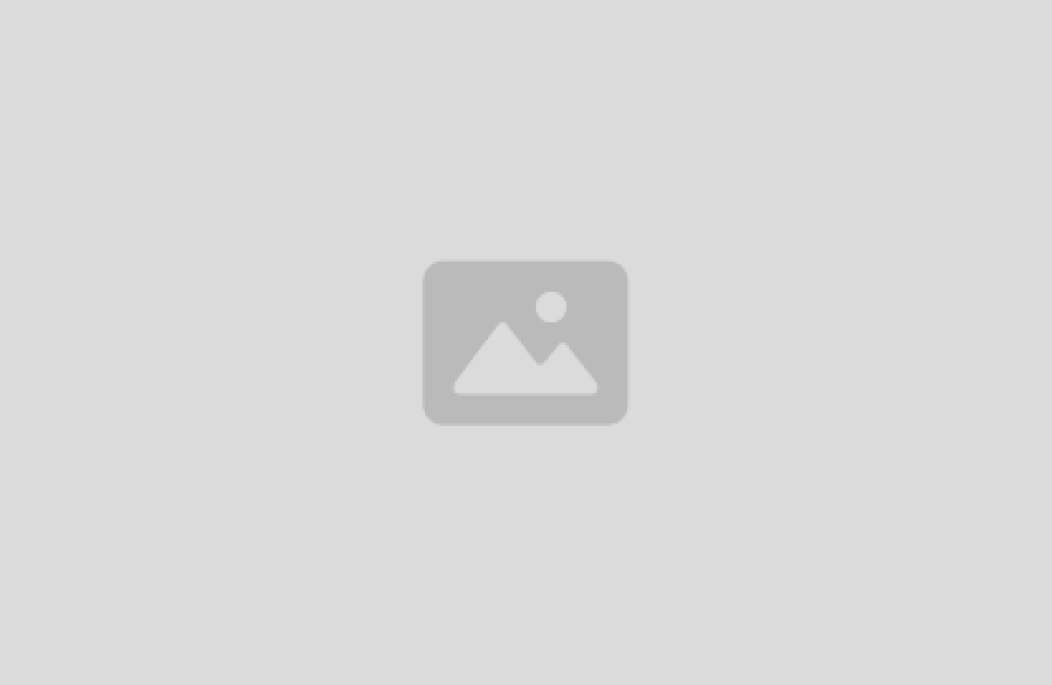
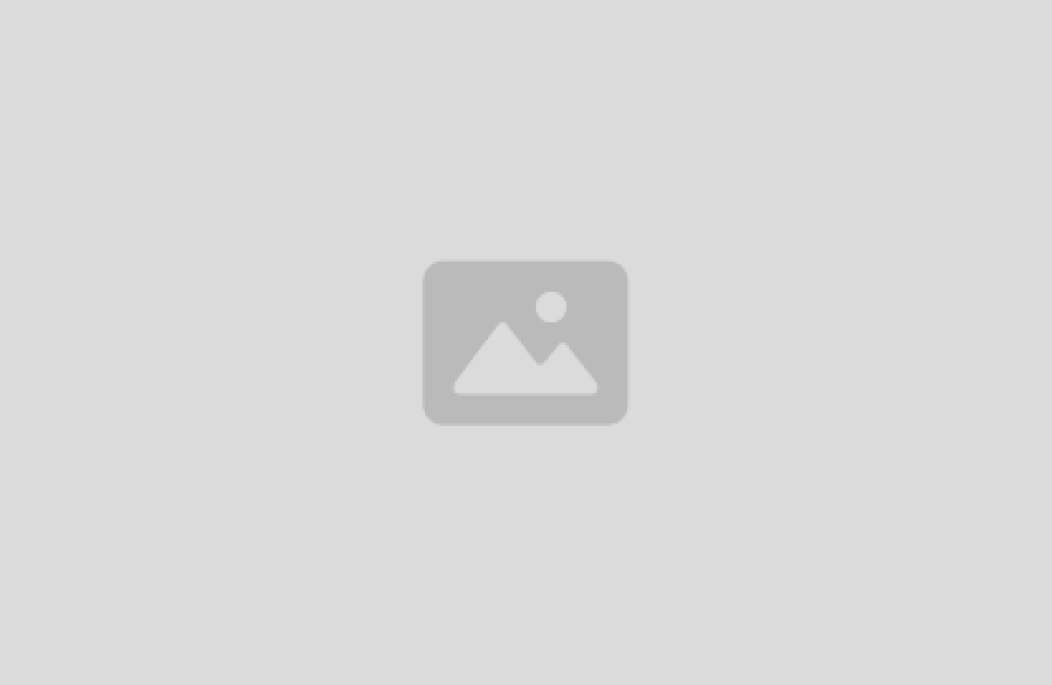
Discover more integrations
No items found.
Get in touch CTA Section
Lorem ipsum dolor sit amet, consectetur adipiscing elit, sed do eiusmod tempor incididunt ut labore et dolore magna aliqua.
Frequently asked questions
Will there be live demonstrations of Sifflet’s observability platform?
Absolutely! Our team will be offering hands-on demos that showcase how our observability tools integrate into your workflows. From real-time metrics to data quality monitoring, you’ll get a full picture of how Sifflet boosts data reliability across your stack.
Is Sifflet Insights easy to set up with my existing tools?
Yes, onboarding is seamless. You can quickly integrate Sifflet Insights with your existing BI tools and start receiving real-time metrics and alerts. It’s designed to enhance efficiency and support incident response automation without disrupting your current workflows.
Why does AI often fail even when the models are technically sound?
Great question! AI doesn't usually fail because of bad models, but because of unreliable data. Without strong data observability in place, it's hard to detect data issues like schema changes, stale tables, or broken pipelines. These problems undermine trust, and without trust in your data, even the best models can't deliver value.
Why is standardization important when scaling dbt, and how does Sifflet support it?
Standardization is key to maintaining control as your dbt project grows. Sifflet supports this by centralizing metadata and enabling compliance monitoring through features like data contracts enforcement and asset tagging. This ensures consistency, improves data governance, and reduces the risk of data drift or unmonitored critical assets.
How does Sifflet help reduce alert fatigue in data observability?
Sifflet uses AI-driven context and dynamic thresholding to prioritize alerts based on impact and relevance. Its intelligent alerting system ensures users only get notified when it truly matters, helping reduce alert fatigue and enabling faster, more focused incident response.
How did Sifflet help reduce onboarding time for new data team members at jobvalley?
Sifflet’s data catalog provided a clear and organized view of jobvalley’s data assets, making it much easier for new team members to understand the data landscape. This significantly cut down onboarding time and helped new hires become productive faster.
Can better design really improve data reliability and efficiency?
Absolutely. A well-designed observability platform not only looks good but also enhances user efficiency and reduces errors. By streamlining workflows for tasks like root cause analysis and data drift detection, Sifflet helps teams maintain high data reliability while saving time and reducing cognitive load.
When should organizations start thinking about data quality and observability?
The earlier, the better. Building good habits like CI/CD, code reviews, and clear documentation from the start helps prevent data issues down the line. Implementing telemetry instrumentation and automated data validation rules early on can significantly improve data pipeline monitoring and support long-term SLA compliance.